Boosted Decision Tree (BDT) model for political analysis: Using machine learning to assess the Anonymous campaign against Islamic Extremists on Twitter
semanticscholar
Abstract
Social scientists now have unprecedented access to a wealth of information on human behavior, but "big data" pose unique analytical challenges. Classic regression models, which make rigid assumptions regarding the data generating process, are often unsuited for extracting information from big data. Machine learning (ML) models, in contrast, are ideal for this task because they are flexible. In this article, we review major families of ML models, with a focus on Boosted Decision Tree (BDT) models, which is the gold standard of ML models. Furthermore, we explain how to compute the "marginal effect" of any variable and uncertainty estimates for ML models. We illustrate our techniques with an analysis of an original dataset of 16,286 suspicious jihadist Twitter accounts reported by cyber activists. This article provides a guide on ML methods for political scientists, and contributes to our understanding of the politics of social media. ∗Corresponding author. ABD, Department of Political Science, and Master’s Candidate in Statistics, Department of Mathematics, Washington University at St. Louis. elena.labzina@wustl.edu. †Dickey Fellow in U.S.Foreign Policy and International Security, Dartmouth College. george.yin1@gmail.com.
MoreTranslated text
AI Read Science
Must-Reading Tree
Example
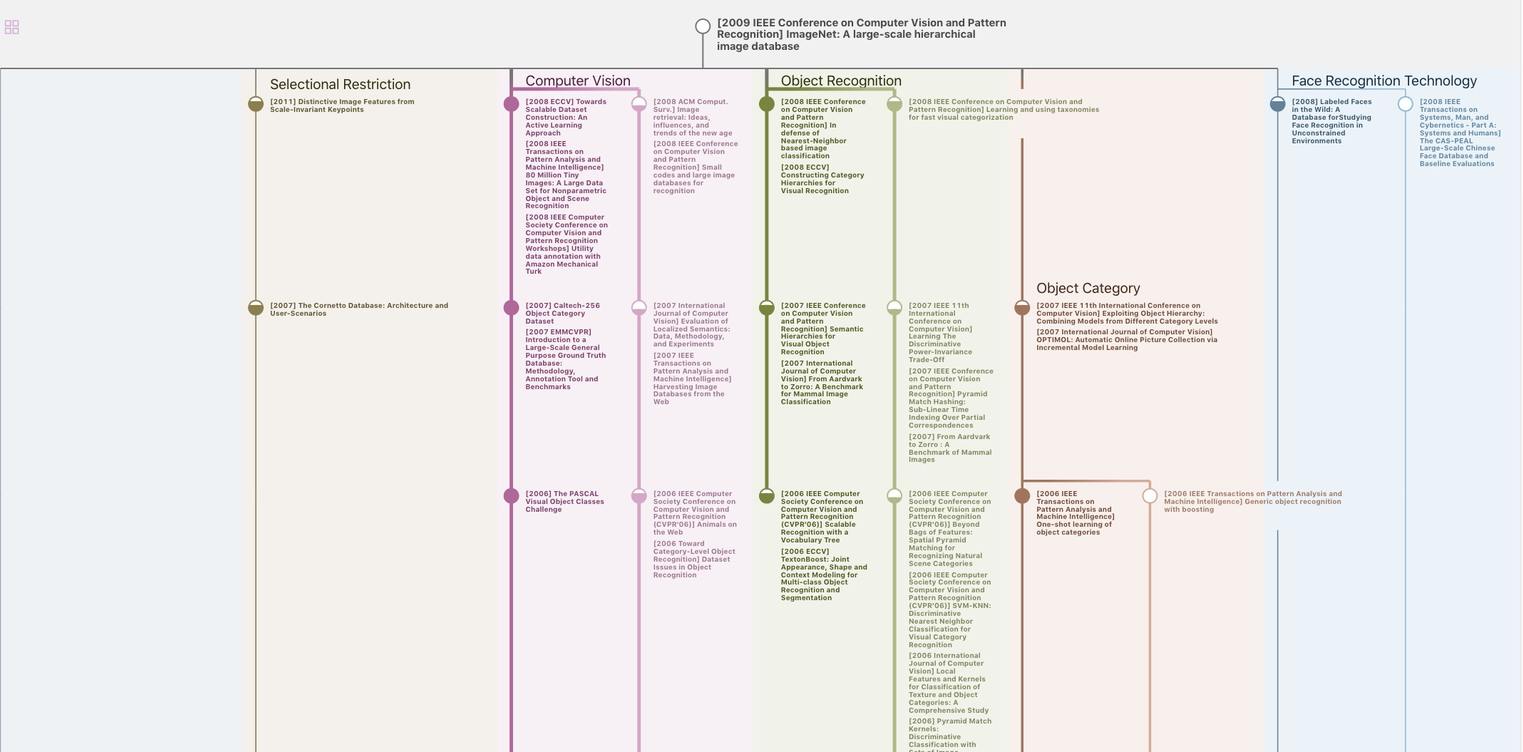
Generate MRT to find the research sequence of this paper
Chat Paper
Summary is being generated by the instructions you defined