Sketching Sparse Covariance Matrices and Graphs
semanticscholar(2013)
摘要
This paper considers the problem of recovering a sparse p×p matrix X given an m×m matrix Y = AXB , where A and B are known m× p matrices with m p. The main result shows that there exist constructions of the “sketching” matrices A and B so that even if X has O(p) non-zeros, it can be recovered exactly and efficiently using convex optimization as long as these non-zeros are not concentrated in any single row/column of X. Furthermore, it suffices for the size of Y (the sketch dimension) to scale as m = O (√ # nonzeros in X × log p ) . Our approach relies on a novel result concerning tensor products of random bipartite graphs, which may be of independent interest. We also describe two interesting applications of our results: (a) estimating sparse covariance matrices from compressed realizations and (b) a novel paradigm of lossless sketching/compression of sparse graphs.
更多查看译文
AI 理解论文
溯源树
样例
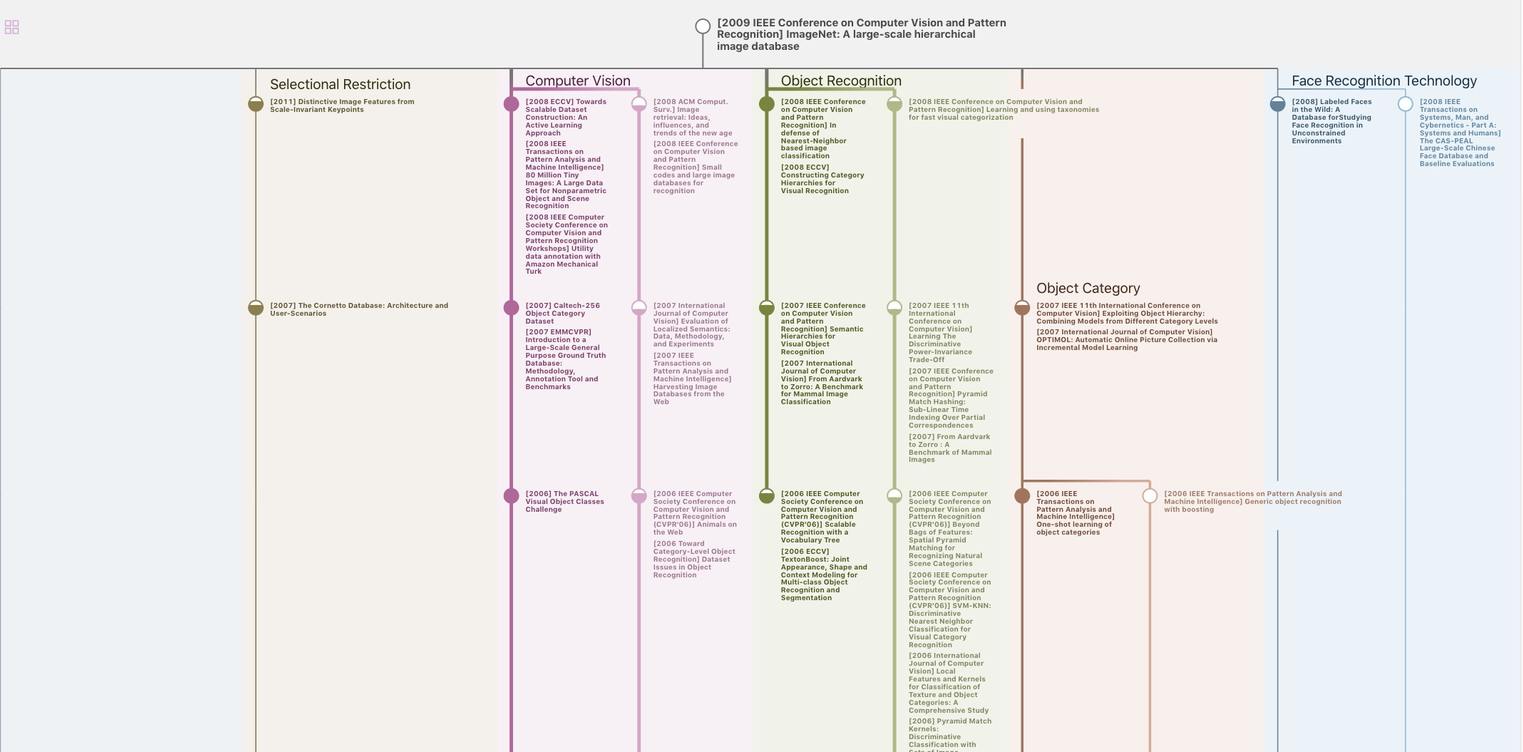
生成溯源树,研究论文发展脉络
Chat Paper
正在生成论文摘要