Fractal Dimension Analysis of EEG Time Series Signal Buried in Random Noise
semanticscholar(2016)
Abstract
The presence of correlated randomness in EEG time series signal buried in random noise was analyzed with the Higuchi fractal algorithm. The signal recognition algorithm design was built on the fact that the theoretical fractal dimension value of 2 is an indicator for a white noise time series and correlated randomness would alter the fractal dimension value from 2. However, the limited number of data points in a real time series would give rise to a fractal dimension value different from 2 as well. A distribution of fractal dimension values can be simulated for short random series (N= 100). A criterion of white noise assignment to time series data (N = 100) with fractal dimension values more than 1.98 suggested that random noise level at 5-fold would not be detrimental in the studied signal recovery process. The analysis of the Walsh-Hadamard transform coefficient Shannon entropy was found to be informative and can serve as a supplemental criterion for the recognition of the presence of a signal. An iterative simulation algorithm was used to extract out the signal using the criterion that the remaining part must have a maximum fractal dimension around the theoretical value of 2. Applications to spike-timingdependent plasticity in neuron network study, signal speed extraction in exercise induced myelinogenesis study, and low budget mobile EEG devices for mobile Health are discussed Keywords—fractal dimension, Higuchi fractal algorithm, correlated randomness, Shannon entropy, Walsh-Hadamard Transform, spike-timing-dependent plasticit, exercise induced myelinogenesis study
MoreTranslated text
AI Read Science
Must-Reading Tree
Example
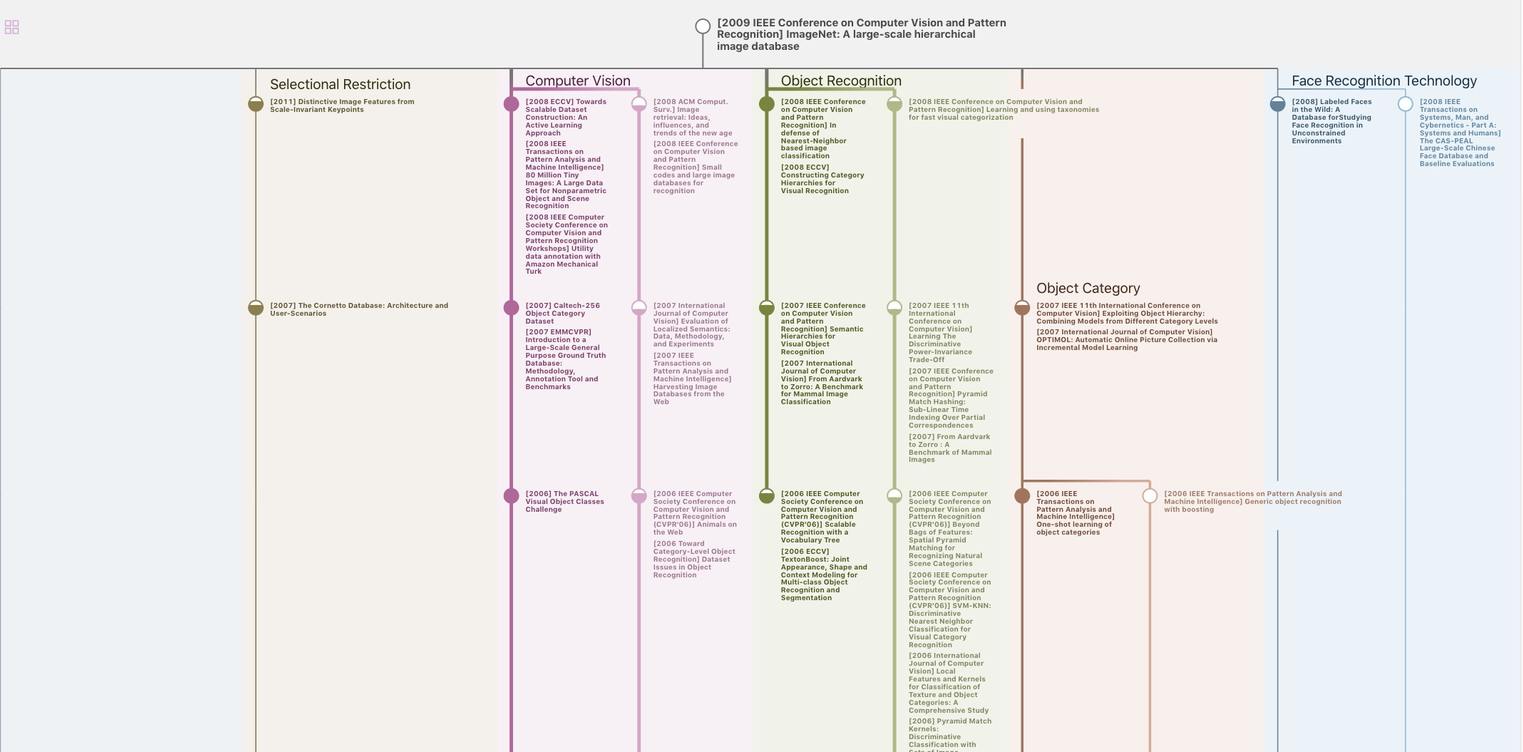
Generate MRT to find the research sequence of this paper
Chat Paper
Summary is being generated by the instructions you defined