Final Program Report : SAMSI Computational Advertising Program Summer
semanticscholar(2012)
摘要
E-commerce has flourished over the last few years, huge volumes of web data are generated and are to be analyzed. Recommender systems aim to match best products with the customers. Quality of recommendations plays a significant role in market place. Matrix factorization models are a type of latent factor models that has shown its strength in coping with the problem of matching consumers with the best products. However, recommendations become difficult when available data are massive and sparse. Such datasets include Yahoo! Music data. The goal of this project is to create a statistical procedure for matching Yahoo! Music users with artists based on their past rating behaviour. Performance of the statistical procedure is evaluated using numerical simulations.
更多查看译文
AI 理解论文
溯源树
样例
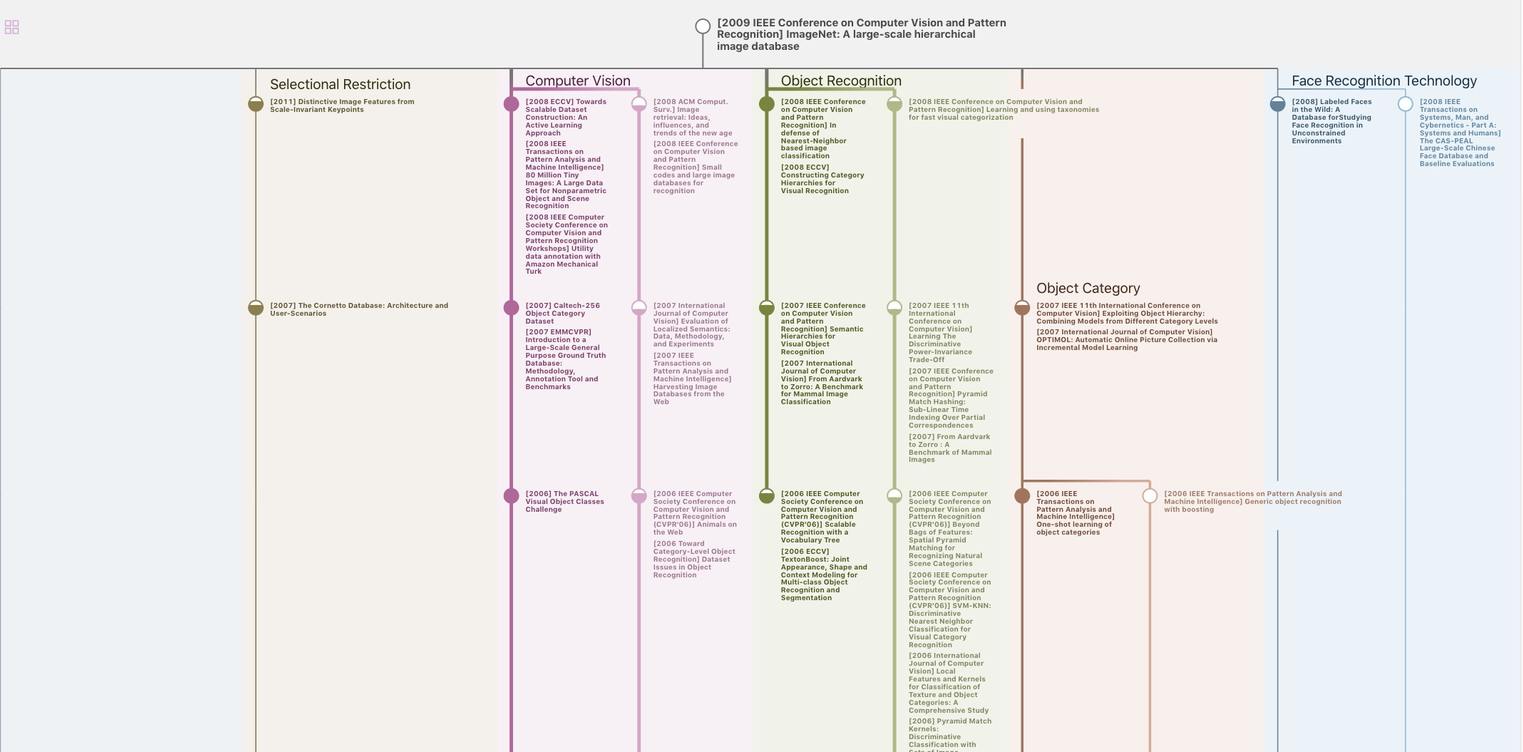
生成溯源树,研究论文发展脉络
Chat Paper
正在生成论文摘要