On the Dynamics of Adversarial Input Attacks
semanticscholar(2018)
摘要
An intriguing property of deep neural networks (DNNs) is their inherent vulnerability to adversarial inputs, which are maliciously crafted inputs to trigger DNNs to misbehave. The threats of adversarial inputs significantly hinder the application of DNNs in security-critical domains. Despite the plethora of work on adversarial input attacks and defenses, many important questions remain mysterious, including (i) How are adversarial inputs crafted to trigger targeted DNNs to misbehave? (ii) How are adversarial inputs generated by varied attack models different in their underlying mechanisms? (iii) How are complicated DNNs more vulnerable to adversarial inputs? (iv) How are existing defenses often ineffective against adaptive attacks? (v) How are transferable adversarial inputs different from non-transferable ones? This work represents a solid step towards answering the above key questions. Rather than focusing on the static properties of adversarial inputs from an input-centric perspective (i.e., whether the given input can deceive a targeted DNN), we conduct the first study on their dynamic properties from a DNNcentric perspective (i.e., how the targeted DNN reacts to a given adversarial input). Specifically, using a data-driven approach, we investigate the information flows of normal and adversarial inputs within varied DNN models and conduct in-depth comparative analysis of their discriminative patterns. Our study sheds light on the aforementioned questions, and points to several promising directions for designing more effective defense mechanisms.
更多查看译文
AI 理解论文
溯源树
样例
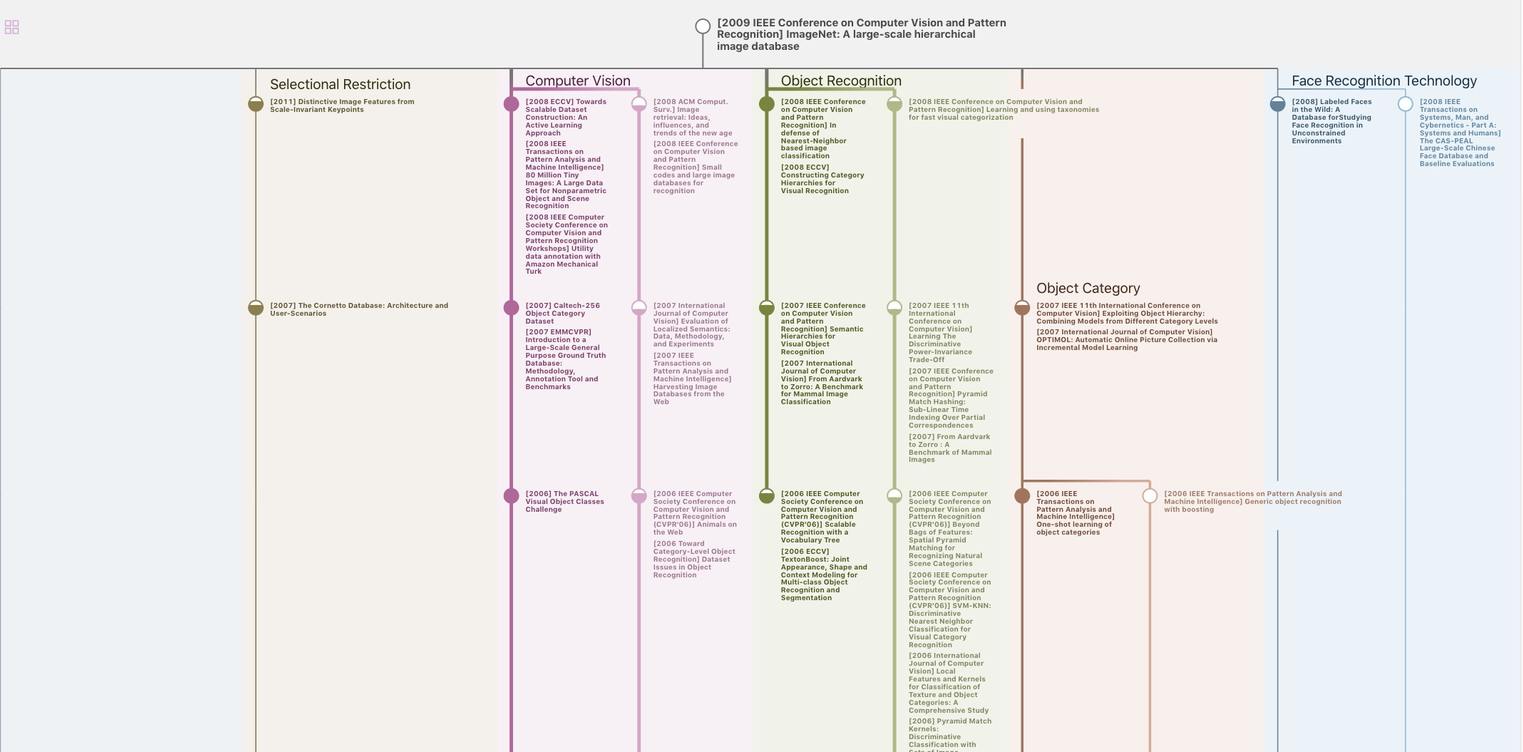
生成溯源树,研究论文发展脉络
Chat Paper
正在生成论文摘要