One-Versus Two-Step Approaches to Survey Nonresponse Adjustments
semanticscholar(2017)
摘要
In a series of papers, Little and Vartivarian (2003, 2005) argued that basing survey nonresponse adjustments on propensity to respond could increase the sampling variance of the estimates while not reducing bias, if the predictors of response were unrelated to key survey outcomes. Applying this idea to a 2014 military workforce survey, RAND researchers used machine learning approaches to develop a two-step method for nonresponse adjustment. The two step method comprises (1) a model for the key outcome variables based on respondents and (2) a response propensity model using the predicted key outcome variables as predictors for all sampled units. At the 2016 JSM, we presented simulation results assessing the predictive performance of competing machine learning algorithms in the first of the two steps. In this paper, we investigate the circumstances necessary for the two-step method to outperform nonresponse approaches in common practice, most of which can be regarded as single-step methods.
更多查看译文
AI 理解论文
溯源树
样例
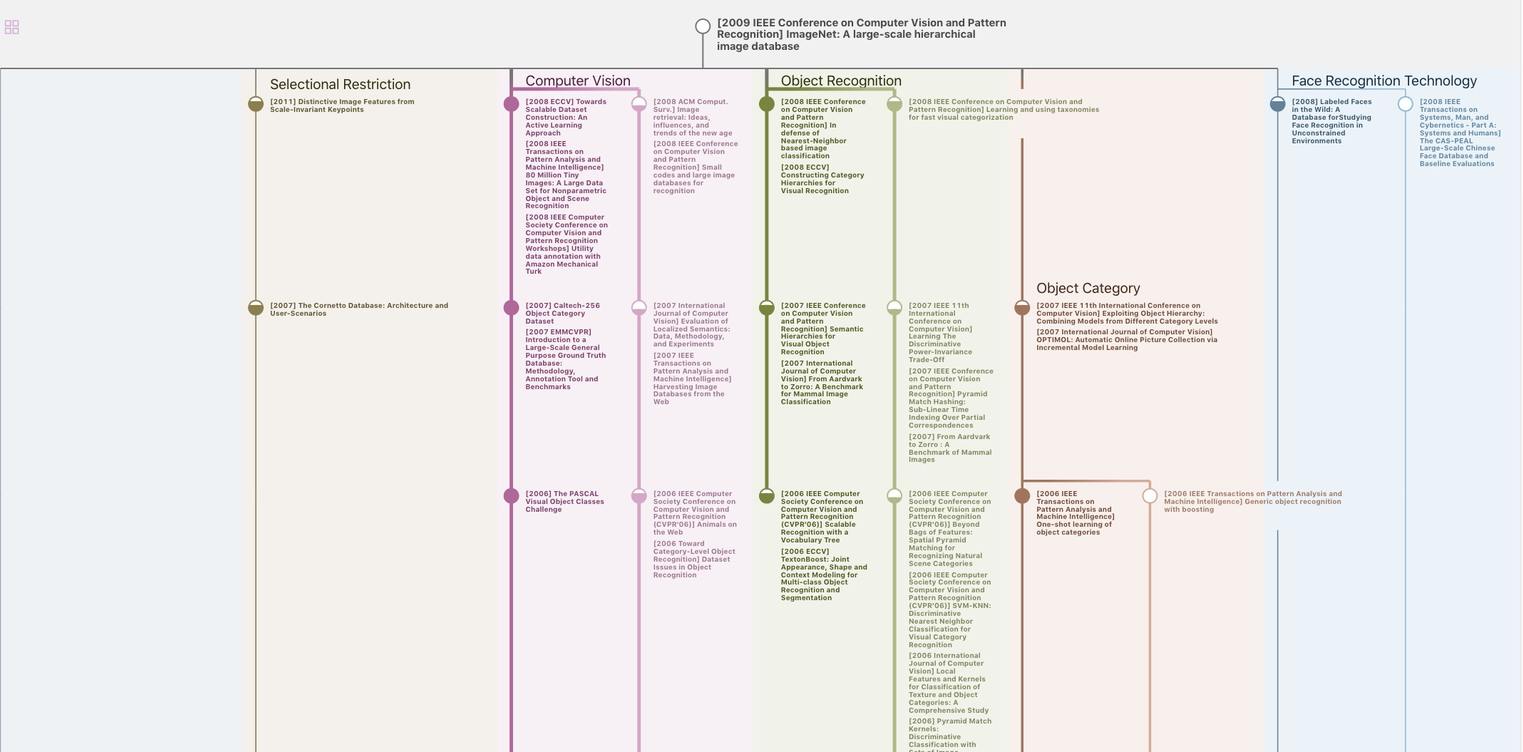
生成溯源树,研究论文发展脉络
Chat Paper
正在生成论文摘要