Clustering Multi-Attribute Uncertain Data using Probability Distribution
semanticscholar(2020)
摘要
Clustering is an unsupervised classification technique for grouping set of abstract objects into classes of similar objects. Clustering uncertain data is one of the essential tasks in mining uncertain data. Uncertain data is typically found in the area of sensor networks, weather data, customer rating data etc. The earlier methods for clustering uncertain data based on probability distribution, uses Kullback-Leibler divergence to measure similarity between two uncertain objects. In this paper, uncertain object in discrete domain is modeled, where uncertain object is treated as a discrete random variable. The Jenson-Shannon divergence is used to measure the similarity between two uncertain objects and integrate it into partitioning and density based clustering approaches. Experiments are performed to verify the effectiveness and efficiency of model developed and results are at par with the existing approaches.
更多查看译文
AI 理解论文
溯源树
样例
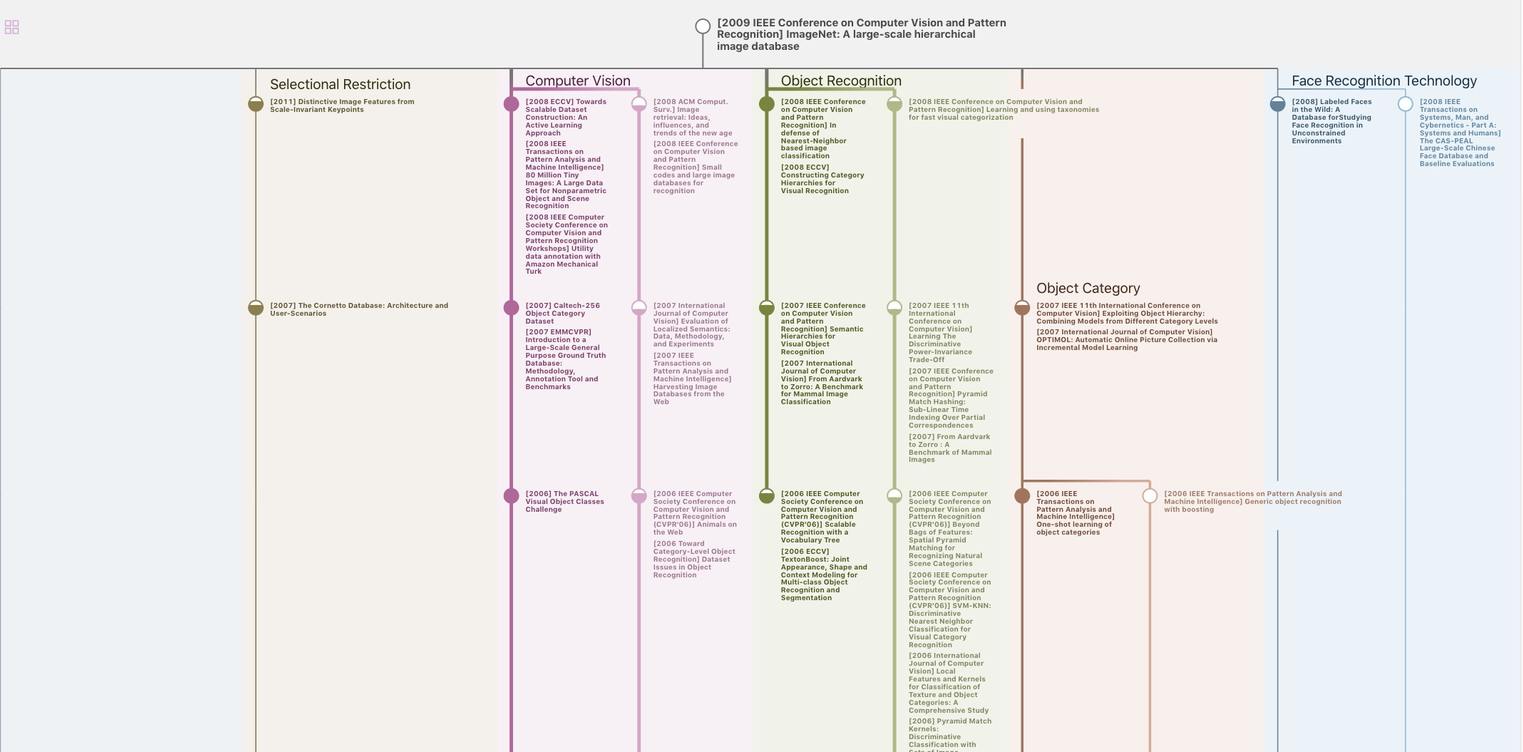
生成溯源树,研究论文发展脉络
Chat Paper
正在生成论文摘要