Passive Ship Localization in a Shallow Water Using Pre-trained Deep Learning Networks
semanticscholar(2019)
Abstract
Subject to the lack of detailed environmental information, the classical matched-field processing (MFP) may not be adapted to the accurate localization of underwater acoustic sources. In this paper, a framework that applies deep learning techniques instead of the MFP method is presented for the localization (direction-finding) of ship acoustic sources in a shallow water environment. The original data is recorded from a 128-element vertical array placed in a shallow water. The acquired array data is first processed by the time-domain conventional beamformer (CBF) in order to obtain the beamformed waveform signals corresponding to each direction-of-arrival (DOA) with a resolution of 1 degree. In the meantime, the GPS and recognized DOA information in the diagram of the target ships are employed to generate the labels for these beamformed signals. Base on the labeled data, a framework is proposed to predict the DOA information of target ships in a deep learning (DL) manner using the pre-trained state-of-the-art convolutional neural networks. Driven directly by the array signal data, the proposed method offers a way for the ship localization to overcome the environmental mismatch problem, which is believed to be better than that of conventional MFP method and some other shallow machine learning methods.
MoreTranslated text
AI Read Science
Must-Reading Tree
Example
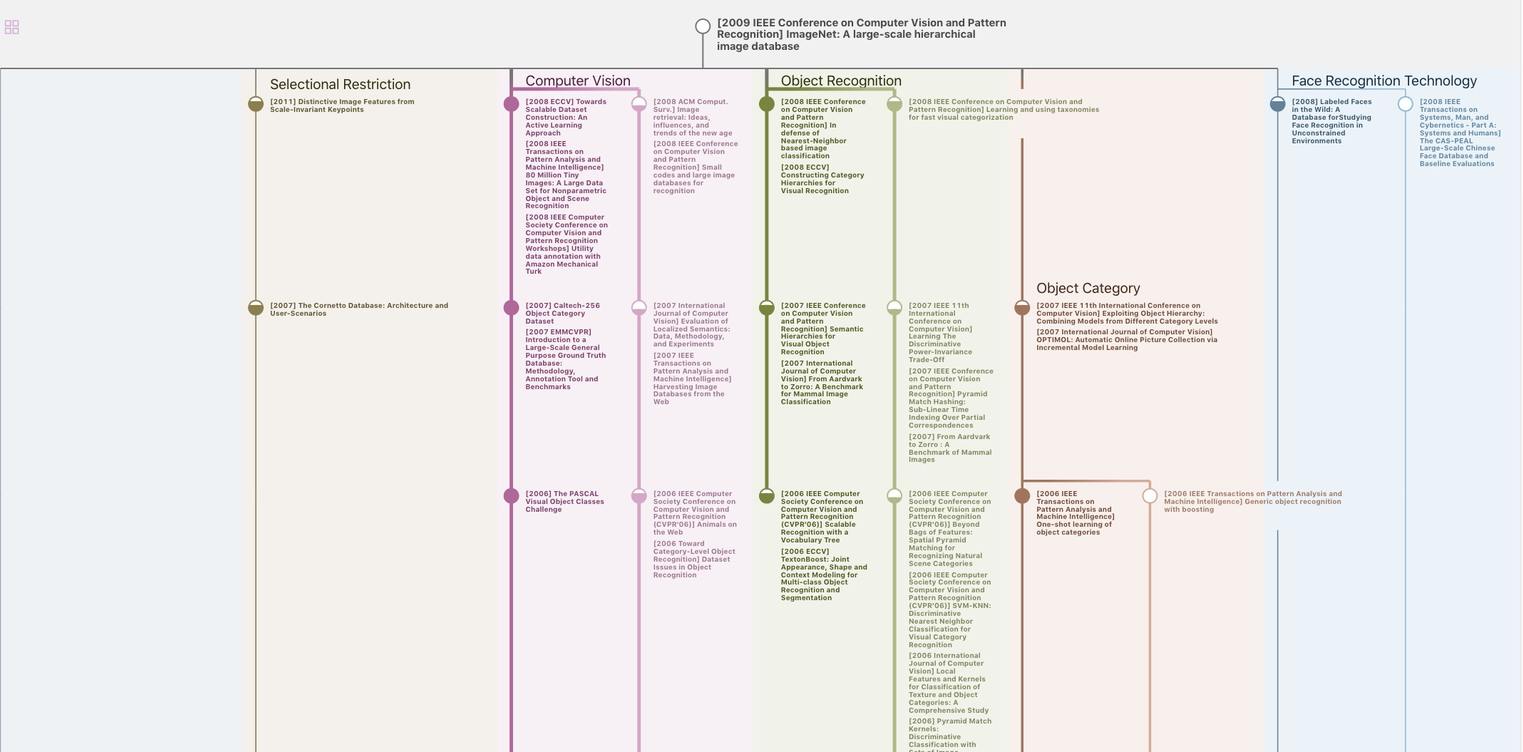
Generate MRT to find the research sequence of this paper
Chat Paper
Summary is being generated by the instructions you defined