Architecting Memory Systems for Emerging Technologies
semanticscholar(2018)
Abstract
The advance of traditional dynamic random access memory (DRAM) technology has slowed down, while the capacity and performance needs of memory system have continued to increase. This is a result of increasing data volume from emerging applications, such as machine learning and big data analytics. In addition to such demands, increasing energy consumption is becoming a major constraint on the capabilities of computer systems. As a result, emerging non-volatile memories, for example, Spin Torque Transfer Magnetic RAM (STT-MRAM), and new memory interfaces, for example, High Bandwidth Memory (HBM), have been developed as an alternative. Thus far, most previous studies have retained a DRAM-like memory architecture and management policy. This preserves compatibility but hides the true benefits of those new memory technologies. In this research, we proposed the co-design of memory architectures and their management policies for emerging technologies. First, we introduced a new memory architecture for an STT-MRAM main memory. In particular, we defined a new page mode operation for efficient activation and sensing. By fully exploiting the non-destructive nature of STTMRAM, our design achieved higher performance, lower energy consumption, and a smaller area than the traditional designs. Second, we developed a cost-effective technique to improve load balancing for HBM memory channels. We showed that the proposed technique was capable of efficiently redistributing memory requests across multiple memory channels to improve the channel utilization, resulting in improved performance.
MoreTranslated text
AI Read Science
Must-Reading Tree
Example
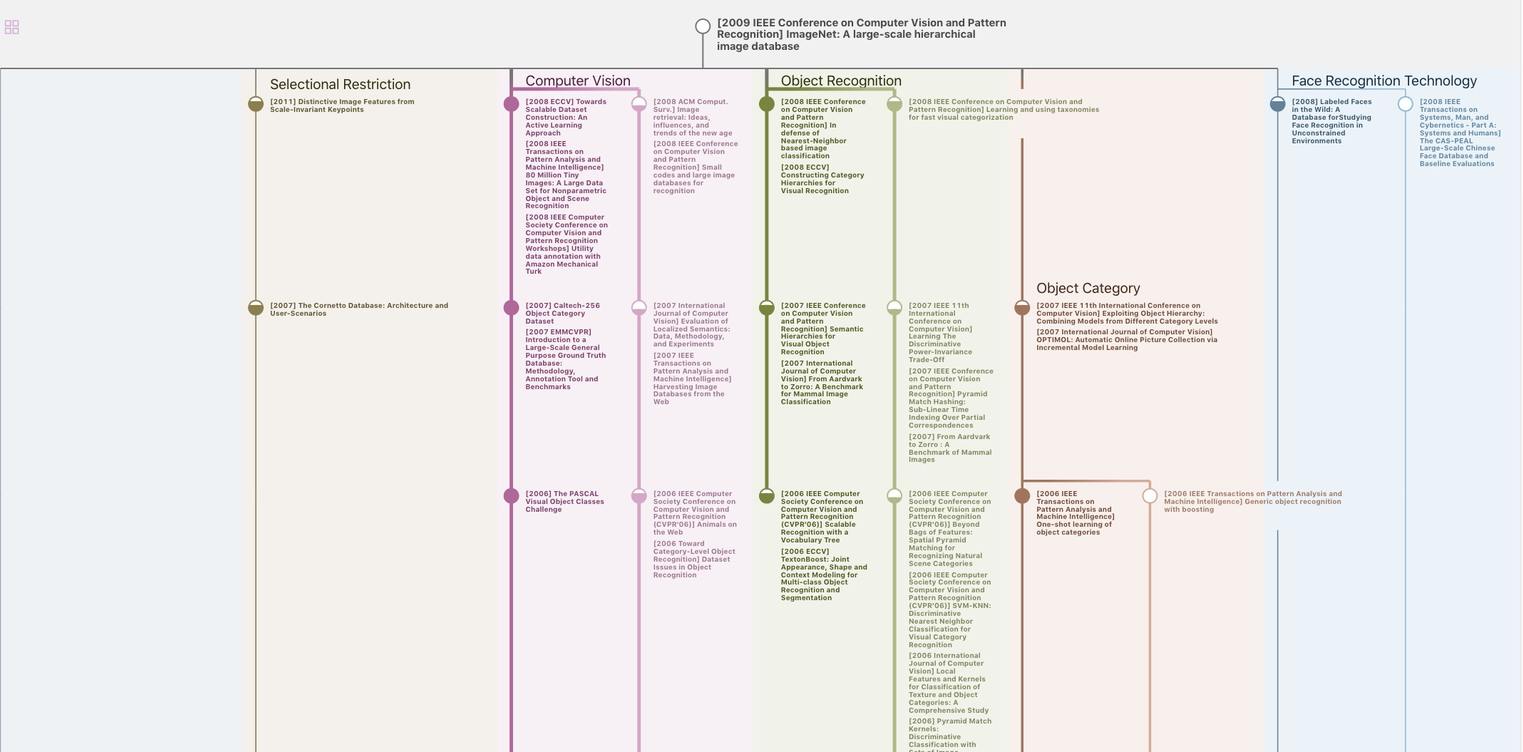
Generate MRT to find the research sequence of this paper
Chat Paper
Summary is being generated by the instructions you defined