Learning segmentations that balance latency versus quality in spoken language translation.
IWSLT(2015)
摘要
Segmentation of the incoming speech stream and translating segments incrementally is a commonly used technique that improves latency in spoken language translation. Previous work (Oda et al. 2014) [1] has explored creating training data for segmentation by finding segments that maximize translation quality with a user-defined bound on segment length. In this work, we provide a new algorithm, using Pareto-optimality, for finding good segment boundaries that can balance the trade-off between latency versus translation quality. We compare against the state-of-the-art greedy algorithm from (Oda et al. 2014) [1]. Our experimental results show that we can improve latency by up to 12% without harming the BLEU score for the same average segment length. Another benefit is that for any segment size, Paretooptimal segments maximize latency and translation quality.
更多查看译文
AI 理解论文
溯源树
样例
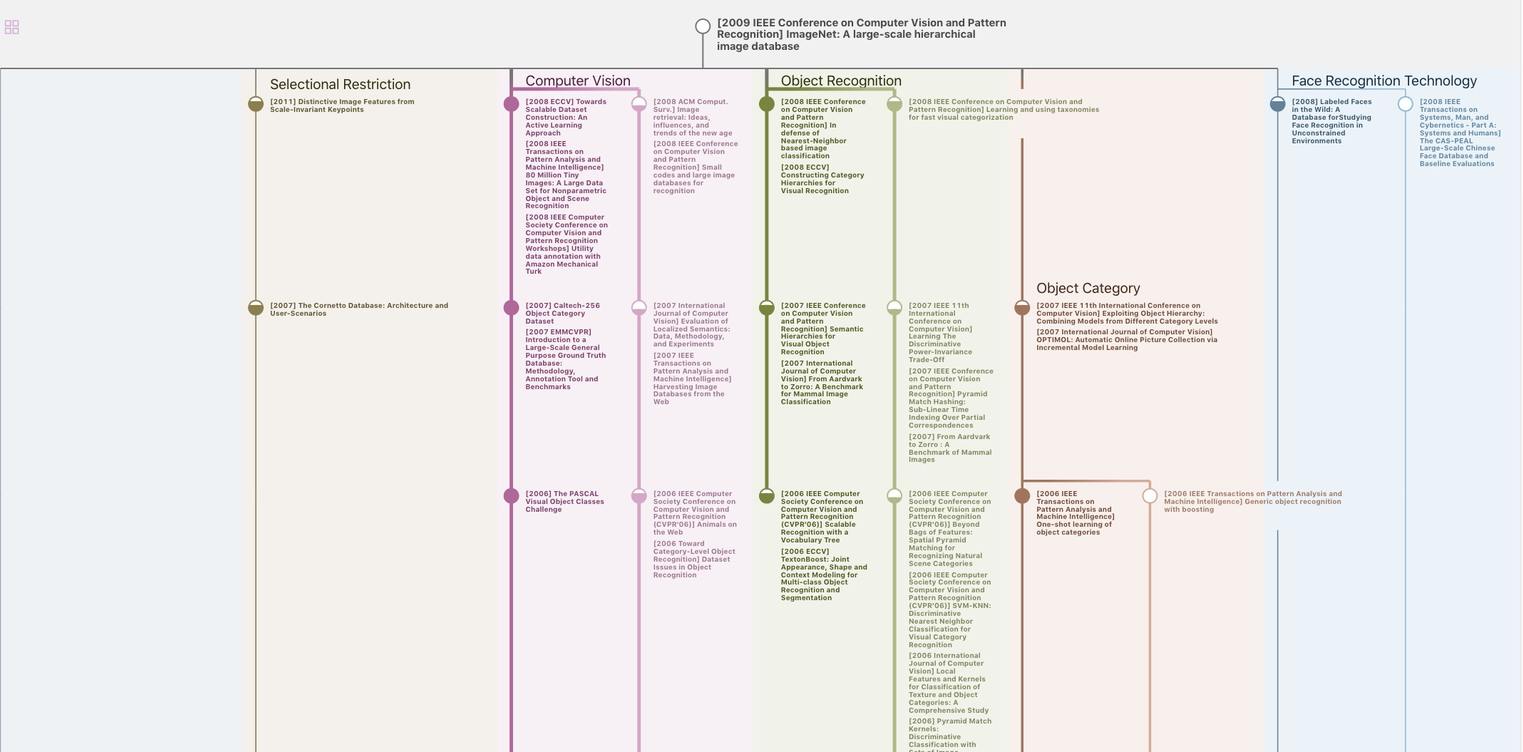
生成溯源树,研究论文发展脉络
Chat Paper
正在生成论文摘要