Deep Learning for Multi-Scale Molecular Modeling
semanticscholar(2020)
Abstract
Molecular simulations are widely applied in the study of chemical and bio-physical systems. However, theaccessible timescales of atomistic simulations are limited, and extracting equilibrium properties of systemscontaining rare events remains challenging. Two distinct strategies are usually adopted in this regard: eithersticking to the atomistic level and performing enhanced sampling, or trading details for speed by leveragingcoarse-grained models. Although both strategies are promising, either of them, if adopted individually,exhibits severe limitations. In this paper we propose a machine-learning approach to ally both strategies sothat simulations on different scales can benefit mutually from their cross-talks: Accurate coarse-grained (CG)models can be inferred from the fine-grained (FG) simulations through deep generative learning; In turn, FGsimulations can be boosted by the guidance of CG models via deep reinforcement learning. Our methoddefines a variational and adaptive training objective which allows end-to-end training of parametricmolecular models using deep neural networks. Through multiple experiments, we show that our method isefficient and flexible, and performs well on challenging chemical and bio-molecular systems.
MoreTranslated text
AI Read Science
Must-Reading Tree
Example
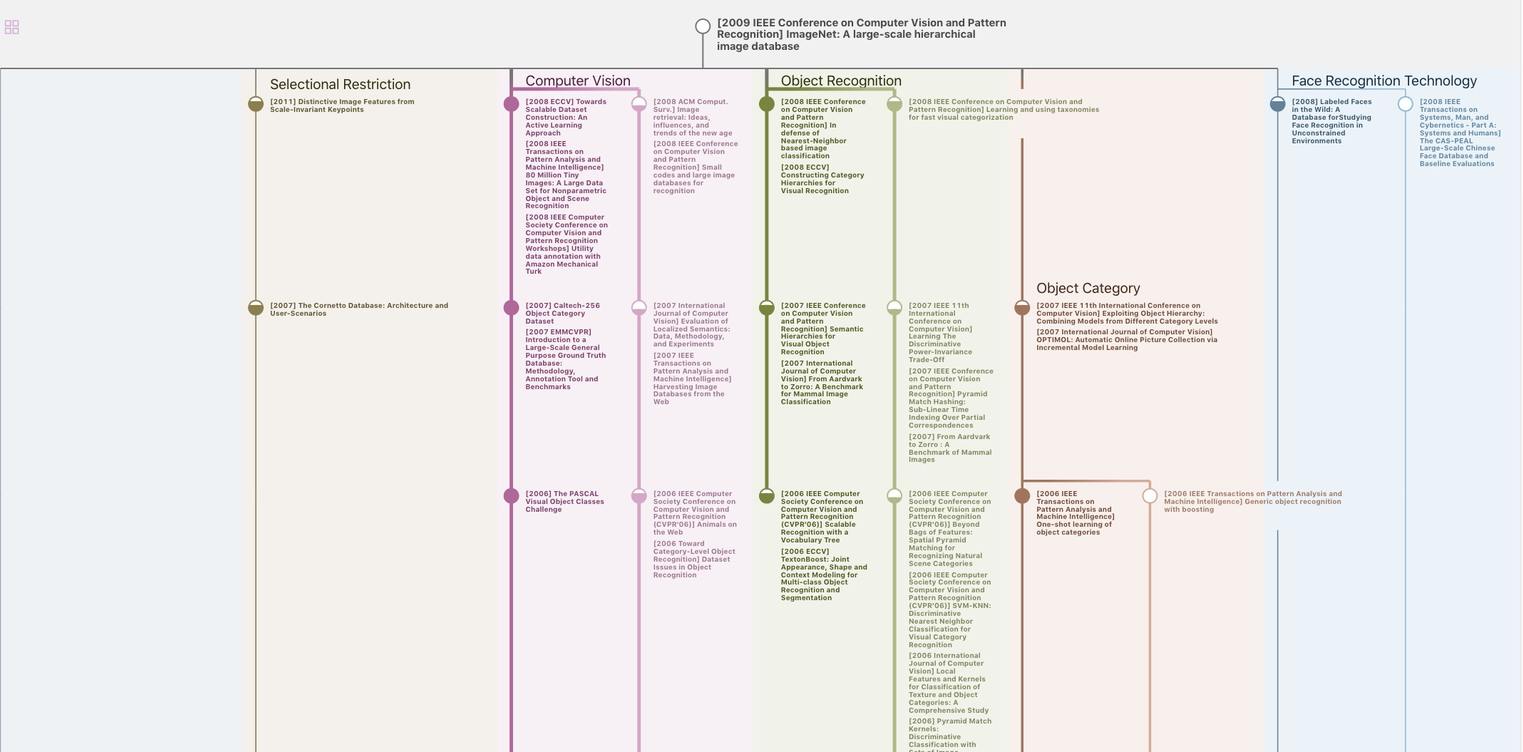
Generate MRT to find the research sequence of this paper
Chat Paper
Summary is being generated by the instructions you defined