Training Restricted Boltzmann Machines Using a Quantum Annealer
semanticscholar(2016)
摘要
Machine learning and the optimization involved therein is of critical importance for commercial and military applications. Due to the extremely complex nature of many-variable optimization, the conventional approach is to employ a meta-heuristic technique to find suboptimal solutions. Quantum Annealing (QA) hardware offers a completely novel approach for obtaining significantly better or optimal solutions with considerably large speedups when compared to traditional computing hardware. In this presentation, we describe our development of new machine learning algorithms tailored for QA hardware. We train a Restricted Boltzmann Machine (RBM) using QA hardware as a sampler. We present our initial results obtained by training RBMs on an image data set. We also discuss strategies for scaling up, including enhanced embedding and partitioned RBMs, to overcome the limitation imposed by current QA hardware. Keywords—machine learning, deep learning, quantum computing, Boltzmann distribution, sampling methods, quantum annealing
更多查看译文
AI 理解论文
溯源树
样例
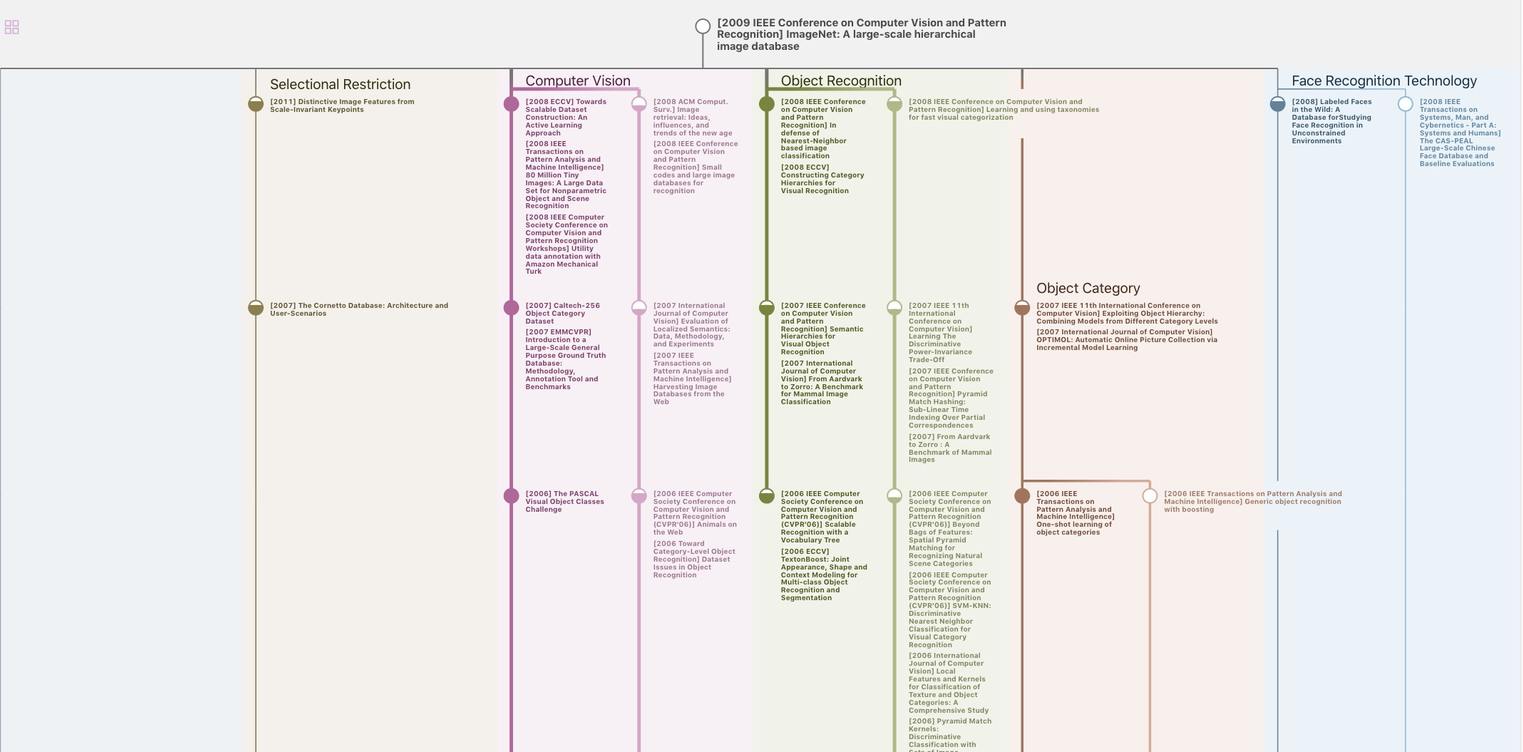
生成溯源树,研究论文发展脉络
Chat Paper
正在生成论文摘要