ERAD 2006 Proceedings of Improving the radar data mosaicking procedure by means of a quality descriptor
semanticscholar(2006)
Abstract
A considerable advantage offered by the presence of two (or more) radar exploring the same area, is the possibility to improve the quality of the final product, by adopting procedures of data composition. Radar data mosaicking is normally performed using simply range-related or value related algorithms of selection and combination. Usually, the followed ways are: a-b) to attribute to the common cell the mean or the maximum value of reflectivity; c) to assign to the common cell the reflectivity value of the nearest radar; d) to combine the data through a weighted average, where the weight is inversely proportional to the second power of the distance from the radar. The first approach distributes equally the probability to fail to the two radars, the second one handles only the problem of attenuation (which could be produced by mountains or rain), the third and the fourth are based on the assumption that radar data reliability diminishes only with the distance. Hence, these methods take into account only a very small part of the problems that spoil radar data and this could limit the advantages offered by the network. The quality of the datum is in fact conditioned by a wide spectrum of error sources, like clutter, bad calibration, beam blockage, measure done far from the ground (when the goal is to convert the reflectivity in precipitation rate), wind drift, anomalous propagation, hail, inhomogeneous beam filling. Moreover it depends on the completeness and efficacy of the correction and elaboration procedures used to obtain the final product. The aim of the present work is the definition, testing and verification of a new methodology of reflectivity radar data mosaicking based on a quality descriptor and the reconstruction of the correspondent rainfall field. This last is obtained propagating quality information from the raw data to the final product: to achieve this, in each step of the correction procedure it is introduced a quality component, finally they are combined in a unique value. This value takes into account the initial condition of the data with respect to the various sources of errors and the efficacy of the applied corrections. Two quality-based composition methods, consisting, the first, on the selection of the highest quality measure and the second on a qualityweighted average, are finally compared toward the methods of the maximum reflectivity and of the minimum distance, using raingauges measures as ‘true’ reference.
MoreTranslated text
AI Read Science
Must-Reading Tree
Example
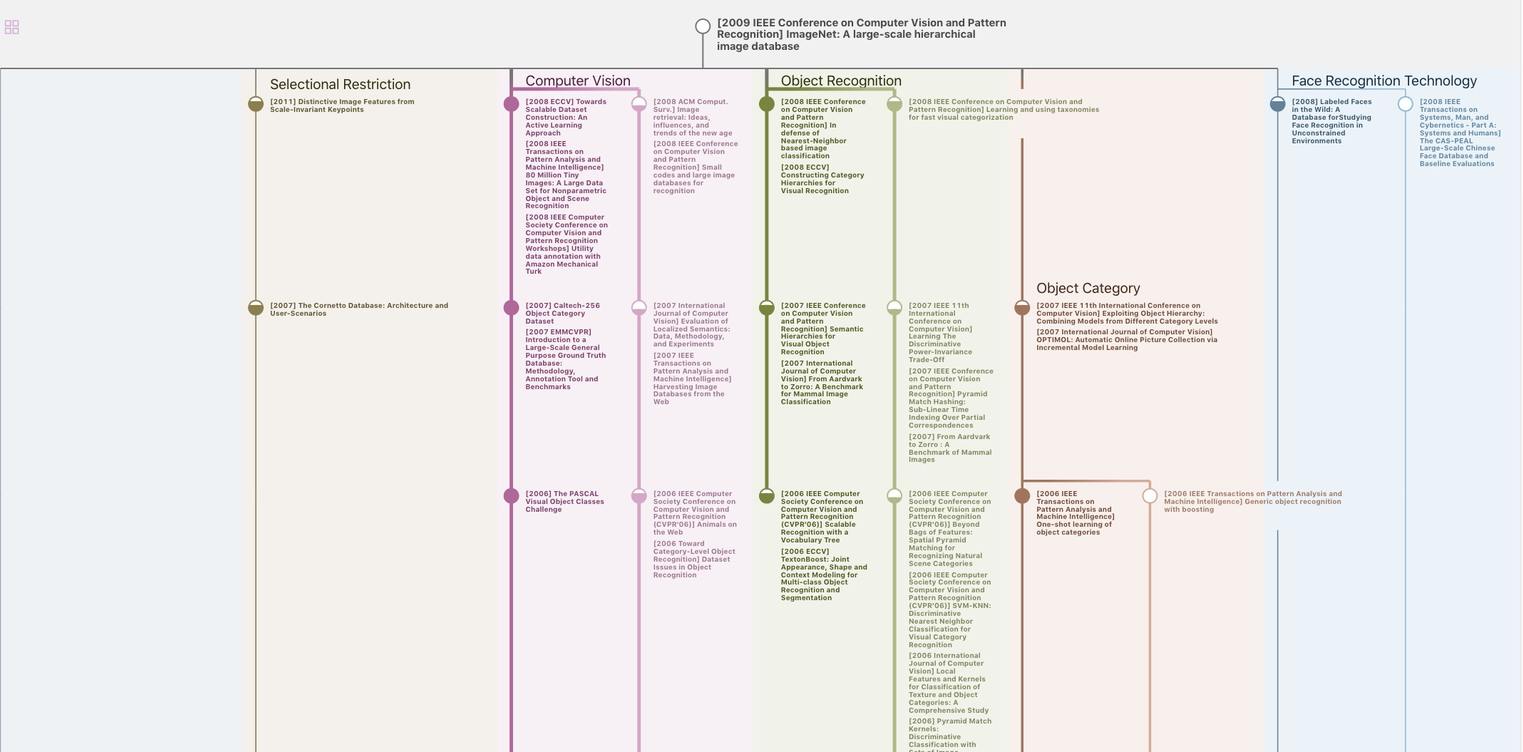
Generate MRT to find the research sequence of this paper
Chat Paper
Summary is being generated by the instructions you defined