Calibrating urban flood models with qualitative probabilistic flooding information extracted from CCTV footage
semanticscholar(2018)
摘要
It is of common agreement that urban flood modelling should be supported with monitoring data, be it for validating the simulation results or for calibrating the model parameters. Nevertheless, many practitioners develop and apply flood models on the basis of only limited monitoring data. This failure can largely be attributed to the lack of suitable sensing technology for urban floods. CCTV systems are an already existing source of flooding information that in recent years has been starting to be used for flood modelling. However, privacy concerns and the sheer volume of potentially available data call for automated data interpretation and assimilation techniques. In this work, we will demonstrate an automated methodology by which qualitative CCTV-sourced information can be used to calibrate a flood model, thus improving the model’s predictive performance. First, a deep learning algorithm based on convolutional neural networks is trained to detect flood water in CCTV images. Second, the qualitative evolution of flood depth is then estimated with an dimensionless index. Third, trend periods of rising and falling water are identified with quantified certainty. Finally, a flood model is calibrated with a novel objective function that takes into account the uncertainty of the trend information. The method will be demonstrated with experimental flash flood data, by which we hope to quantify the value of qualitative flooding information for modelling. If successful, the methods developed could pave the way to a new paradigm in urban flood model calibration.
更多查看译文
AI 理解论文
溯源树
样例
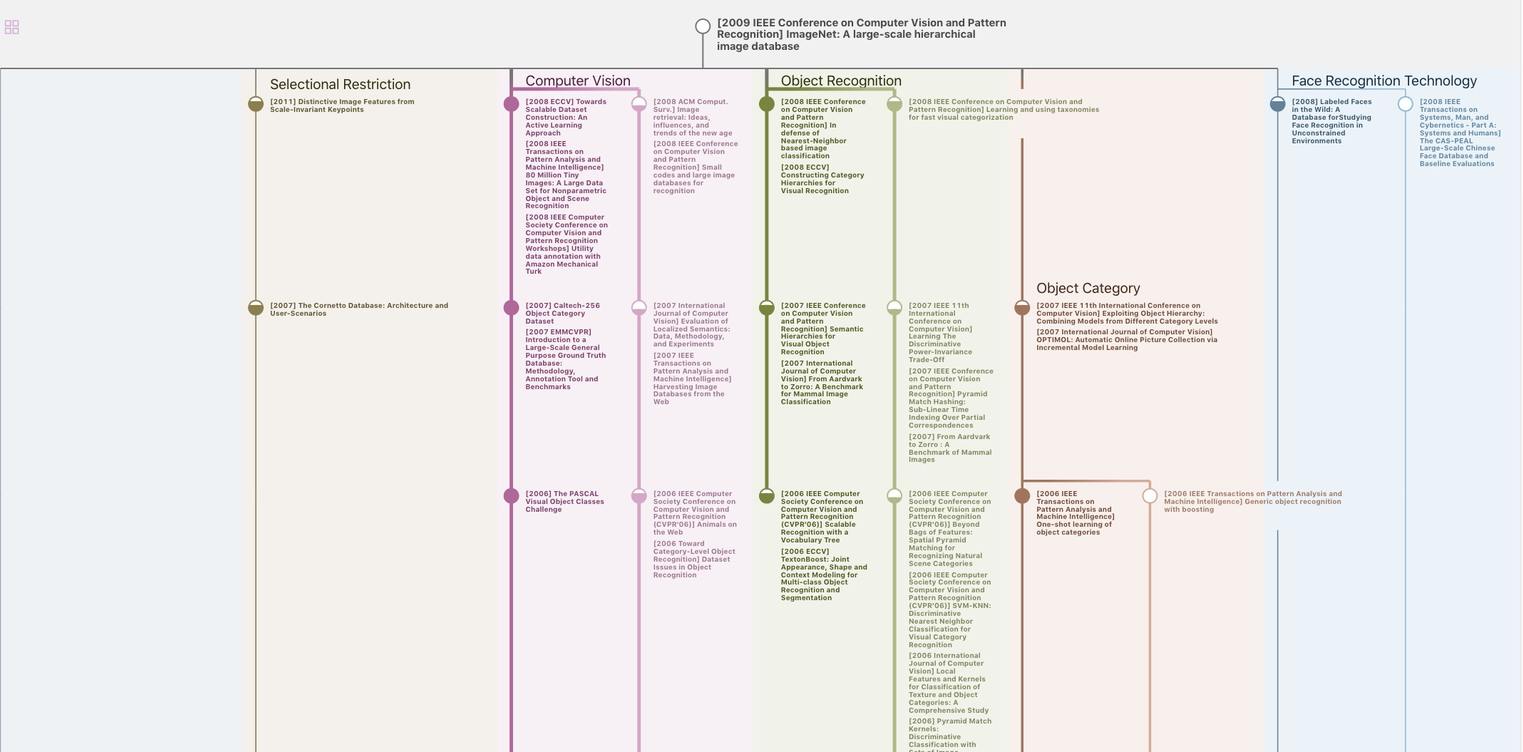
生成溯源树,研究论文发展脉络
Chat Paper
正在生成论文摘要