Learning Group Communication from Demonstration
semanticscholar(2018)
摘要
We consider the design of a communication policy for multi-group multi-agent communication, which takes as input the state of the world (e.g., history of communication, gaze direction, body pose of others) and outputs an optimal communication mode (e.g., speaking, listening, responding) for appropriate social interaction. A key component of our communication policy design is a communication gating module, termed the KinesicProxemic-Message Gate (KPM-Gate), that automatically infers group membership so that the actions generated by the communication policy depend only on the relevant group members. We pose the communication policy learning problem as a multiagent imitation learning problem and we learn a single shared policy across all agents under the assumption of a decentralized Markov decision process. We term our entire policy network as the Multi-Agent Group Discovery and Communication Mode Network (MAGDAM network) as it learns social group structure as well as the dynamics of group communication. Our experimental validation on both synthetic and real world data shows that our model is able to discover social group structure in addition to learning an accurate multi-agent communication policy.
更多查看译文
AI 理解论文
溯源树
样例
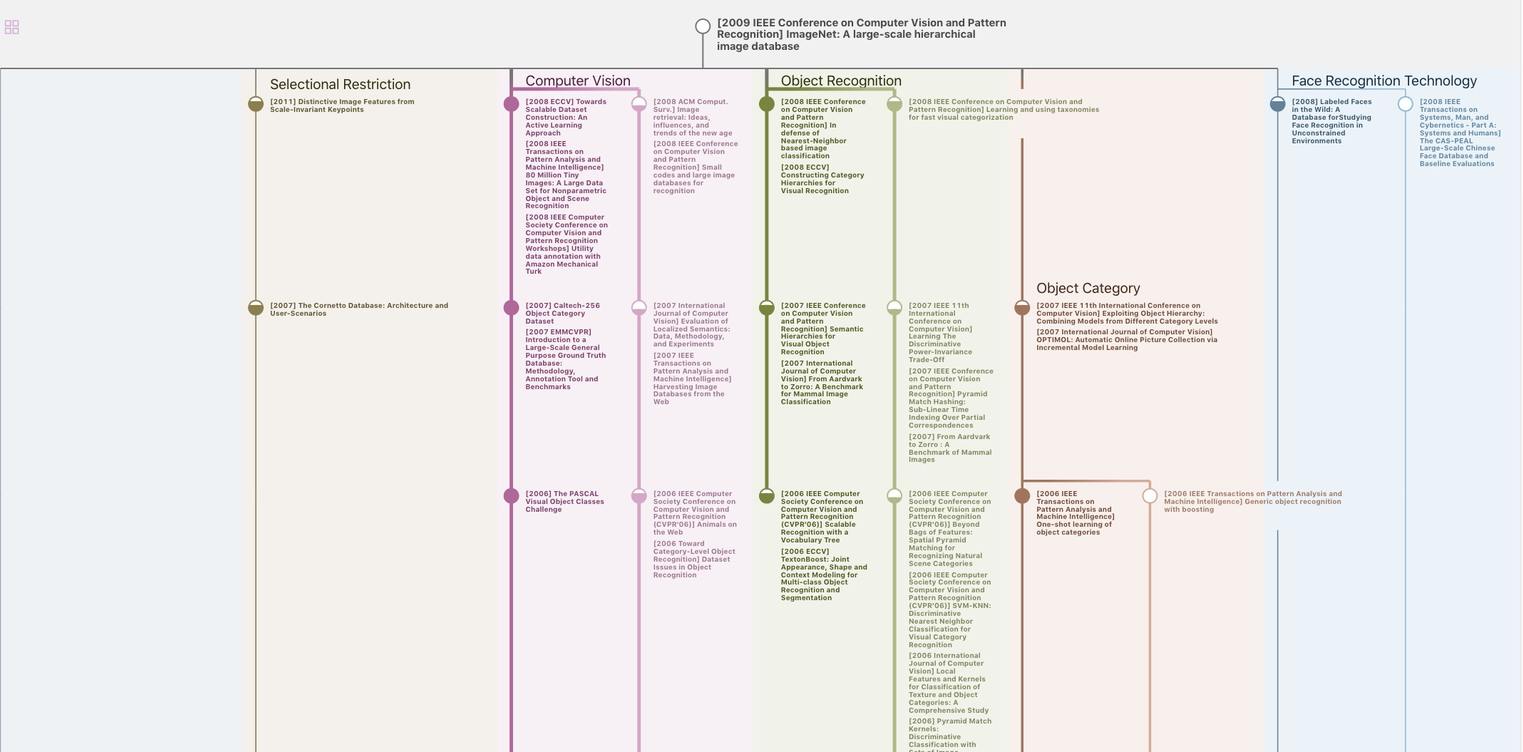
生成溯源树,研究论文发展脉络
Chat Paper
正在生成论文摘要