Improved model bias correction for precipitation extremes considering non-stationarity
semanticscholar(2017)
Abstract
The process of climate impact evaluation on water resources is inherent with various sources of uncertainties. In their earnest efforts scientists are attempting to narrow down these uncertainties for a robust future scenario assessment. The absence of recorded data for future hampers the computation of climate model bias in the corresponding period which compels to embrace the assumption that the bias in the scenario (future) period is similar to that in the historical period. Hence the stationarity of bias is adopted by default adding to the uncertainty and reducing the predictive ability of the hydrological model. It is well known that the behavior of hydrological process changes and their probabilistic parameters may no longer be constant due to non-stationary conditions arising from climate change. Several statistical methodologies referred to as bias correction methods, such as Quantile Mapping (QM) approaches have been proposed to correct the model outputs so that the results can be used for impact studies. Although QM approaches can correct the entire distribution of a climate variable, they are based on an assumption that climate model biases are stationary over time; however, owing to climatic nonstationarity, this assumption may not hold true as mentioned in the literature. Hence, alternative approaches that tackle the nonstationarity of the parameters are found in the recent literature, such as EDCDFm, equiratio CDFm, CNCDFm, etc. These methods explicitly take into account the changes between the reference and future distributions that consequently consider the changes of variables over time. Although these methods adjust all the moments by maintaining the rank correlation between the model and observation in training period, still they fail to realistically correct the extreme events. In the present research it is attempted to improve these methods for bias correction by taking into account the nonstationarity aspect. The future period data is bias corrected based on the non-parametric quantile mapping approach considering the cumulative distribution of both control period and test period model data which accounts for the non-stationarity. In addition to this two different approaches are applied; first the mean of control period data is also included in the correction, and then the standard deviation is separately included, as presented in the equation (1) and (2), respectively.
MoreTranslated text
AI Read Science
Must-Reading Tree
Example
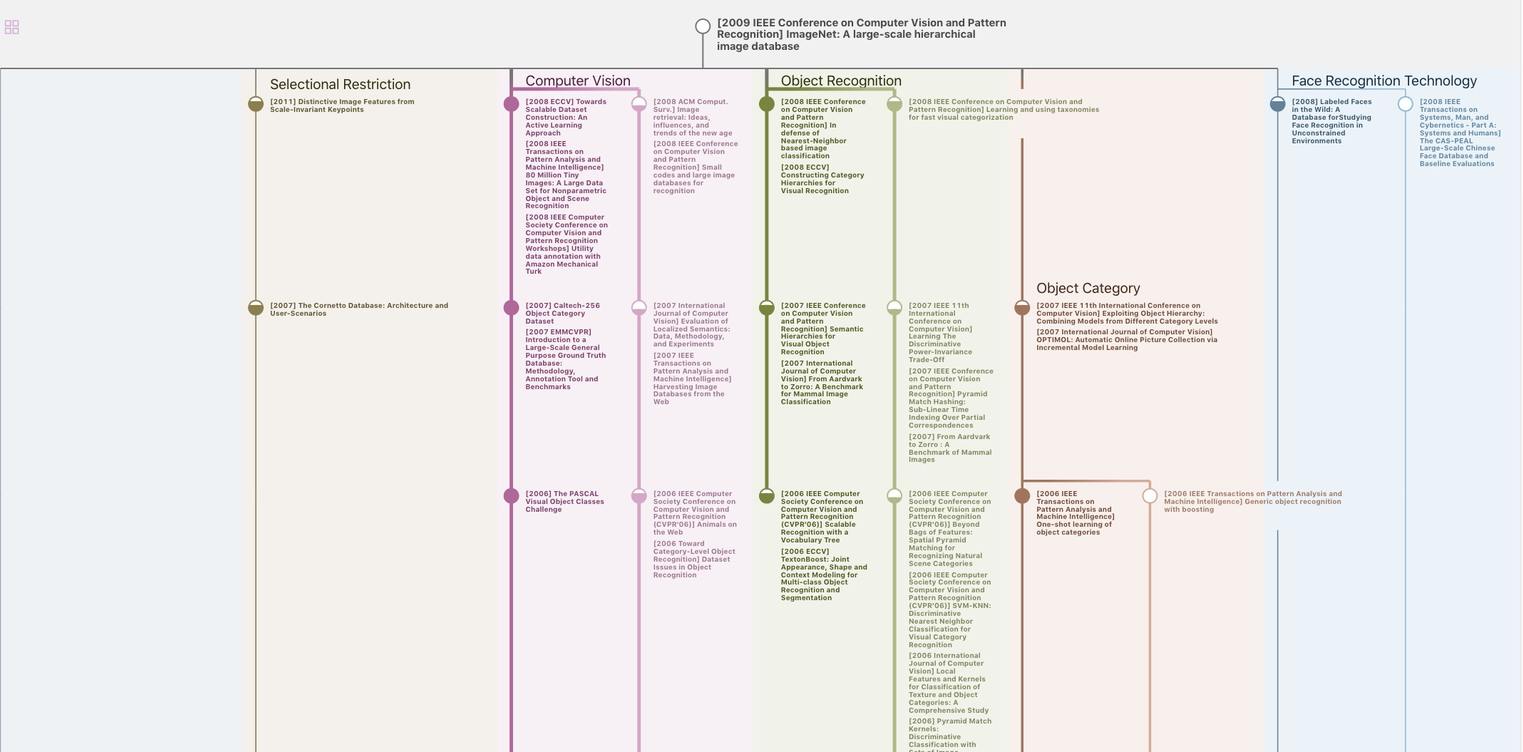
Generate MRT to find the research sequence of this paper
Chat Paper
Summary is being generated by the instructions you defined