Examining Committee
semanticscholar(1997)
摘要
Heterogeneous computing platforms that use GPUs and CPUs in tandem for computation have become an important choice to build low-cost high-performance computing platforms. The computing ability of modern GPUs surpasses that of CPUs can offer for certain classes of applications. GPUs can deliver several Tera-Flops in peak performance. However, programmers must adopt a more complicated and more difficult new programming paradigm. To alleviate the burden of programming for heterogeneous systems, Garg and Amaral developed a Python compiling framework that combines an ahead-of-time compiler called unPython with a just-in-time compiler called jit4GPU. This compilation framework generates code for systems with AMD GPUs. We extend the framework to retarget it to generate OpenCL code, an industry standard that is implemented for most GPUs. Therefore, by generating OpenCL code, this new compiler, called jit4OpenCL, enables the execution of the same program in a wider selection of heterogeneous platforms. To further improve the target-code performance on nVidia GPUs, we developed an array-access analysis tool that helps to exploit the data reusability by utilizing the shared (local) memory space hierarchy in OpenCL. The thesis presents an experimental performance evaluation indicating that, in comparison with jit4GPU, jit4OpenCL has performance degradation because of the current performance of implementations of OpenCL, and also because of the extra time needed for the additional just-in-time compilation. However, the portable code generated by jit4OpenCL still have performance gains in some applications compared to highly optimized CPU code.
更多查看译文
AI 理解论文
溯源树
样例
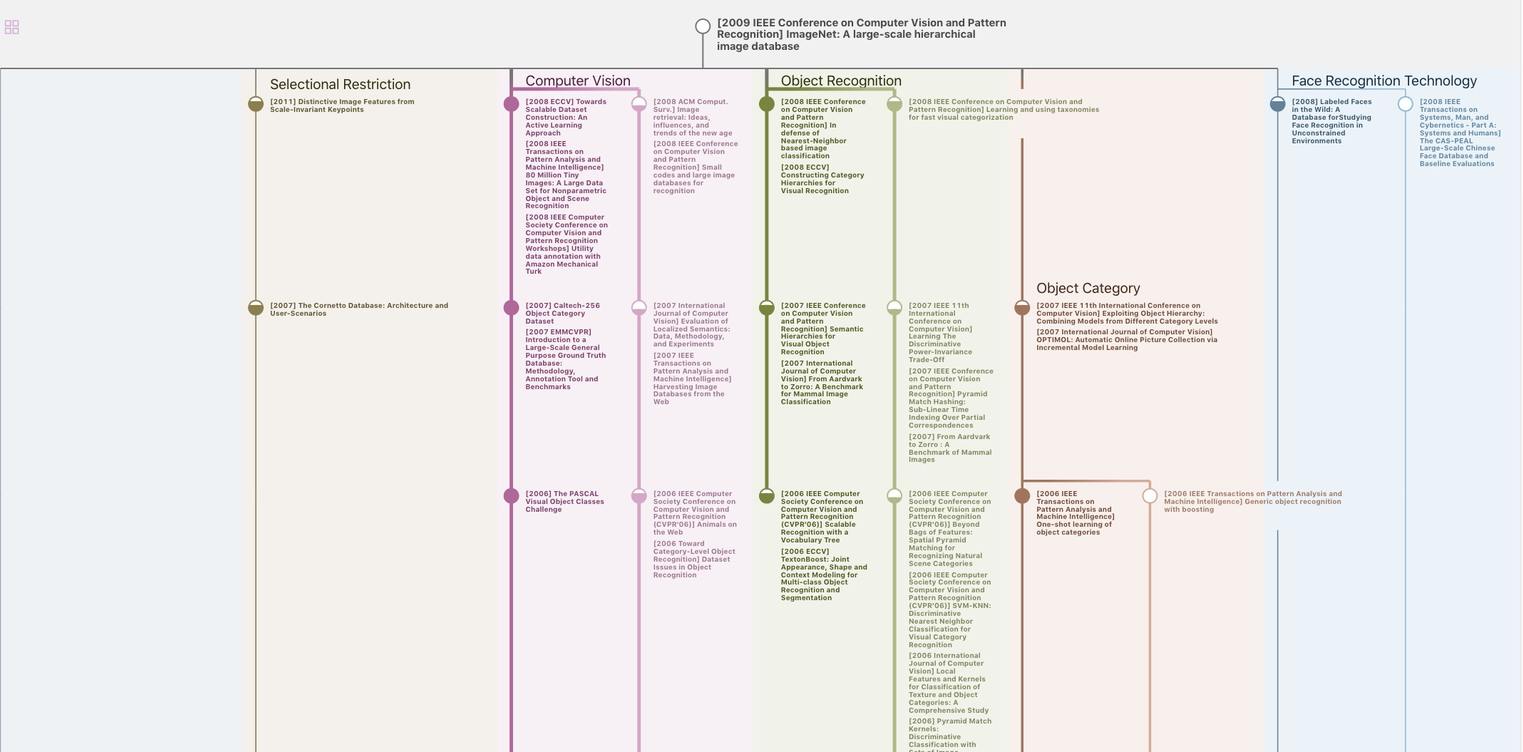
生成溯源树,研究论文发展脉络
Chat Paper
正在生成论文摘要