On the Equivalence of Sparse Statistical Problems
semanticscholar(2017)
摘要
Sparsity is a widely used and theoretically well understood notion that has allowed inference to be statistically and computationally possible in the high-dimensional setting. Sparse Principal Component Analysis (SPCA) and Sparse Linear Regression (SLR) are two problems that have a wide range of applications and have attracted a tremendous amount of attention in the last two decades as canonical examples of statistical problems in high dimension. A variety of algorithms have been proposed for both SPCA and SLR, but their literature has been disjoint for the most part. We have a fairly good understanding of conditions and regimes under which these algorithms succeed. But is there be a deeper connection between computational structure of SPCA and SLR? In this paper we show how to efficiently transform a blackbox solver for SLR into an algorithm for SPCA. Assuming the SLR solver satisfies prediction error guarantees achieved by existing efficient algorithms such as those based on the Lasso, we show that the SPCA algorithm derived from it achieves state of the art performance, matching guarantees for testing and for support recovery under the single spiked covariance model as obtained by the current best polynomial-time algorithms. Our reduction not only highlights the inherent similarity between the two problems, but also, from a practical standpoint, it allows one to obtain a collection of algorithms for SPCA directly from known algorithms for SLR. Experiments on simulated data show that these algorithms perform well. Thesis Supervisor: Guy Bresler Title: Assistant Professor
更多查看译文
AI 理解论文
溯源树
样例
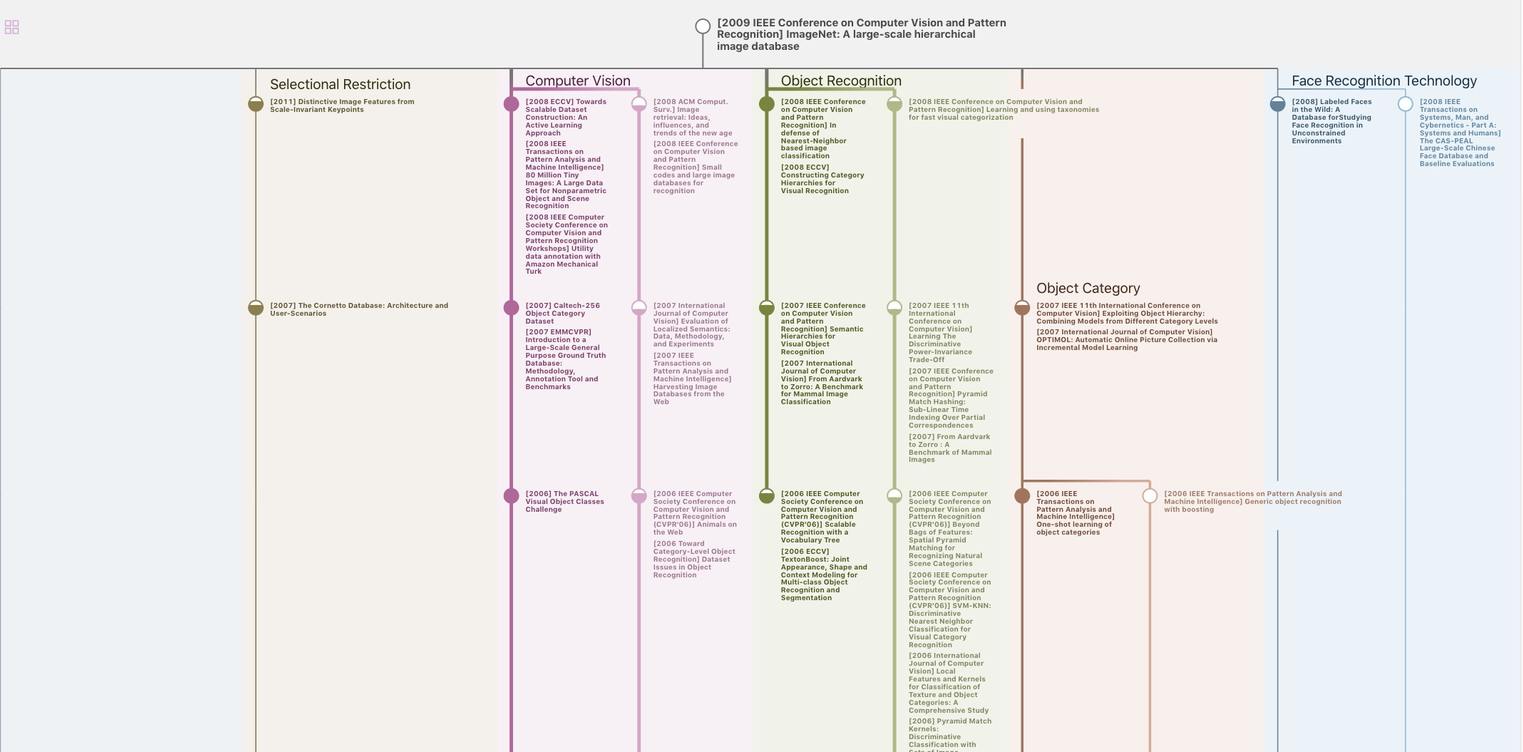
生成溯源树,研究论文发展脉络
Chat Paper
正在生成论文摘要