Unsupervised Risk Discovery for Child Welfare Screening
semanticscholar(2019)
摘要
Child protection agencies across the US are routinely tasked with the screening millions of cases in order to identify those that are likely to result in adverse outcomes. To help with this task, many agencies have implemented and deployed risk prediction models that use administrative data in order to aid call workers. Nonetheless, the use of these predictive models for child welfare has proven onerous, raising concerns that go from how to validate their efficacy, to how to guarantee the fair treatment of disadvantaged groups. In particular, one concern that has inspired significant recent work is the fact that the variable of interest is never observed: we never see what happens to a child if the case was not investigated. In this work, we propose to tackle this obstacle by using unsupervised methods to discover latent risk structures in the agencies’ administrative data, completely bypassing the “missing labels” predicament. We formulate two different risk models (with and without time dependencies among calls) and show (1) that the risk structures learned by both models are meaningful for experts, and (2) that taking into account time dependencies ends up hurting the performance on downstream predictive tasks.
更多查看译文
AI 理解论文
溯源树
样例
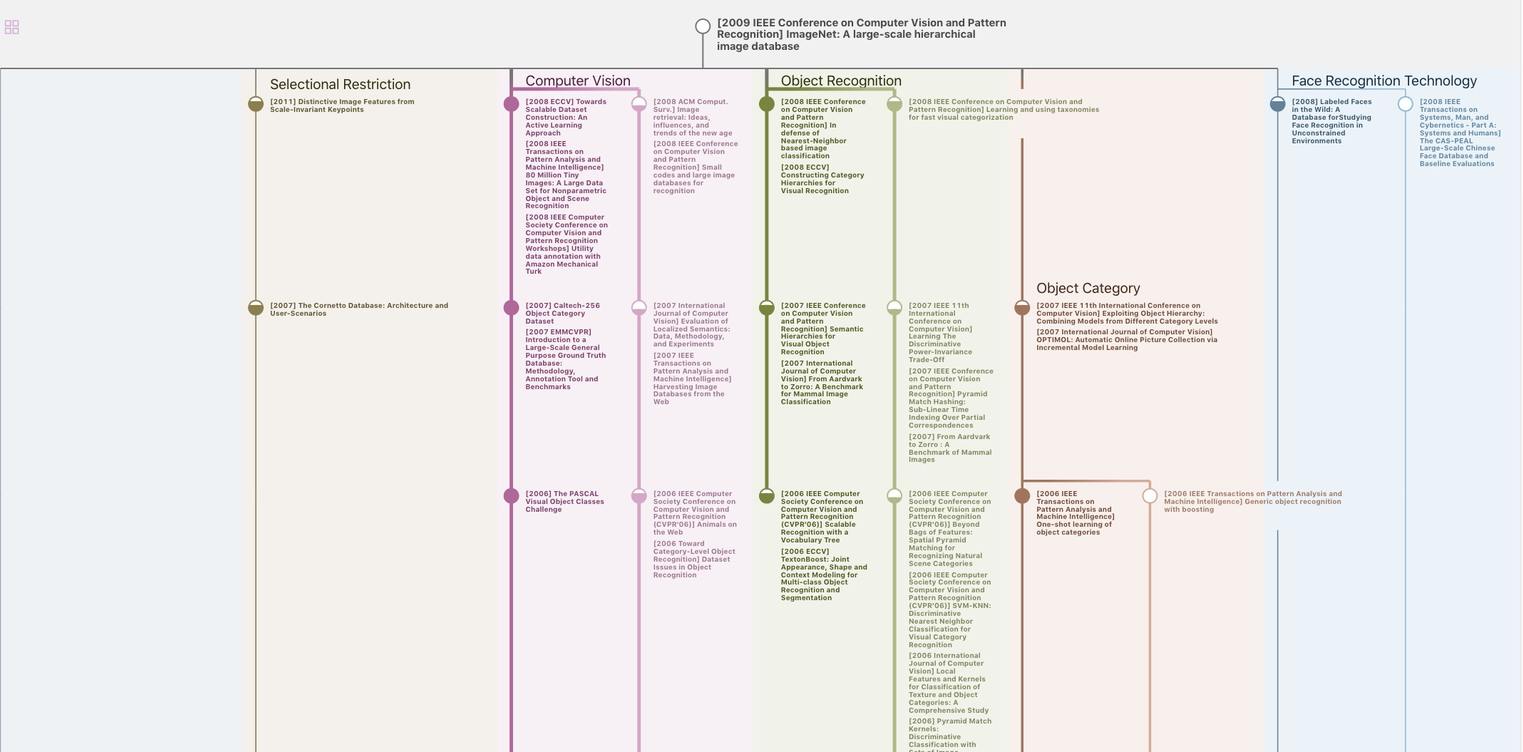
生成溯源树,研究论文发展脉络
Chat Paper
正在生成论文摘要