Effect of Nonnormality on Test Statistics for One-Way Independent Gr
semanticscholar(2017)
摘要
The data obtained from one-way independent groups designs is typically nonnormal in form and rarely is equally variable across treatment populations (i.e., population variances are heterogeneous). Consequently, the classical test statistic that is used to assess statistical significance [i.e., the analysis of variance (ANOVA) F-test] typically provides invalid results (e.g., too many Type I errors, reduced power). For this reason, there has been considerable interest in finding a test statistic that is appropriate under conditions of nonnormality and variance heterogeneity. Previously recommended procedures for analyzing such data include the James (1951) test, the Welch (1951) test applied either to the usual least squares estimators of central tendency and variability, or the Welch test with robust estimators, i.e., trimmed means and Winsorized variances. A new statistic proposed by Krishnamoorthy, Lu and Mathew (2007), intended to deal with heterogeneous variances, though not nonnormality, uses a parametric bootstrap procedure. In their investigation of the parametric bootstrap test, the authors examined its operating characteristics under limited conditions and did not compare it to the Welch test based on robust estimators. Thus, we investigated how the parametric bootstrap procedure, and a modified parametric bootstrap procedure based on trimmed means, perform relative to previously recommended procedures when data are nonnormal and heterogeneous. The results indicated that the tests based on trimmed means offer the best Type I error control and power when variances are unequal and at least some of the distribution shapes are nonnormal. Effects of Nonnormality on Test Statistics 3 Effects of Nonnormality on Test Statistics for One-Way Independent Groups Designs A common question in the behavioural sciences is whether treatment groups differ on an outcome variable. For example, a researcher may be interested in determining if eating disorder symptomatology (e.g., obsession with weight) vary across different cultural backgrounds. The procedure that is most popular for analyzing data from one-way independent groups designs is the analysis of variance (ANOVA) F-test. The ANOVA can be a valid and powerful test for identifying treatment effects; but, when the validity assumptions underlying the test are violated, the results from the test are typically unreliable and invalid. One mathematical validity assumption of the test (i.e., a condition that was stipulated in order to derive the test statistic) is that the distribution of each population is normal in form. Although this is assumed by most researchers, it is very often not the case (Micceri, 1989). Nonnormality can have deleterious effects on the F-test, where predominantly there is a lack of sensitivity to detect treatment effects (Wilcox, 1997). As well, there is an increased risk that null effects will be falsely declared statistically significant (i.e., an elevated probability of committing a Type I error), especially when sample sizes are small. A second mathematical restriction that was adopted when deriving the test statistic was that the population variances be equal. It is well known that unequal variances are the norm, rather than the exception, with behavioral science data (Erceg-Hurn & Mirosevich, 2008; Golinski & Cribbie, 2009; Grissom, 2000; Keselman et al., 1998), with largest to smallest group ratios greater than ten not uncommon (Grissom, 2000; Wilcox, 1987). Moreover, unequal variances can have drastic effects on the reliability and validity of the F-test, especially when Effects of Nonnormality on Test Statistics 4 group sample sizes are also unequal (Glass, Peckham & Sanders, 1972; Harwell, Rubenstein, Hayes & Olds, 1992; Kohr & Games, 1974; Scheffé, 1959). When distributions are nonnormal and variances are unequal, the empirical probability of a Type I or Type II error for the F-test can deviate even more substantially from the nominal levels than when either assumption is independently violated (Glass, Peckham & Sanders, 1972; Luh & Guo, 2001). Several procedures have been recommended for analyzing the data from one-way independent groups designs when distributions are nonnormal and variances are unequal (e.g., Brunner, Dette, & Munk, 1997; Cribbie, Wilcox, Bewell & Keselman, 2007; Wilcox & Keselman, 2003). Currently, the most recommended approaches involve utilizing the James (1951) or Welch (1951) heteroscedastic F-tests (based on the usual least squares estimators), or the Welch heteroscedastic F-test with trimmed means and Winsorized variances. Several studies have demonstrated that the original James and Welch procedures are generally robust (with respect to Type I errors and power) when group variances and sample sizes are extremely unequal (e.g., Kohr & Games, 1974; Krisnamoorty, Lu & Mathew, 2007), and further that the test is robust to unequal variances and nonnormal data, as long as the nonnormality is mild to moderate (Algina, Oshima, & Lin, 1994). The Welch test with trimmed means and Winsorized variances has also been shown to provide excellent Type I error control and power even under extreme violations of the normality and variance equality assumptions (Keselman, Wilcox, Othman & Fradette, 2002). An important condition of nonnormality that has received very little attention in the methodological literature is the case of dissimilar distribution shapes across treatment groups. For example, it is not uncommon for behavioral science researchers to encounter one group with Effects of Nonnormality on Test Statistics 5 an approximately normal distribution and another group with a skewed distribution. For example, Leentjens, Wielaert, van Harskamp and Wilmink (1998) found that scores on many measures of nonverbal aspects of language (i.e., prosody) were normally distributed in control groups, but were extremely skewed in schizophrenic patients. Wilcox (2005) notes that skewed distributions in general are not as problematic as when groups have different amounts of skewness. Indeed, Tiku (1964) explored situations where skew differed between groups and found that Type I and Type II errors were adversely affected when groups are skewed in opposite directions, especially with smaller sample sizes. It is important to point out that when distribution shapes are dissimilar, isolating the specific nature of the differences in the distributions is an important part of the data analysis (and comparisons of central tendencies may be less informative). For example, when distribution shapes are dissimilar, alternative descriptive statistics, such as the specific quantiles (e.g., 10th, 25th, 75th, 90th) for each distribution, can be useful in understanding differences between the distributions. Further, if one suspects that distribution shapes might be dissimilar, it might be fruitful to explicitly test for differences in the distributions using a runs test, such as the Wald-Wolfowitz, or a test of a common distribution, such as the Kolmogorov-Smirnov or Cramer-von Mises tests (see Sprent & Smeeton, 2001, pages 185-188). For example, in the Leentjens et al. (1998) study described above, the goal of the researchers was to compare the central tendencies of the groups, although specific tests used to isolate differences in the shapes of the distributions may have also been informative. Thus, when distribution shapes differ, researchers may be interested in exploring differences in the central tendencies, exploring the nature of the distributional differences, or both. Since the underlying goal of most studies in psychology that involve comparing groups is to compare the Effects of Nonnormality on Test Statistics 6 central tendencies, this study addresses the important question of how available test statistics perform under these conditions. The parametric bootstrap procedure proposed by Krishnamoorthy et al. (2007) is a relatively new statistic for comparing the means of independent groups when the variances of the groups are unequal. This test involves generating sample statistics from parametric models, where the parameters in the model are replaced by their estimates (see below for details regarding the parametric bootstrap procedure). This procedure was found by the authors to provide a better balance of Type I error control and power than the original Welch (1951) procedure, especially when sample sizes were small and the number of groups was large. There are, however, important questions that were not explored by Krishnamoorthy et al. (2007). For example, how well will the Krishnamoorthy et al. procedure perform (with respect to controlling Type I and II error rates) when distribution shapes are nonnormal? This question is important because, as discussed earlier, distributions in the behavioural sciences are rarely normal. An important point related to this issue is how to distinguish between a normally distributed variable and nonnormally distributed variable. Although numerous test statistics have been proposed for detecting deviations from normality (e.g., Chen & Shapiro, 1995; D’Agostino, 1971; Shapiro & Wilk, 1965), it is also important to consider that: 1) the performance of tests of normality are greatly affected by sample size, the form of nonnormality, etc. (Seier, 2002); 2) graphical methods (e.g, histograms, boxplots, normal quantile plots) can sometimes be as informative as tests of normality for detecting deviations from normality (Holgersson, 2006); and most importantly, 3) the power of many traditional parametric tests can be severely affected by even slight deviations from normality (Wilcox, 2005). Therefore, even though there is Effects of Nonnormality on Test Statistics 7 subjectivity in deciding whether or not a distribution is normal, it is important that we are aware of how various test statistics perform under different degrees of nonnormality in order to be able to make informed recommendations regarding the appr
更多查看译文
AI 理解论文
溯源树
样例
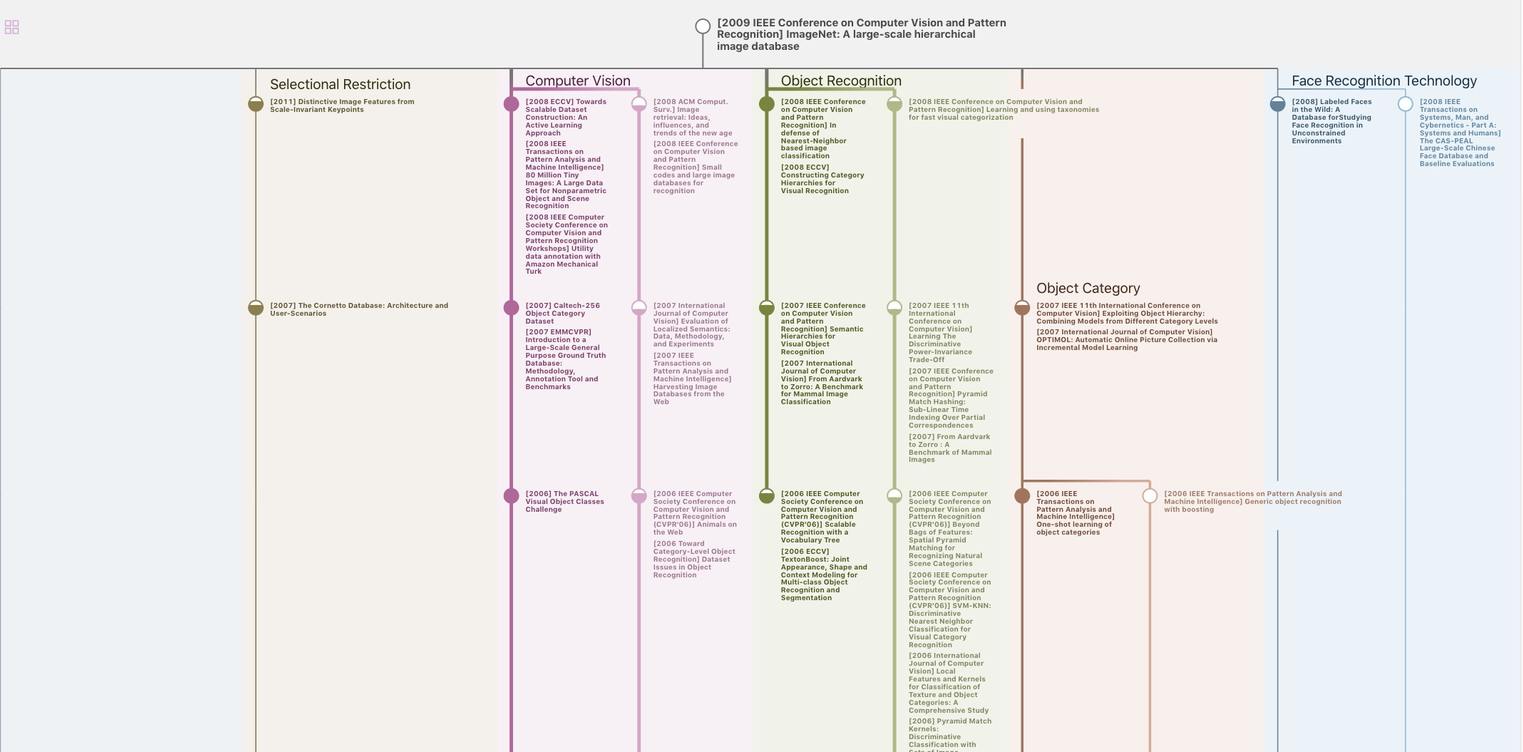
生成溯源树,研究论文发展脉络
Chat Paper
正在生成论文摘要