Automatic Segmentation of Hippocampal Subfields in T 2-Weighted In Vivo MRI
semanticscholar(2009)
摘要
Introduction The hippocampal formation is a critically important structure in the study of Alzheimer’s disease (AD) and other disorders affecting memory. Substantial evidence has been generated in support of using MRI-derived measures of hippocampal volume and thickness as biomarkers for disease progression and treatment monitoring in clinical trials of disease-modifying drugs for AD [1,2]. Yet the hippocampal formation is a complex and heterogeneous anatomical structure, formed by several subfields with distinct functional roles and varying levels of vulnerability to AD neuropathology. In recent years, there has been a push to refine hippocampal morphometry, so as to yield subfield-specific biomarkers. Some approaches have taken a surface-based view, where the boundary of the hippocampus is divided into patches corresponding to subfields [3,4]. Such approaches give limited insight into changes in the dentate gyrus, which is largely buried under the hippocampal boundary. Others [5,6] have used specialized MRI sequences that reveal the dark band between the CA1 and dentate gyrus subfield, allowing direct delineation of subfields based on available strong intensity cues. Particularly, in [6], subfield volumetry in such data was found to correlate with known neuropathological findings when examining group differences between AD patients, MCI patients and healthy controls. However, such volumetry currently requires manual delineation of subfields, which is costly and limits scalability. To address this problem, we present a novel computational method for automatic subfield segmentation in hippocampus-targeting T2-weighted MRI. To our knowledge, this is the first such method to be applied and evaluated in MRI data that can be acquired in the clinical setting (3 Tesla, <10 min acq. time). Materials and Methods MRI data in 33 subjects (14 controls, 19 MCI patients) were acquired on a Brucker Med-Spec 4T system equipped with an 8-channel array coil. T2weighted images of a slab oriented orthogonally to the long axis of the hippocampal formation were acquired with a FSE sequence (TR/TE=3500/19 ms, ETL=15, ESP = 18.6 ms, FA = 160, 0.4x0.4x2.0 mm resolution, 5:30 min acq. time). Whole-brain T1-weighted MP-RAGE images were also acquired. We have found that image quality and contrast is comparable when the same sequences are applied on a clinical-grade 3T Siemens Trio scanner. Manual segmentation of subfields was performed, providing training and validation data for automatic segmentation. The protocol in [6] was refined to include additional slices anteriorly and posteriorly, and to include more subfields: cornu ammonis fields CA1, CA2, CA3, dentate gyrus (DG), subiculum (SUB), parahippocampal gyrus (PHG), entorhinal cortex (ERC). Initially, the hippocampal formation is partitioned into “head,” “tail,” and “body” sections along slice boundaries. The body section, typically spanning 5-8 slices, is partitioned into subfields listed above. Reliability was established by having two raters (JP, CC) repeat segmentation in 10 subjects. Two segmentation pipelines were implemented. The first semi-automatic pipeline requires the whole hippocampus ROI (consisting of CA1-3 and DG subfields) as the input. Such an ROI is substantially less labor-intensive to generate than subfield segmentations. The second pipeline is fully automatic. The semi-automatic pipeline uses shape-based normalization [7] to map probability maps of subfield spatial distribution from atlas space to subject space. The fully automatic pipeline uses multi-modality (T1+T2) deformable image registration [8] to perform a similar mapping. In both methods, subfield spatial distribution probability maps are used to generate an initial segmentation. AdaBoost classifiers are trained to recognize voxels wrongly labeled by the initial segmentation and to correct them. To evaluate segmentation performance, a 3-fold cross-validation strategy is implemented with 22 randomly selected subjects used to train the classifier and 11 subjects used to test classifier/segmentation performance. Results Examples of automatic and semi-automatic segmentation are shown in Figure 1. Segmentation accuracy is evaluated by computing the Dice overlap coefficient between subfields segmented automatically and manually (Table 1). For comparison, inter-rater and intra-rater reliability of manual segmentation is summarized using the same two metrics. Overall, the semi-automatic method achieves high accuracy. However, it must be noted that errors made by this method are restricted to subfield boundaries inside the hippocampus ROI, as the boundaries of this ROI are given a priori. The fully automatic method must infer both the whole-hippocampus and subfield boundaries, which leads to lower overall accuracy. Discussion Overall, it appears that larger subfields (CA1, DG) can be segmented automatically in T2-weighted MRI with accuracy comparable to wholehippocampus automatic segmentation in T1-weighted data [9]. Smaller subfields pose greater challenges. We anticipate that improvements to the registration algorithm and landmark-based user guidance may improve the accuracy of fully automatic segmentation substantially. Acknowledgments This work is supported by NIH grants K25 AG027785, R21 NS061111, R01 AG010798 and Penn-Pfizer Alliance grant 10295. References [1] Jack CR et al., Neurology 60:253-260, 2003 [2] de Leon MJ et al., Neurobiology of Aging, 27:394-401, 2006 [3] Wang L et al., Neuroimage, 30:52-60, 2006 [4] Apostolova LG et al., Brain, 129:2867-73, 2006 [5] Zeineh MM et al., Science, 299:577-80, 2003 [6] Mueller SG and Weiner MW, Hippocampus, 19:558-64, 2009 [7] Yushkevich PA et al., Neuroimage, 35:1516-30, 2007 [8] Avants BB et al., Medical Image Analysis, 12:26-41, 2008 [9] Carmichael OT et al., Neuroimage, 27:979-90, 2005
更多查看译文
AI 理解论文
溯源树
样例
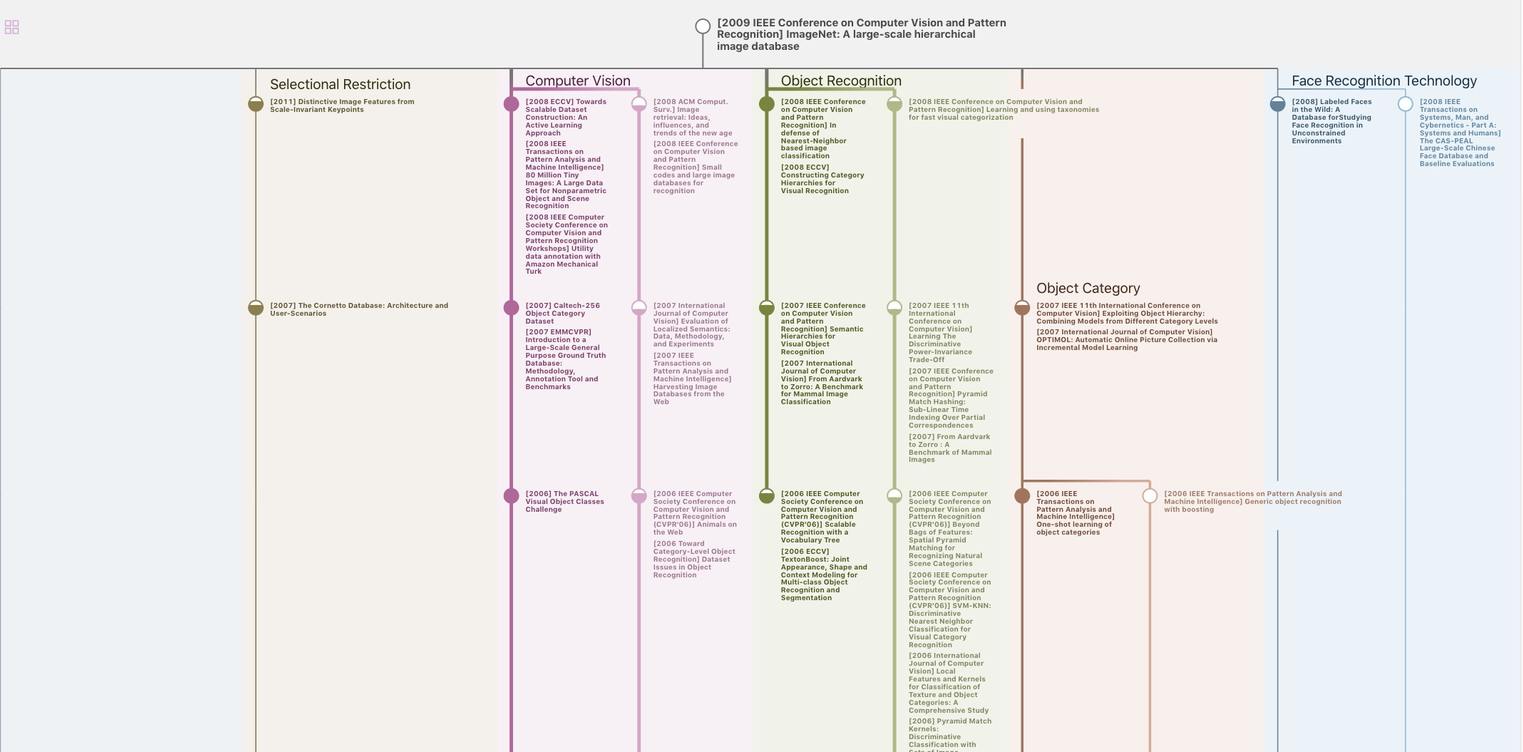
生成溯源树,研究论文发展脉络
Chat Paper
正在生成论文摘要