Prediction of Frost Events using Bayesian networks and Random Forest
semanticscholar(2019)
摘要
In a couple of hours, farmers can lose everything because of frost events. Handling frost events is possible by using a number of countermeasures such as heating or removing the surrounding air among crops. Given the socio-economical implications of this problem, involving not only loss of jobs but also valuable resources, there have been a number of efforts to design a system to predict frost events, but with partial success: either they are based on formulas needing many unknown coefficients, or the prediction performance is poor. In this paper we propose a new approach, which instead of using the sensor’s past information for prediction, as state of the art methods do, we assume that frost prediction in one location could be improved by using the information of the most relevant neighboring sensors. However, given the small amount of frost events during the year, available data, even after incorporating information of neighboring sensors, it is not enough to build an accurate frost forecasting system, which defines an unbalanced dataset problem. In order to overcome this disadvantage, we propose to use machine learning algorithms such as Bayesian networks and Random Forest where the training set includes new samples using SMOTE (Synthetic Minority Oversampling Technique). Our results show that selecting the most relevant neighbors and training the models with SMOTE increases significatively the frost detection rate of the predictor, turning the results into a useful resource for decision makers.
更多查看译文
AI 理解论文
溯源树
样例
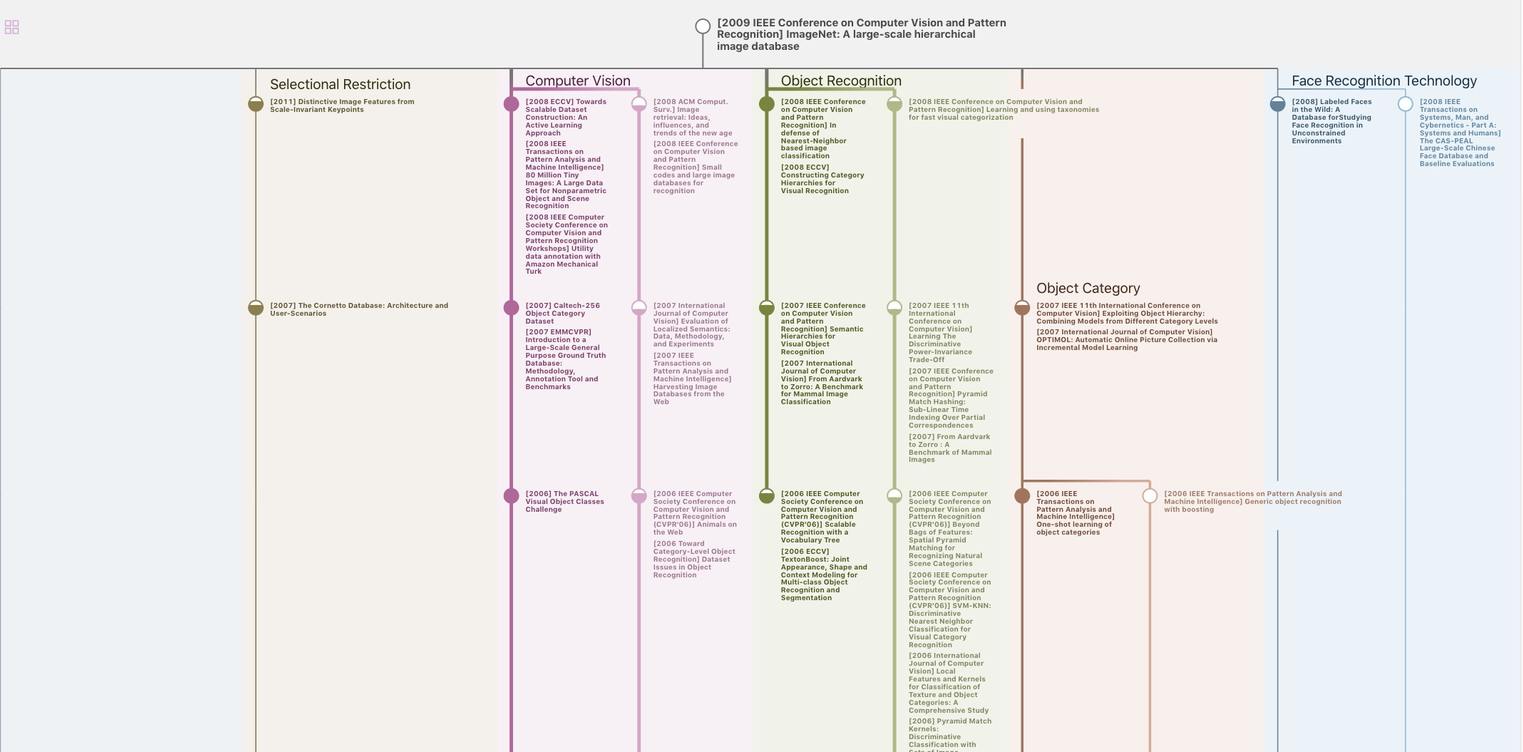
生成溯源树,研究论文发展脉络
Chat Paper
正在生成论文摘要