Feature discovery and Hebbian learning
semanticscholar(2017)
摘要
Real-world pattern recognition problems often involve data spaces of exceedingly large dimension. In order to ease the computational burden on pattern-classifier algorithms, the naive, high-dimensional features can be encoded in a lower dimensional set. Principal component analysis (PCA) provides a means for this encoding. More importantly, PCA can be implemented in neural networks that use local, Hebbian learning rules to change synaptic strengths. Thus, data encoding can be accomplished in the same environment that serves the computational needs of the classifier. This report discusses PCA from statistical and geometric points of view, and its implementation in neural networks. 1 Feature Discovery by Hebbian Learning 1.
更多查看译文
AI 理解论文
溯源树
样例
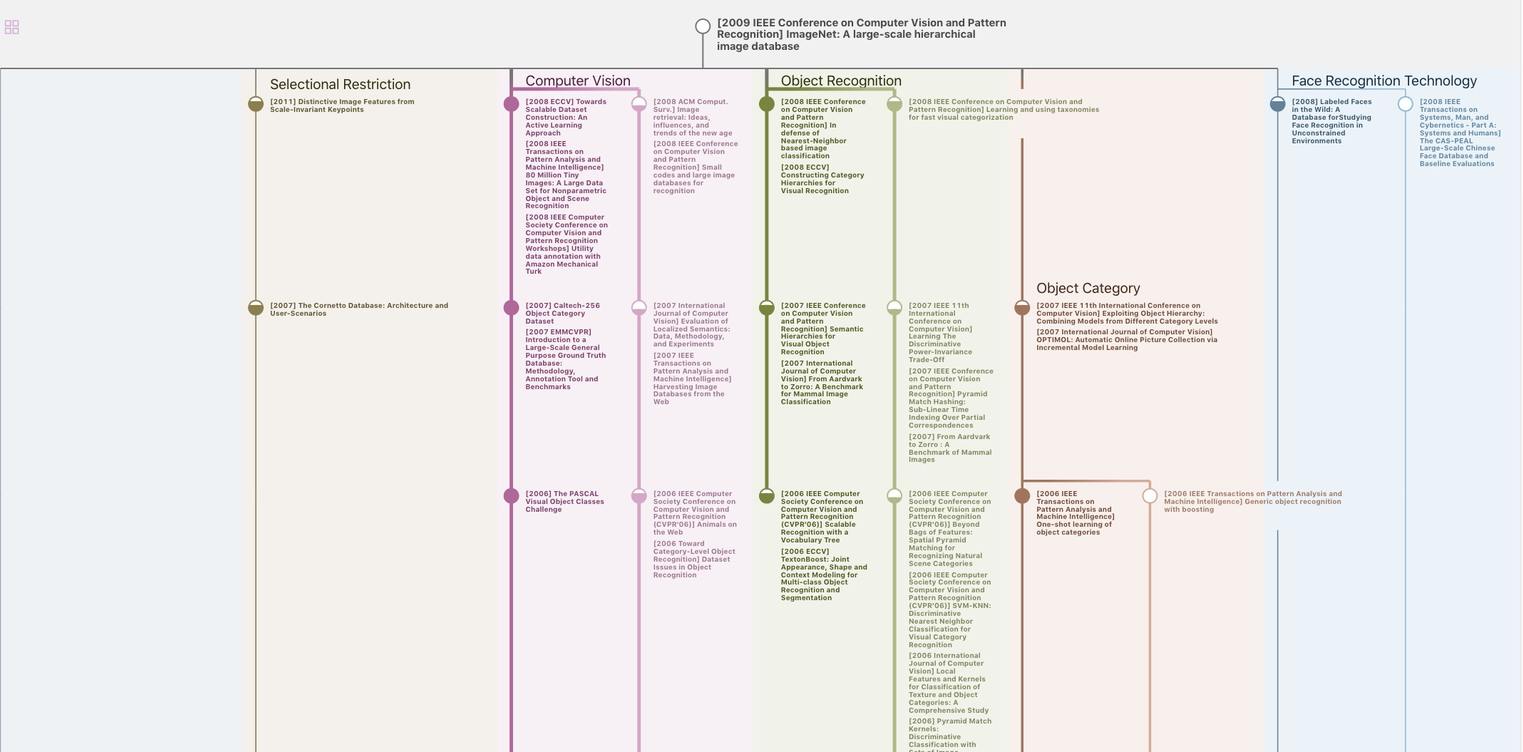
生成溯源树,研究论文发展脉络
Chat Paper
正在生成论文摘要