Feature-Matching Auto-Encoders
semanticscholar(2017)
摘要
We examine how learning from unaligned data can improve both the data efficiency of supervised tasks as well as enable alignments without any supervision. For example, consider unsupervised machine translation: the input is two corpora of English and French, and the task is to translate from one language to the other but without any pairs of English and French sentences. To address this, we develop feature matching auto-encoders (FMAEs). FMAEs ensure that the marginal distribution of feature layers is preserved across forward and inverse mappings between domains. FMAEs achieve state of the art for semi-supervised neural machine translation with significant BLEU score differences of up to 5.7 and 6.3 over traditional supervised models. Furthermore, on English-to-German, FMAEs outperform last year’s best models such as ByteNet [8] while using only half as many supervised examples.
更多查看译文
AI 理解论文
溯源树
样例
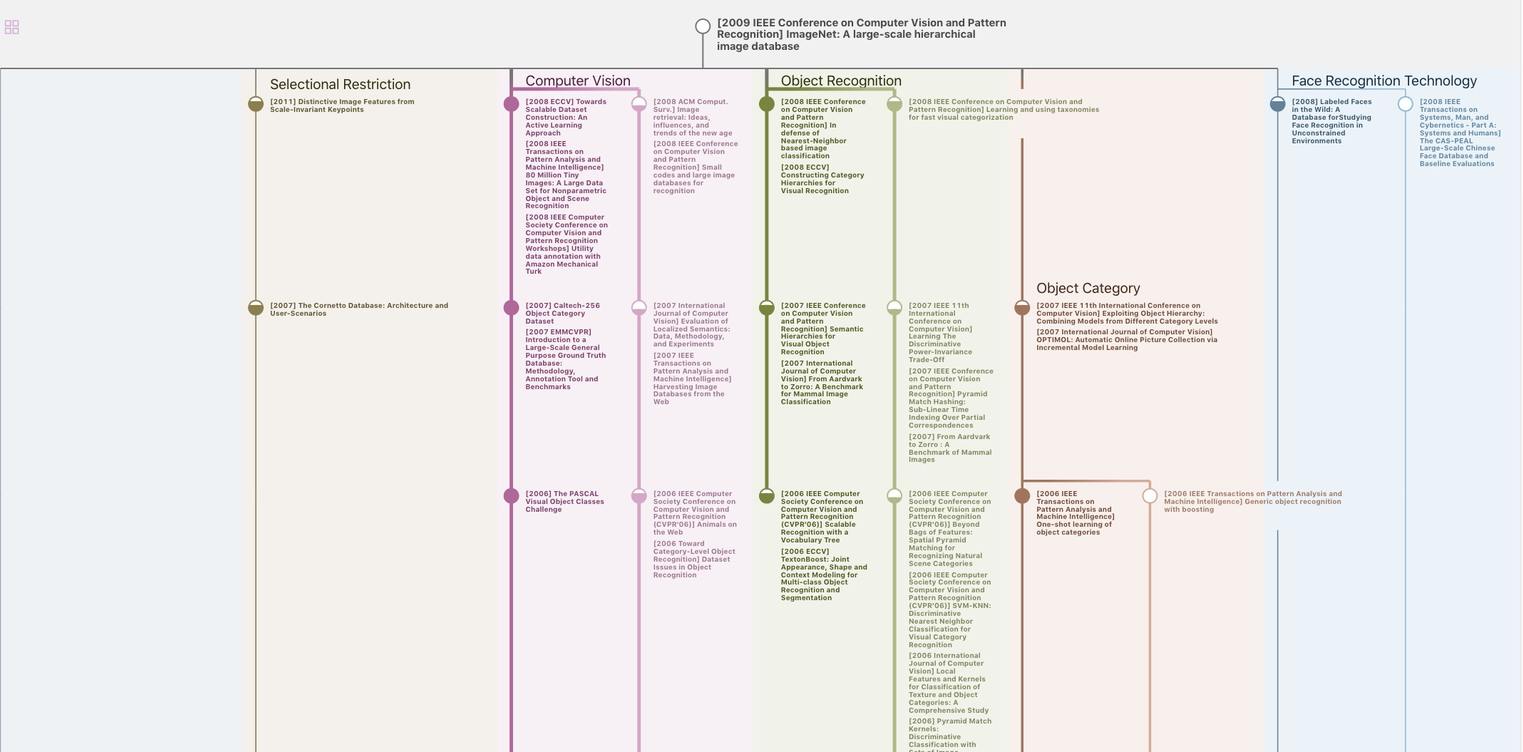
生成溯源树,研究论文发展脉络
Chat Paper
正在生成论文摘要