Lecture Lecture 15 : Distribution testing : uniformity , identity
semanticscholar(2019)
摘要
Recall that last time we considered the problem of uniformity testing : Given a distribution D over [n], we want to distinguish between D being uniform and D being -far from uniform. In particular, we saw that ‖D‖2 = 1 n when D is uniform and ‖D‖ 2 2 > 1 n + 2 n if D is -far from uniform. Given m samples from D, we hope to be able to do distinguish between these cases using a number of samples that is sublinear in n. We saw before that the algorithm for uniformity testing works as follows: first compute C := #collisions. If C M < 1 n + 2 2n output “uniform”; otherwise, output “ -far from uniform”. Throughout this lecture, we let d = ‖D‖2 for simplicity of notation. We saw last time that E[ C M ] = d, where M := ( m 2 ) .
更多查看译文
AI 理解论文
溯源树
样例
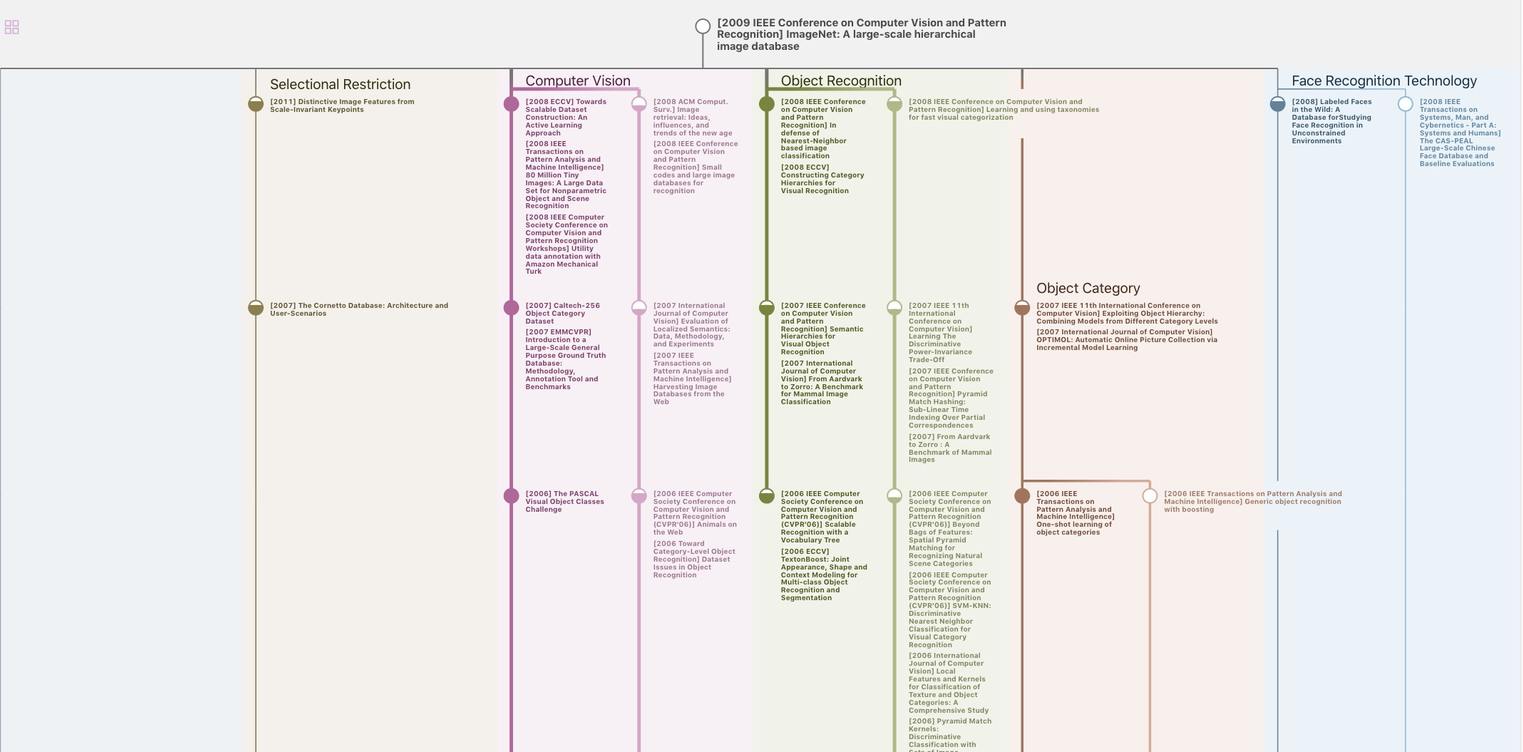
生成溯源树,研究论文发展脉络
Chat Paper
正在生成论文摘要