Forecasting El Niño with Convolutional and Recurrent Neural Networks
semanticscholar(2019)
摘要
The El Niño Southern Oscillation (ENSO) is the dominant mode of variability in the climate system on seasonal to decadal timescales. With foreknowledge of the state of ENSO, stakeholders can anticipate and mitigate impacts in climate-sensitive sectors such as agriculture and energy. Traditionally, ENSO forecasts have been produced using either computationally intensive physics-based dynamical models or statistical models that make limiting assumptions, such as linearity between predictors and predictands. Here we present a deep-learning-based methodology for forecasting monthly ENSO temperatures at various lead times. While traditional statistical methods both train and validate on observational data, our method trains exclusively on physical simulations. With the entire observational record as an out-of-sample validation set, the method’s skill is comparable to that of operational dynamical models. The method is also used to identify disagreements among climate models about the predictability of ENSO in a world with climate change.
更多查看译文
AI 理解论文
溯源树
样例
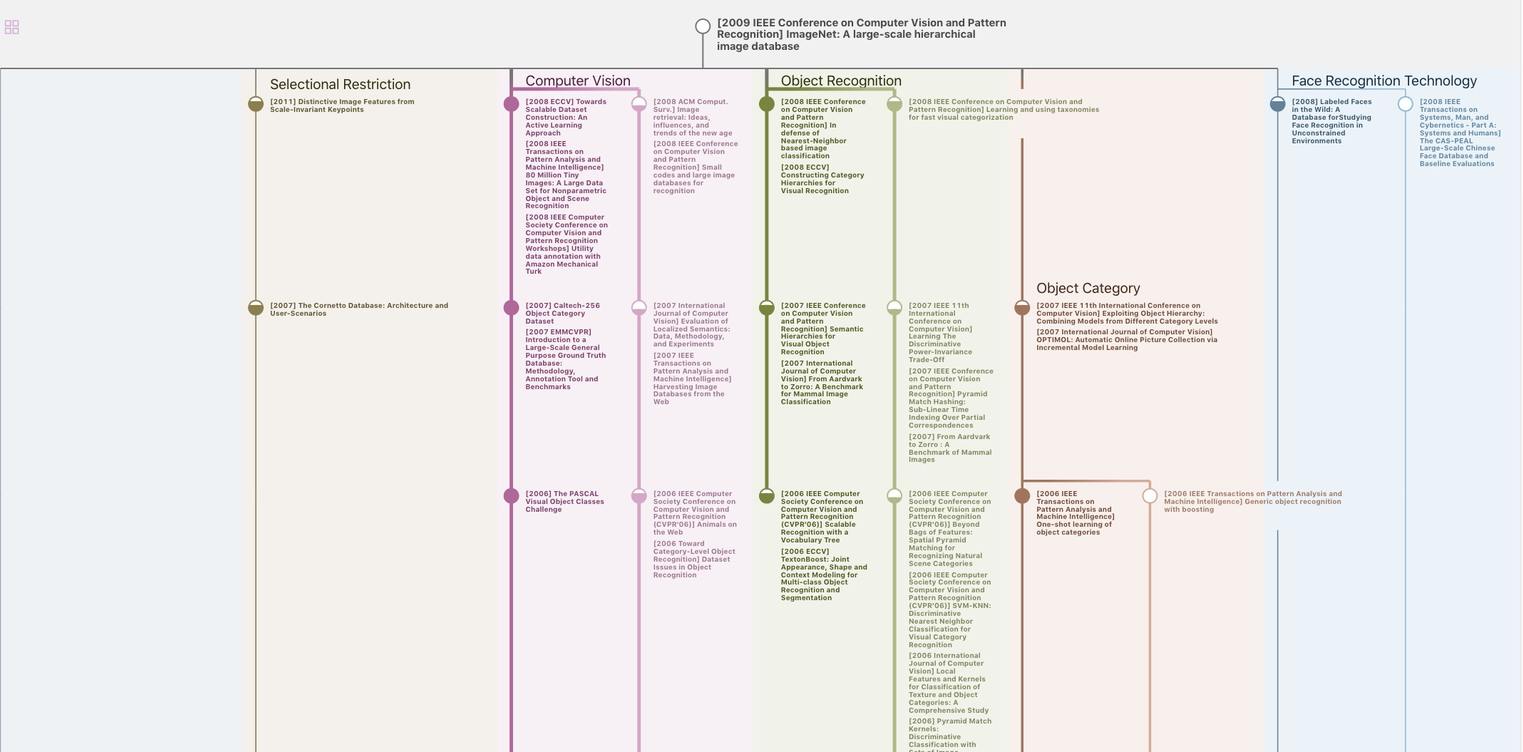
生成溯源树,研究论文发展脉络
Chat Paper
正在生成论文摘要