Logically Scalable and Efficient Relational Learning
semanticscholar(2018)
摘要
Relational learning algorithms learn the definition of a new relation in terms of existing relations in the database. The same database may be represented under different schemas for various reasons, such as efficiency, data quality, and usability. Unfortunately, the output of current relational learning algorithms tends to vary quite substantially over the choice of schema, both in terms of learning accuracy and efficiency. We introduce the property of schema independence of relational learning algorithms, and study both the theoretical and empirical dependence of existing algorithms on the common class of (de) composition schema transformations. We show theoretically and empirically that current relational learning algorithms are generally not schema independent. We propose Castor, a relational learning algorithm that achieves schema independence.
更多查看译文
AI 理解论文
溯源树
样例
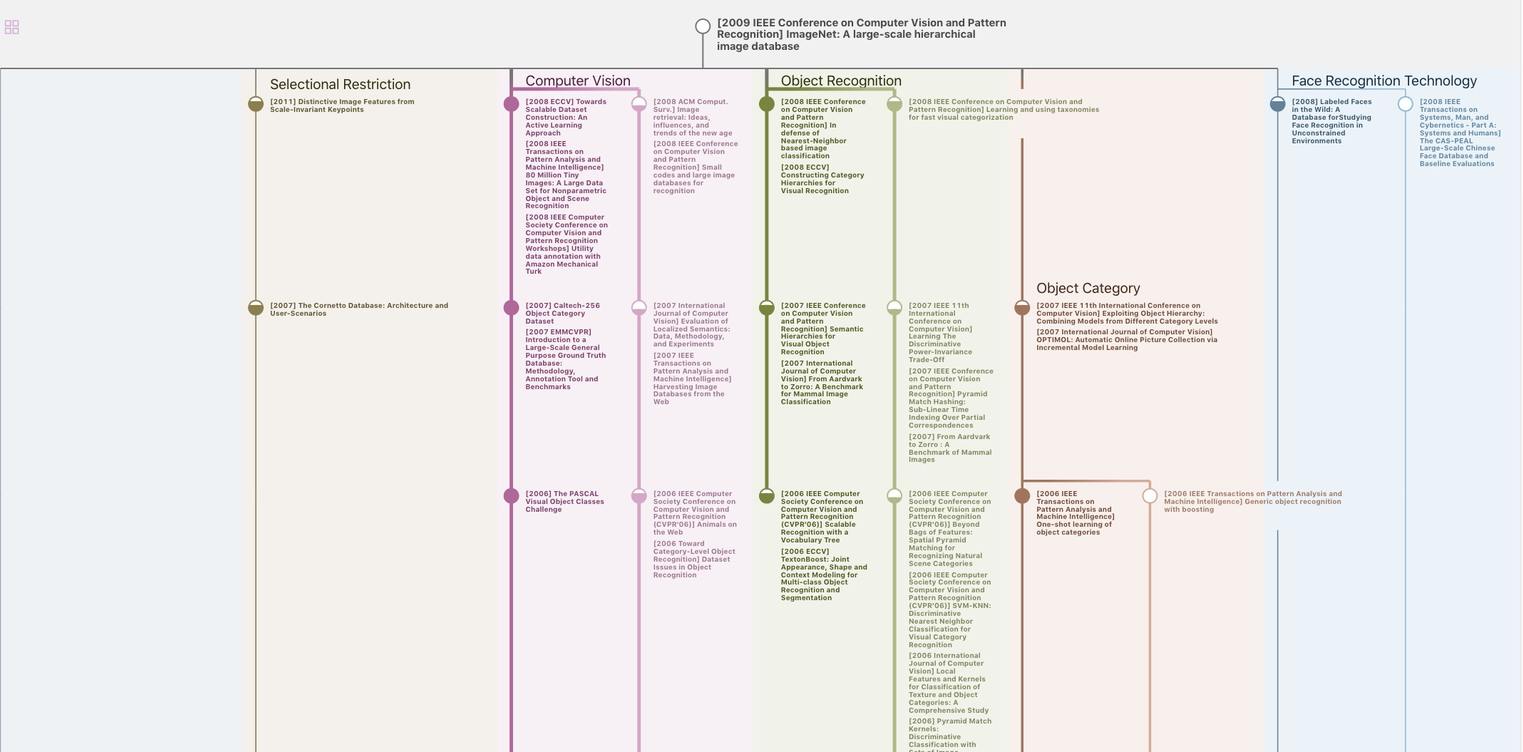
生成溯源树,研究论文发展脉络
Chat Paper
正在生成论文摘要