Uplift Modeling: State-of-the-art and novel approaches
semanticscholar(2019)
摘要
Knowing whether a certain action causes a certain reaction is significant in business analytics. Uplift modeling is at the heart of prescriptive analytics and aims at estimating the net difference in an outcome resulting from a specific action or treatment that is applied. Prescriptive analytics extends on predictive analytics by allowing to estimate an outcome in function of control variables. We introduce the core concepts of uplift modeling, compare it to predictive modeling and present multiple extensions towards the literature. First, a survey on uplift modeling is provided by identifying and comparing various groups of approaches and reviewing evaluation metrics for assessing the performance of uplift models. To assess the strength of the state-of-the-art, an experimental evaluation is done on four real-world data sets which provide further insight into their use. Uplift random forests are found to be consistently among the best performing techniques in terms of the Qini and Gini measures. Depending on the data, uplift models are frequently observed to be unstable and display a strong variability in terms of performance across different folds in the cross-validation experimental setup. We find that the available evaluation metrics do not provide an intuitively understandable indication of the actual use and performance of the model. Specifically, existing evaluation metrics do not facilitate a comparison of uplift models and predictive models and evaluate performance either at an arbitrary cutoff or over the full spectrum of potential cutoffs. Therefore, we introduce a novel evaluation metric which is called the Maximum Profit Uplift (MPU) measure which allows to assess the performance in terms of the maximum potential profit that can be achieved by adopting an uplift model. The measure is developed for evaluating customer churn uplift models and extends upon the maximum profit measure for evaluating customer churn prediction models. As part of the introduction of the MPU measure, we introduce the generally applicable liftup curve and liftup measure for evaluating uplift models, as counterparts of the lift curve and lift measure that are broadly used for evaluating predictive models. These measures are subsequently applied to assess and compare the performance of customer churn prediction and uplift models in a case study that applies uplift modeling for customer retention in the financial industry. We find that uplift models outperform predictive models and allow to boost the profitability of retention campaigns. Lastly, as the output of uplift models is used to produce rankings, we investigate the potential to learn these rankings directly by looking into learning-to-rank techniques in the context of uplift modeling. We explore how uplift evaluation measures can be integrated into the learning-to-rank framework and introduce a new learning-to-rank metric called the promoted cumulative gain (PCG), which optimizes the area under the uplift curve. We utilize the learning-to-rank technique LambdaMART to optimize the ranking according to the PCG and show improved results comparing to standard learning-to-rank measures on all datasets and improved results over state-of-the-art uplift modeling techniques on some datasets. We further show how learning-to-rank models can be optimized for top fractions of the data, although these results do not generalize well. This dissertation shows the value of uplift modeling in today's world of business analytics. We conclude the dissertation by presenting several open research topics for future work. We believe there is a lot of value in profit-driven uplift modeling techniques. Additionally, we would like to see more research on a practical case-study spanning multiple campaigns and the issues that arise with it. Finally, uplift modeling is part of a larger field called causal inference, however few links between the two fields have been made in the research today. Connecting the two research fields could be beneficial for both fields.
更多查看译文
AI 理解论文
溯源树
样例
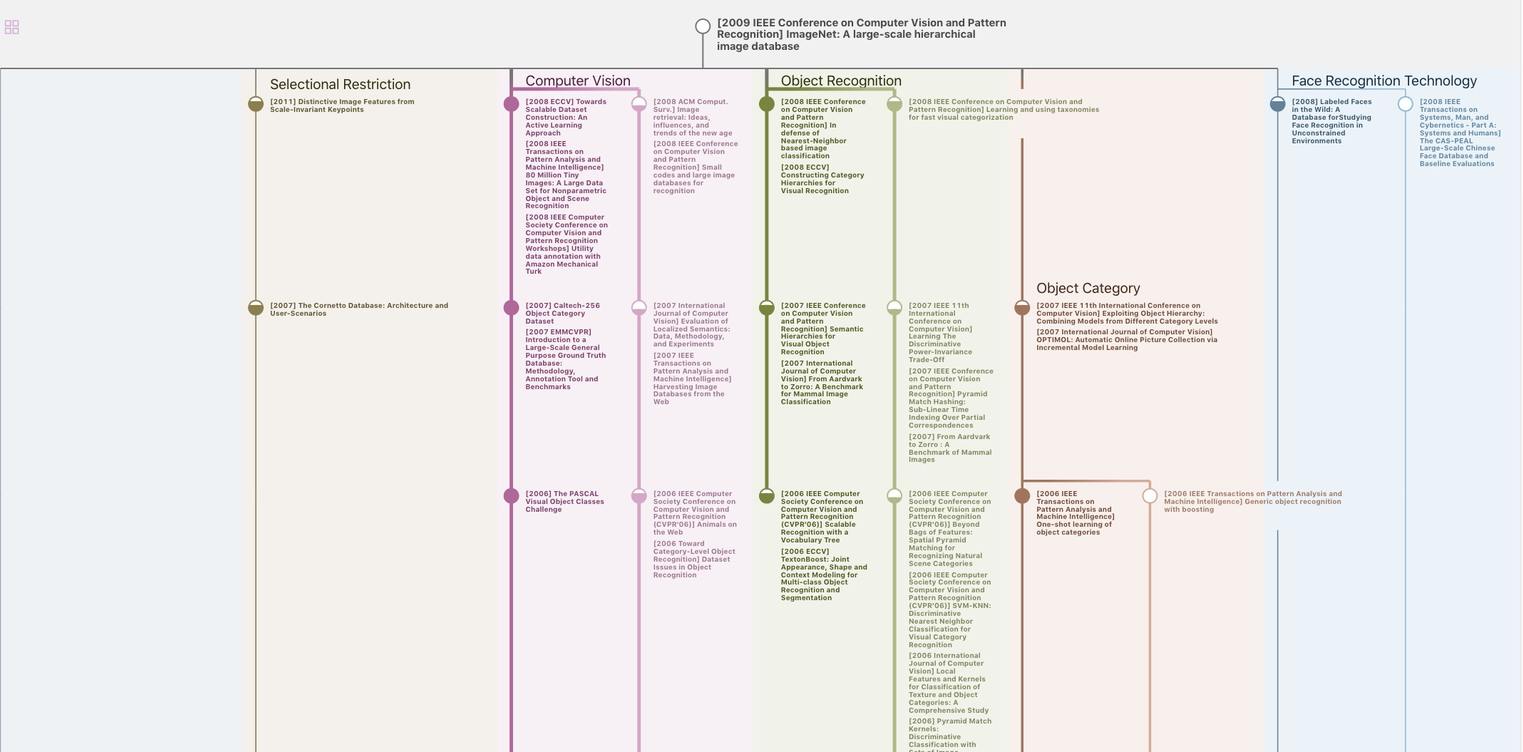
生成溯源树,研究论文发展脉络
Chat Paper
正在生成论文摘要