Improved Activation Detection via Rice-Distributed fMRI Time Series Modeling
semanticscholar(2017)
摘要
Two developments in fMRI magnitude time series modeling, namely, the incorporation of temporal dependence and the Ricean distribution, have been separated by a distributional “mismatch”: such time series modeling is largely based upon Gaussian-distributional-based extensions to the general linear model, which precludes its use under Ricean modeling. We bridge this gap by applying AR(p) errors to the latent, Gaussian-distributed real and imaginary components from which the Riceandistributed magnitudes are computed by augmenting the observed magnitude data by missing phase data in an EM algorithm framework. We use the EM algorithm for parameter estimation and extend it to compute approximate standard errors and test statistics for activation and AR order detection. When compared to the standard Gaussian AR(p) model, this “AR(p) Ricean model” produces lessbiased parameter estimates and similar performance on a real fMRI dataset.
更多查看译文
AI 理解论文
溯源树
样例
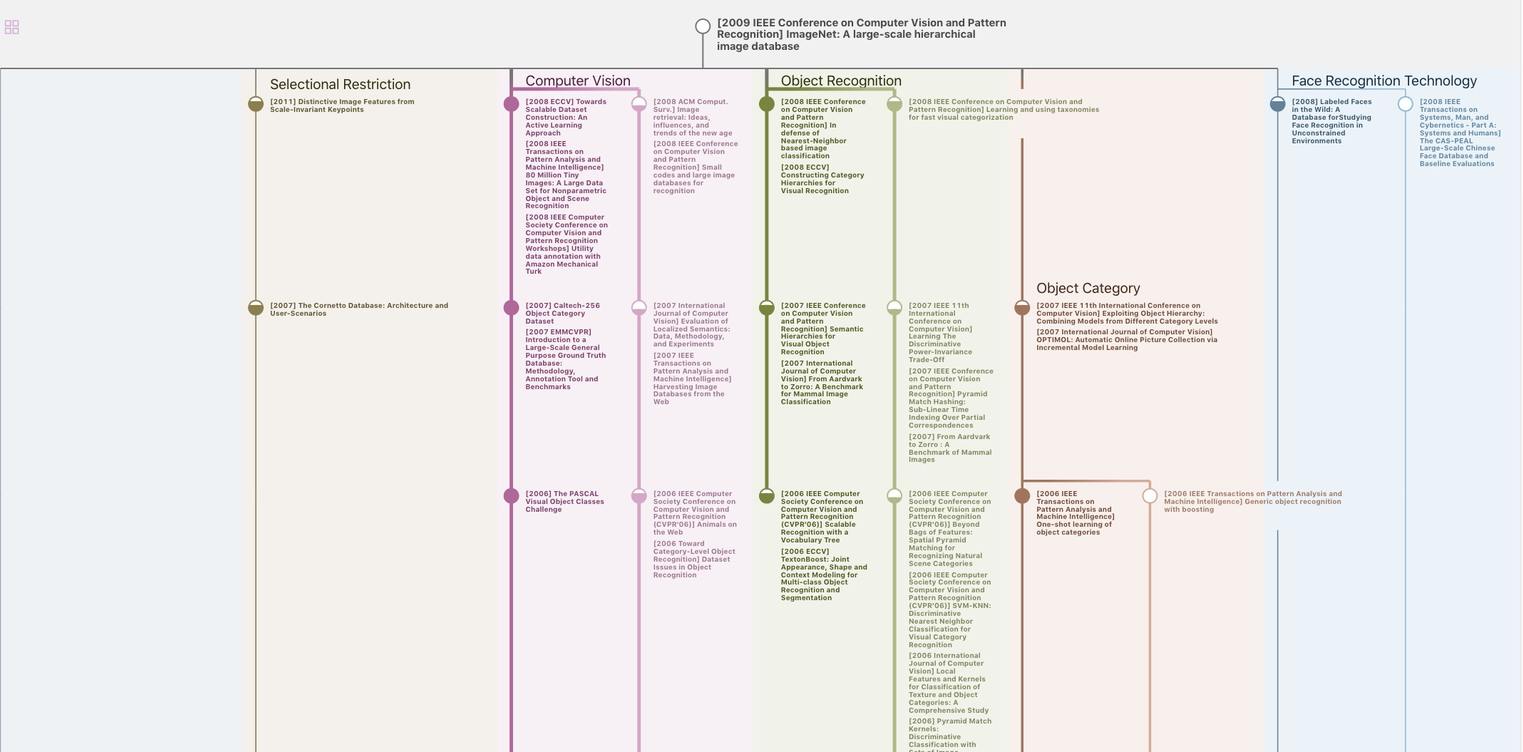
生成溯源树,研究论文发展脉络
Chat Paper
正在生成论文摘要