Policy Continuation and Policy Evolution with Hindsight Inverse Dynamics
semanticscholar(2019)
摘要
Solving goal-oriented tasks is an important but challenging problem in reinforcement learning (RL). For such tasks, the rewards are often sparse, making it difficult to learn a policy effectively. To tackle this difficulty, we propose a new approach called Policy Continuation and and Policy Evolution with Hindsight Inverse Dynamics (PC&PEHID). This approach learns from Hindsight Inverse Dynamics based on Hindsight Experience Replay. This work also extends it to multi-step settings with Policy Continuation and Policy Evolution. The proposed method is general – it can work in isolation or be combined with other on-policy and off-policy algorithms. On challenging multi-goal tasks, PC&PEHID significantly improves the sample efficiency as well as the final performance.
更多查看译文
AI 理解论文
溯源树
样例
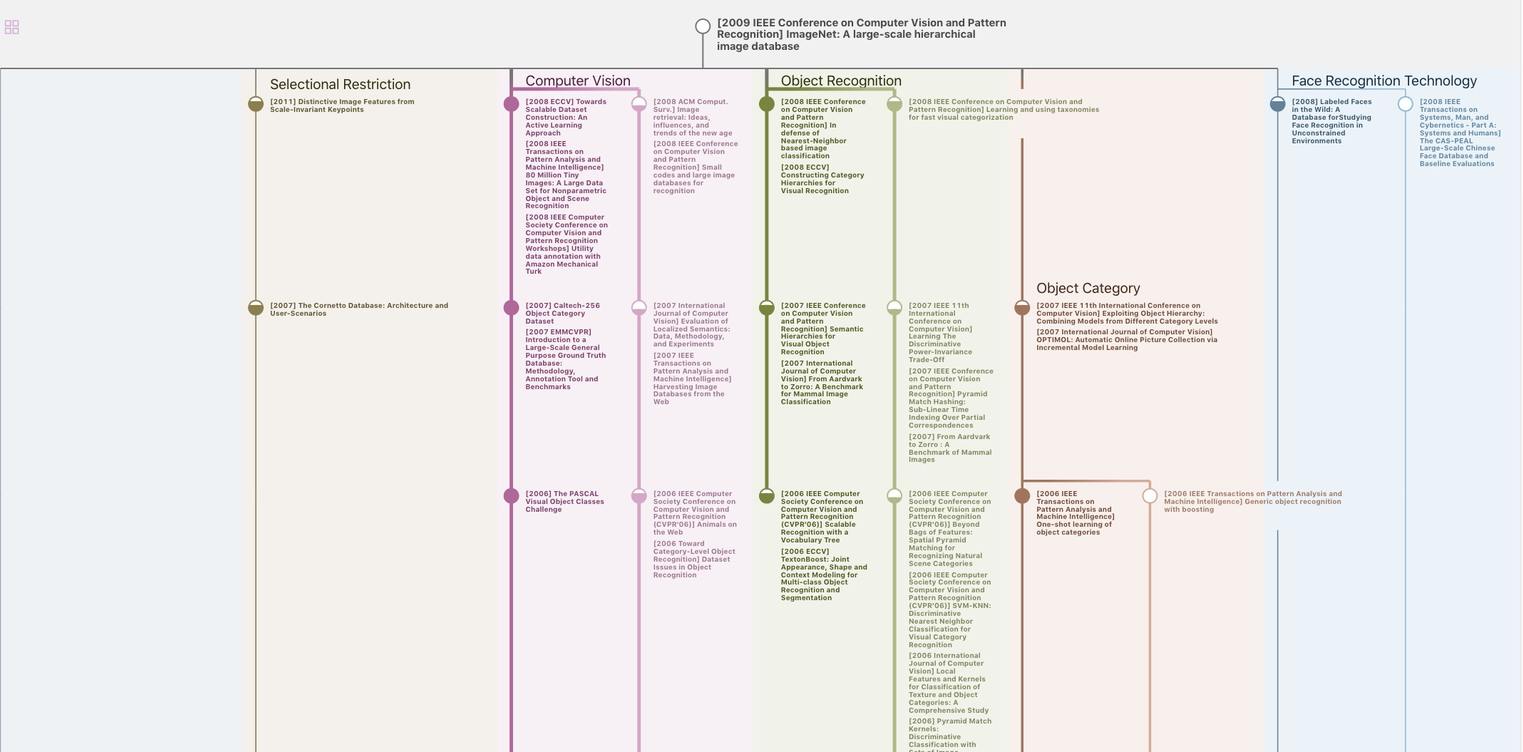
生成溯源树,研究论文发展脉络
Chat Paper
正在生成论文摘要