Accelerating Multi-agent Reinforcement Learning with Dynamic Co-learning
semanticscholar(2015)
摘要
We introduce an approach to adaptively identify opportunities to periodically transfer experiences between agents in large-scale, stochastic, homogeneous, multi-agent systems. This algorithm operates in an on-line, distributed manner, using supervisor-directed transfer, leading to more rapid acquisition of appropriate policies in systems with a large number of cooperating reinforcement learning agents. Our method constructs high-level characterizations of the system—called contexts—and uses them to identify which agents operate under approximately similar dynamics. A set of supervisory agents compute and reason over contextual similarity between agents, identifying candidates for experience sharing, or co-learning. Using a tiered architecture, state, action, and reward tuples are propagated amongst the members of co-learning groups. We demonstrate the effectiveness of this approach on a large-scale distributed task allocation problem with hundreds of co-learning agents operating in an unknown environment with non-stationary neighbors.
更多查看译文
AI 理解论文
溯源树
样例
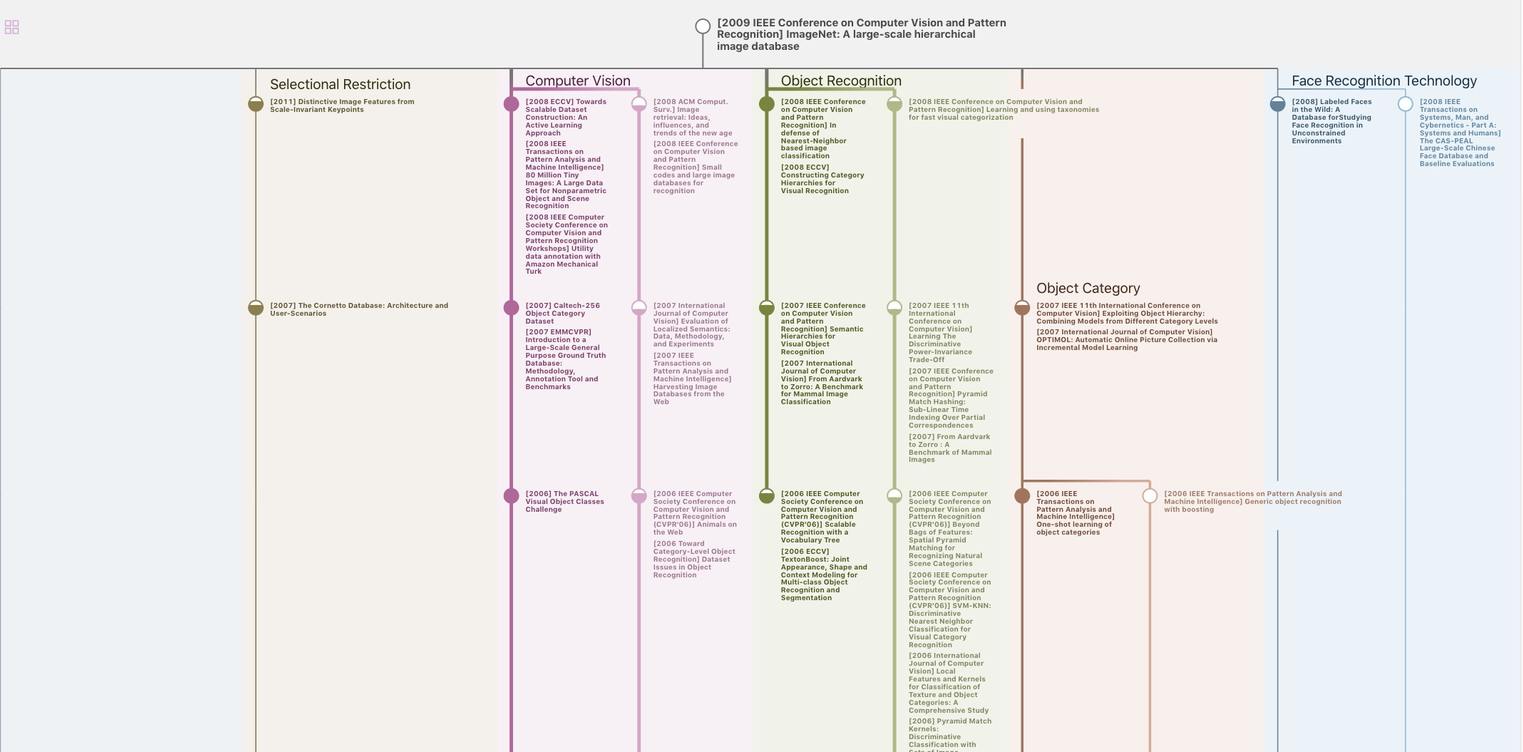
生成溯源树,研究论文发展脉络
Chat Paper
正在生成论文摘要