Anomaly Detection based on Recursive Least-Square Filter for Robust Intelligent Transportation Systems
semanticscholar(2015)
摘要
For unmanned autonomous vehicles, obstacle detection is one of the most important aspects to support robust collision avoidance systems. In order to prevent collision, autonomous vehicles are equipped with heterogeneous sensors to monitor surrounding environments. While these sensors assist such needs, recent studies have demonstrated that malicious attackers can manipulate them to yield false values and trigger harms. In addition, unintended sensor failures can also cause critical situations where vehicles cannot reliably avoid the collisions. In this paper, we propose a robust collision avoidance system using an Anomaly detection mechanism. Our approach is based on Recursive Least Squares (RLS) filter, which determines a fault sensor value based on Anomaly detection. We deploy our system in an indoor test-bed and demonstrate improved safety in an intelligent transportation environment.
更多查看译文
AI 理解论文
溯源树
样例
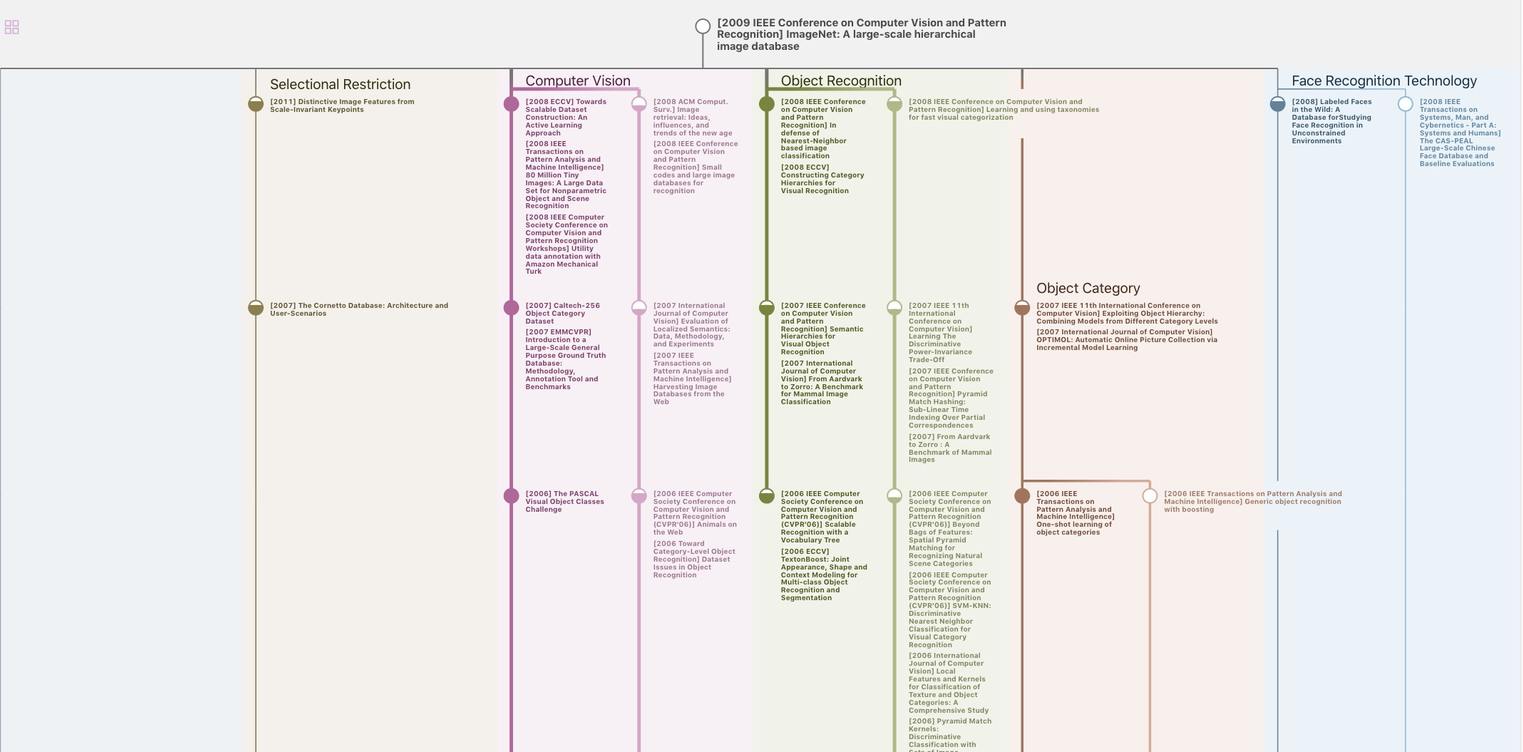
生成溯源树,研究论文发展脉络
Chat Paper
正在生成论文摘要