A Cold Start Recommendation System Using Item Correlation and User Similarity
semanticscholar(2011)
摘要
Conventional recommendation systems tend to focus on variations of well-known information retrieval techniques. We took a fresh approach, rather than to follow the traditional, commonly applied recommendation methodology of creating a user-item matrix, and then using them to make recommendations. Instead, we established and examined three types of relationships: user-user similarity, wine-wine similarity and user preference relationships, in the form of adjacency lists. Using this approach, we did not encounter the usual problems associated with large dimension matrices, such as sparsity [5] and synonymy, as well as the basic problems of storing the large matrix, and having to perform a large number of computations every single time. We attempted to address the synonymy problem by using the wine-wine similarity index we formed. Also, we developed a model that took care of the cold start [6] problem which is fairly common in recommendation systems. We attempted to address the grey sheep problem as well, by minimizing the effect of any one outlying element, and taking the overall cumulative effect of all the elements. With our recommendation system, we wanted to establish a relationship of trust with the user, because just a few erroneous values would greatly discredit our models. In this paper, we defined 25 attributes by which a wine could be classified, and determined the wine-wine and useruser similarities, as well as the user preferences for the wines he has tried so far using our own novel scoring mechanism. The wines as such have had no scores associated with them with regards to the 25 attributes, rather, the scores were meaningful only when the relationships between the two wines were considered. We defined our own two methodologies, cold start and top k1, k2 models to address the issues associated with recommendation systems. Our experimental results showed that the newly introduced cold start model performed better than the traditional models of pure content-based [3], pure collaborative-based and content + colloborative based [10, 1] approaches with regards to Permission to make digital or hard copies of all or part of this work for personal or classroom use is granted without fee provided that copies are not made or distributed for profit or commercial advantage and that copies bear this notice and the full citation on the first page. To copy otherwise, to republish, to post on servers or to redistribute to lists, requires prior specific permission and/or a fee. CSE 635 ’11 Buffalo, NY USA Copyright 20XX ACM X-XXXXX-XX-X/XX/XX ...$10.00. the quality. Also, our top k1, k2 model performed almost as well as the content + colloborative based model. Our resulting wine recommendations were consistent with the expectations of the user’s particular wine tastes.
更多查看译文
AI 理解论文
溯源树
样例
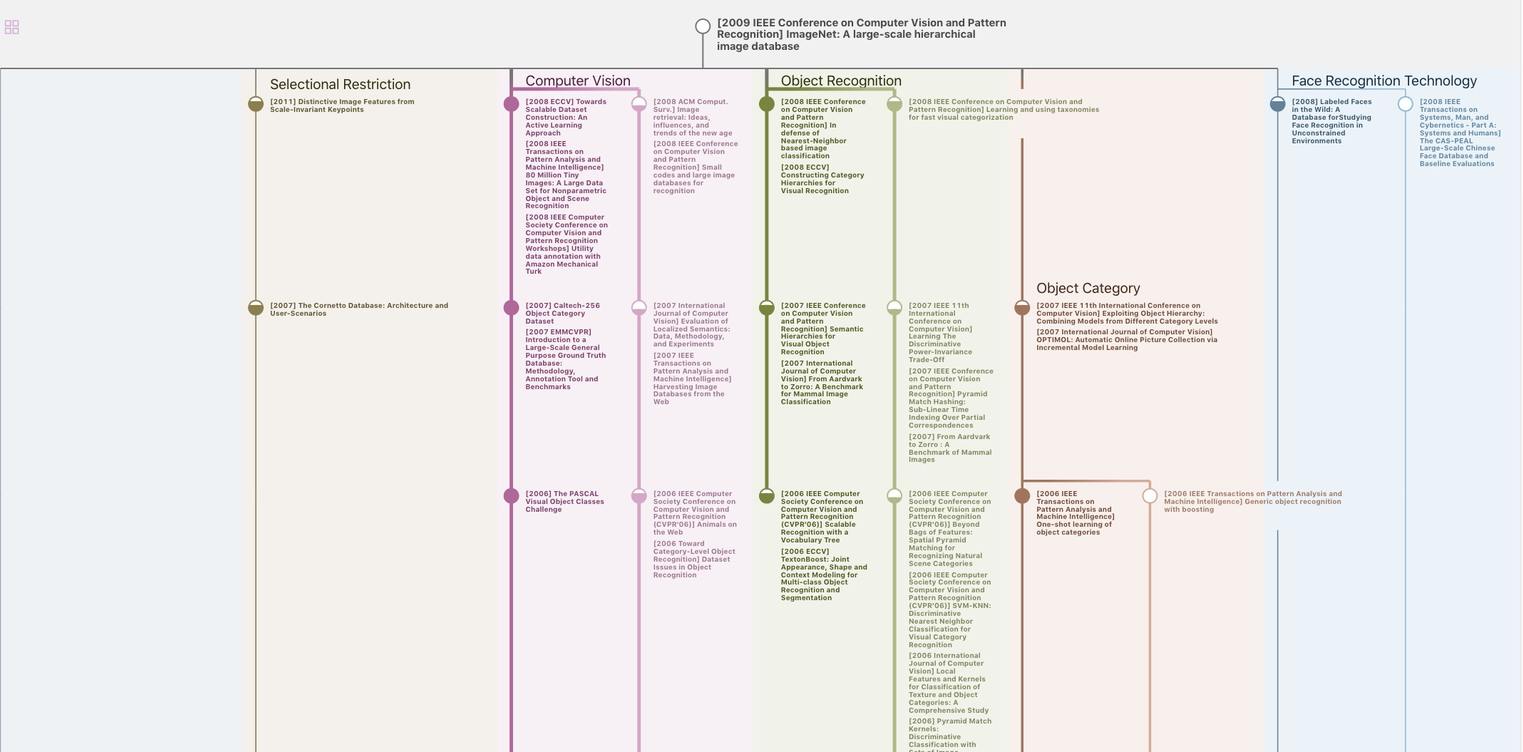
生成溯源树,研究论文发展脉络
Chat Paper
正在生成论文摘要