A Close Examination of Factual Correctness Evaluation in Abstractive Summarization
semanticscholar(2020)
摘要
Generating fabricated facts has been a long-standing problem of abstractive summarization models, and has significantly limited their applicability in practice. Previous works about improving factual correctness only rely on human evaluations, which weakens the transparency and reproducibility. In this work, we aim to examine how to evaluate factual correctness. We start with a human study to thoroughly understand what affects factual correctness evaluations, and we further assess whether current automatic factual evaluation metrics are able to capture factual errors. Our experiments demonstrate that the attributes of models and datasets can drastically affect the evaluation of factual correctness, and how to design an accurate, modeland data-agnostic evaluation metrics still remains a challenge to the NLP community.
更多查看译文
AI 理解论文
溯源树
样例
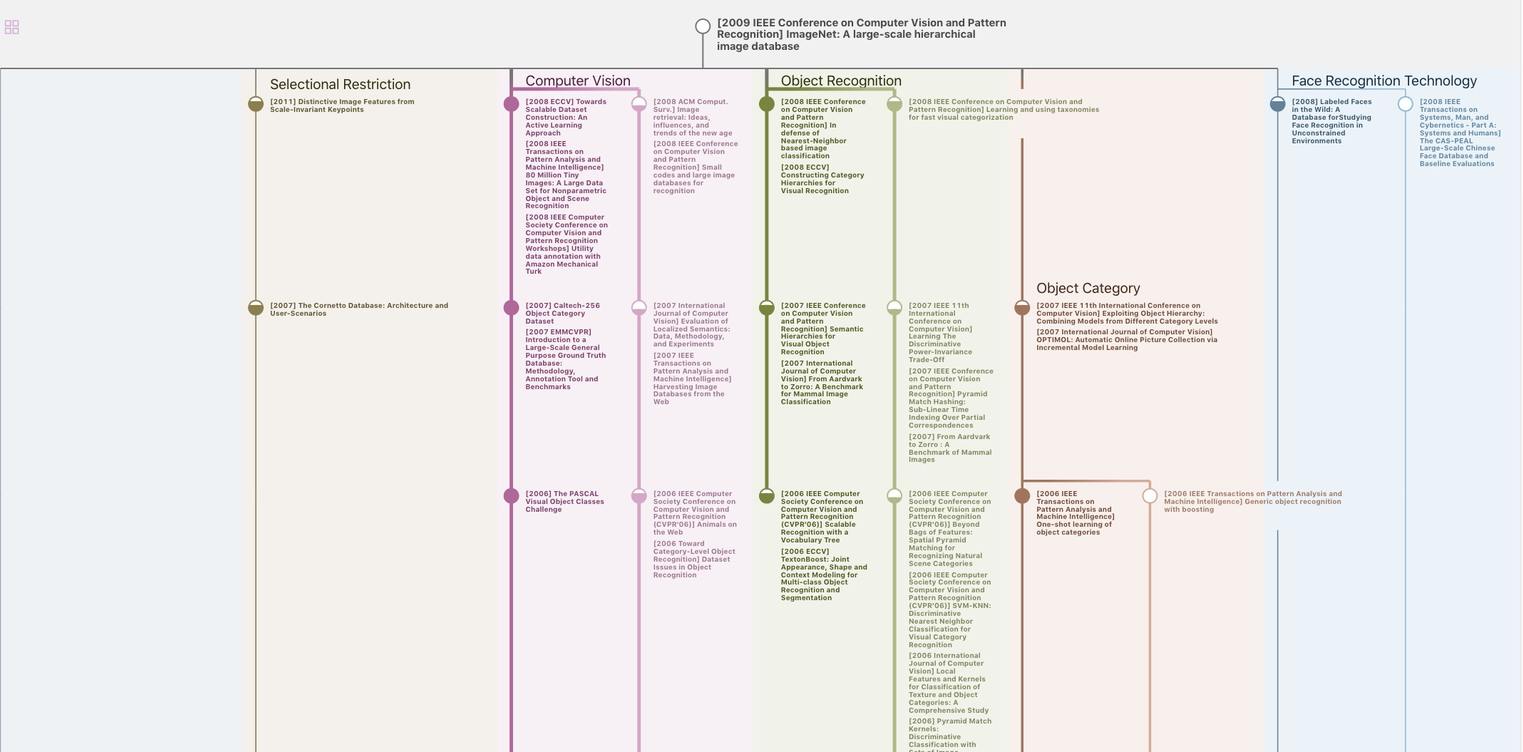
生成溯源树,研究论文发展脉络
Chat Paper
正在生成论文摘要