Analysis of unevenly spaced time series data in highly distributed and unreliable networks
semanticscholar(2016)
摘要
Missing values may appear in collected time series for a number of reasons such as: network failure, power failures and other unexpected conditions during data transfer or their collection what creates a challenge when trying to analyze such data. Accordingly, there is need for reconstructing these missing values and gaps, i.e. to do transformation from unevenly spaced into evenly spaced time series, but based on the best fitted forecast method. Several types of time series are generally distinguished, including stationarity time series (e.g. white noise) and different patterns in non-stationary time series such as: trend, seasonal and a combination of them. By selecting a forecasting method that has the best accuracy (the smallest error) for adequate and recognized type of time series, an automated recursive predicting function is presented. An important factor in determining the best forecasting method involves the different forecast accuracy measures.
更多查看译文
AI 理解论文
溯源树
样例
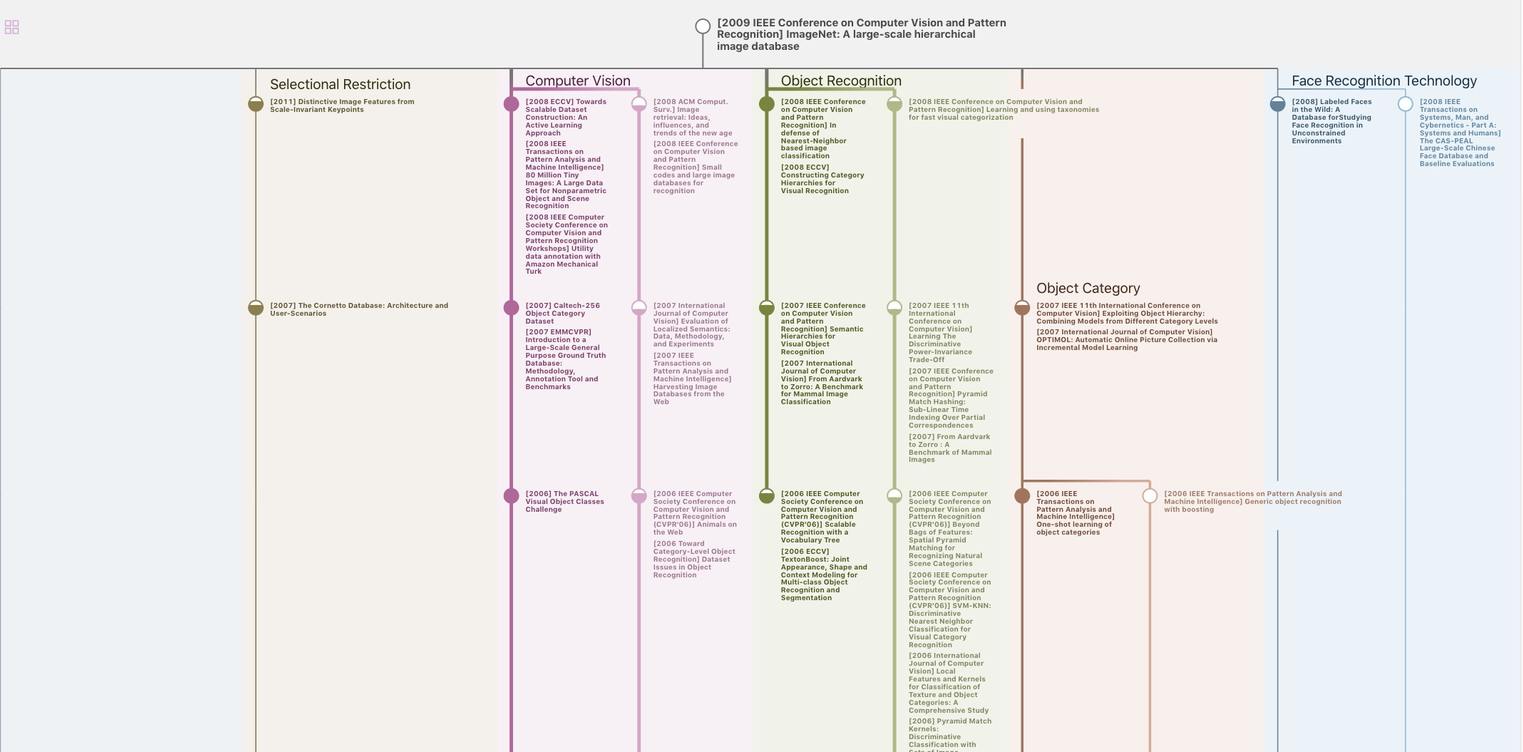
生成溯源树,研究论文发展脉络
Chat Paper
正在生成论文摘要