Using zolotarev ’ s rational approximation for computing the polar , symmetric eigenvalue , and singular value decompositions
semanticscholar(2015)
摘要
The polar decomposition A = UpH finds many uses in applications, and it is a fundamental tool for computing the symmetric eigenvalue decomposition and the singular value decomposition via a spectral divide-and-conquer process. Conventional algorithms for these decompositions are suboptimal in view of recent trends in computer architectures, which require minimizing communication together with arithmetic costs. Spectral divide-and-conquer algorithms, which recursively decouple the problem into two smaller subproblems, can achieve both requirements. For computing the polar decomposition, the scaled Newton and QDWH iterations are two of the most popular algorithms, as they are backward stable and converge in at most nine and six iterations, respectively. Following this framework, in this paper, we develop a higher-order variant of the QDWH iteration for the polar decomposition. The key idea of this algorithm comes from approximation theory: we use the best rational approximant for the scalar sign function due to Zolotarev in 1877. The algorithm exploits the extraordinary property enjoyed by the sign function that a high-degree Zolotarev function (best rational approximant) can be obtained by appropriately composing low-degree Zolotarev functions. This lets the algorithm converge in just two iterations in double-precision arithmetic, with the whopping rate of convergence seventeen. The resulting algorithms for the symmetric eigenvalue and singular value decompositions have higher arithmetic costs than the QDWH-based algorithms, but are better-suited for parallel computing and exhibit excellent numerical backward stability.
更多查看译文
AI 理解论文
溯源树
样例
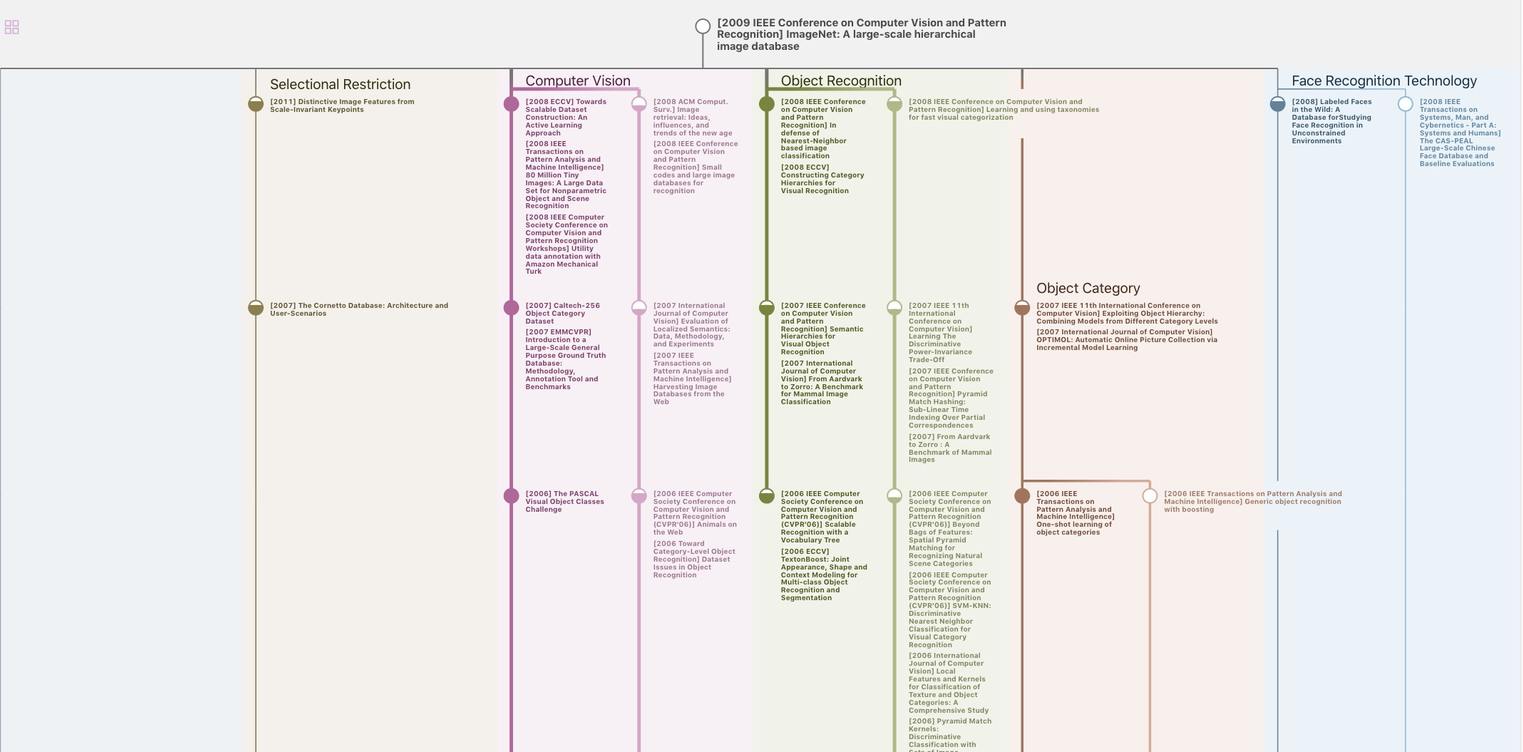
生成溯源树,研究论文发展脉络
Chat Paper
正在生成论文摘要