Natural Option
semanticscholar(2018)
摘要
The recently proposed option-critic architecture (Bacon, Harb, and Precup 2017) provides a stochastic policy gradient approach to hierarchical reinforcement learning. Specifically, it provides a way to estimate the gradient of the expected discounted return with respect to parameters that define a finite number of temporally extended actions, called options. In this paper we show how the option-critic architecture can be extended to estimate the natural gradient (Amari 1998) of the expected discounted return. To this end, the central questions that we consider in this paper are: 1) what is the definition of the natural gradient in this context, 2) what is the Fisher information matrix associated with an option’s parameterized policy, 3) what is the Fisher information matrix associated with an option’s parameterized termination function, and 4) how can a compatible function approximation approach be leveraged to obtain natural gradient estimates for both the parameterized policy and parameterized termination functions of an option with per-time-step time and space complexity linear in the total number of parameters. Based on answers to these questions we introduce the natural option critic algorithm. Experimental results showcase improvement over the vanilla gradient approach.
更多查看译文
AI 理解论文
溯源树
样例
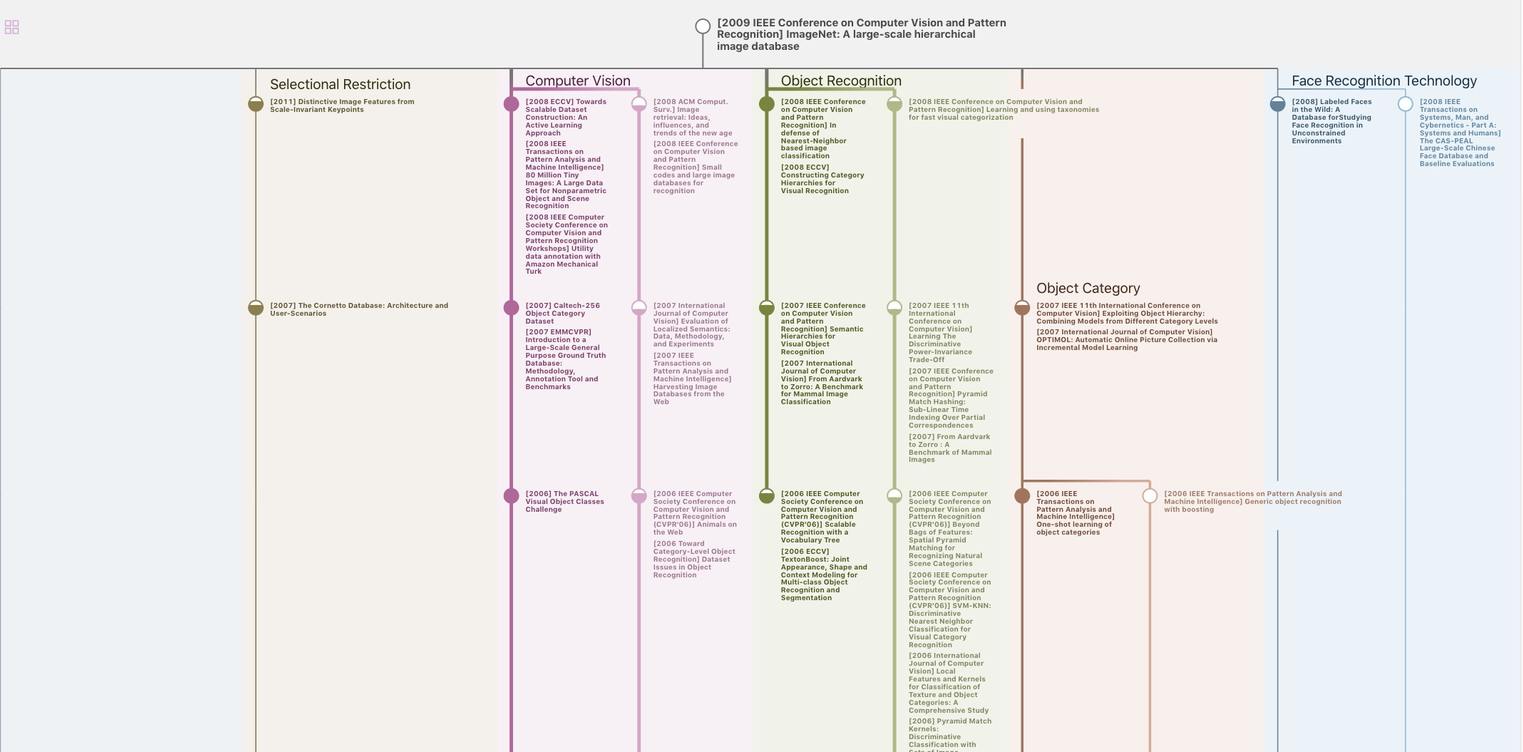
生成溯源树,研究论文发展脉络
Chat Paper
正在生成论文摘要