Modelling imbalanced classes
semanticscholar(2020)
摘要
In this study separate sampling was applied to various modelling procedures to assist in the identification of the most important variables describing smartphone users who are security compliant. Initial analysis of the data found that only 7% of smartphone users reported applying security measures to protect their phones and/or their personal information stored on their devices. Due to the class imbalance in the target variable, predictive modelling procedures failed to produce accurate models. Separate sampling proportions were introduced to establish if classification accuracy could be improved. This study tested target class over-sampling ratios of 20%, 30%, 40% and 50% and compared the results of the models fitted on these data sets to those fitted on the original data where no separate sampling was applied. Models fitted included: decision trees, 5-fold cross-validated decision trees, logistic regression, neural networks and gradient boosted decision trees. The results showed that the logistic regression and neural network models produced unstable models regardless of the target class ratios. More stable models were however reported for the decision trees, 5fold cross-validated decision trees and gradient boosted decision trees. Variables found to influence mobile security compliance included age, gender and various security/privacy related behaviors.
更多查看译文
AI 理解论文
溯源树
样例
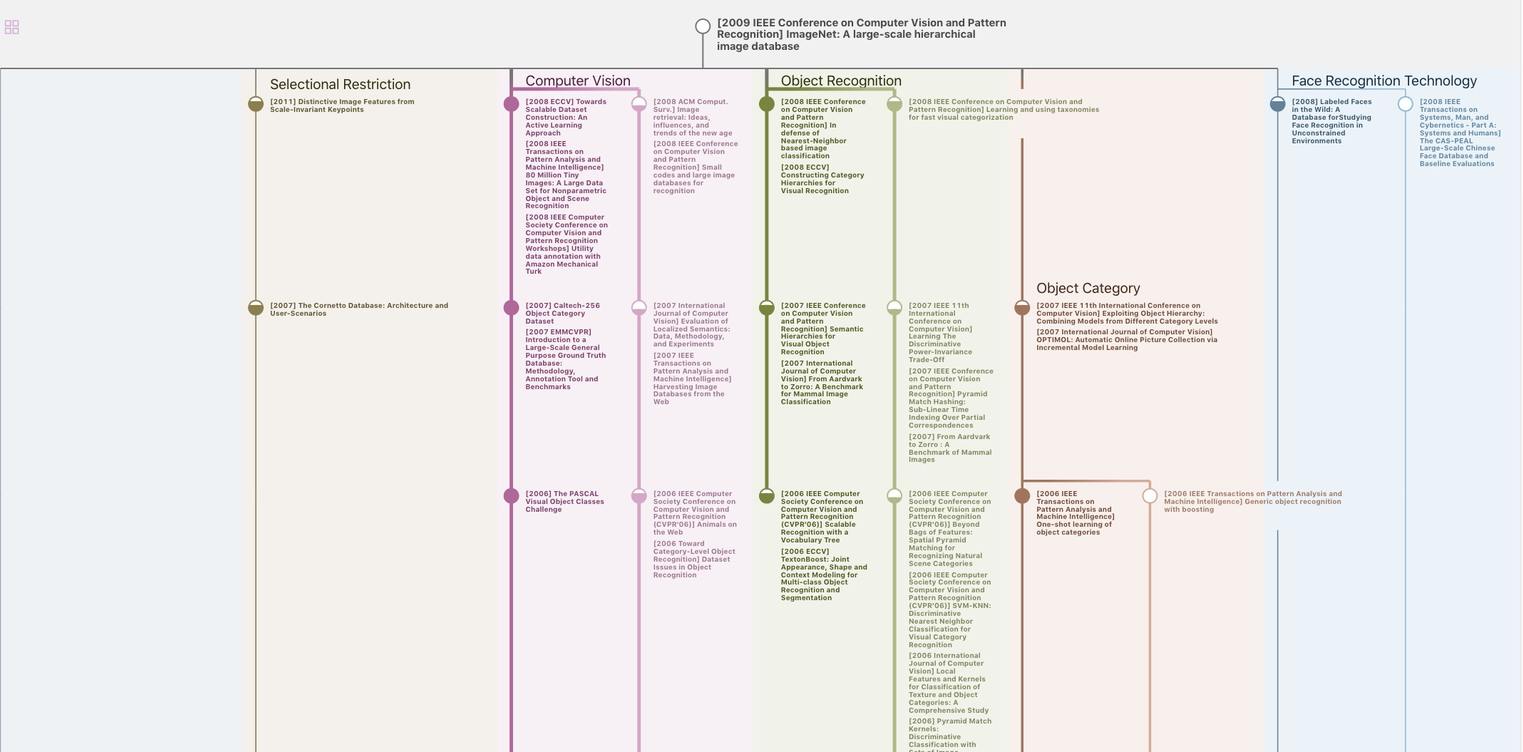
生成溯源树,研究论文发展脉络
Chat Paper
正在生成论文摘要