White Matter Hyperintensity Segmentation Via Ensembled Semi-supervised Large Margin Learning
semanticscholar(2017)
摘要
Precise detection and quantification of white matter hyperintensities (WMHs) is of great interest in studies of neurological and vascular disorders. It is believed that the accurate quantification of WMHs in terms of total volume and distribution is of clinical importance for disease diagnosis, prognosis, and tracking of disease progressions. In this work, we propose a novel ensembled semi-supervised machine learning algorithm for the segmentation of WMHs. The proposed algorithm optimizes a kernel based max-margin objective function which aims to maximize the margin averaged over inliers and outliers while exploiting a limited amount of available labelled data. We show that the learning problem can be formulated as a joint framework, learning a classifier and label assignment simultaneously, which can be solved efficiently by an iterative algorithm. The learned ensemble model can give a probability estimate of whether a voxel is WMH or not, which is then further refined in a post-processing step by spatial priors and fully connected conditional random field (CRF). We evaluate our method on a database of 167 brain fluid-attenuated inversion recovery (FLAIR) magnetic resonance (MR) images from subjects with vascular disease. Compared with other well established methods, the WMHs identified by the proposed algorithm were found to have better agreement with WMHs determined by semi-automated computational processing with expert visual correction. Both qualitative and quantitative evaluation of the proposed algorithm show that it outperforms other well known methods for WMH segmentation.
更多查看译文
AI 理解论文
溯源树
样例
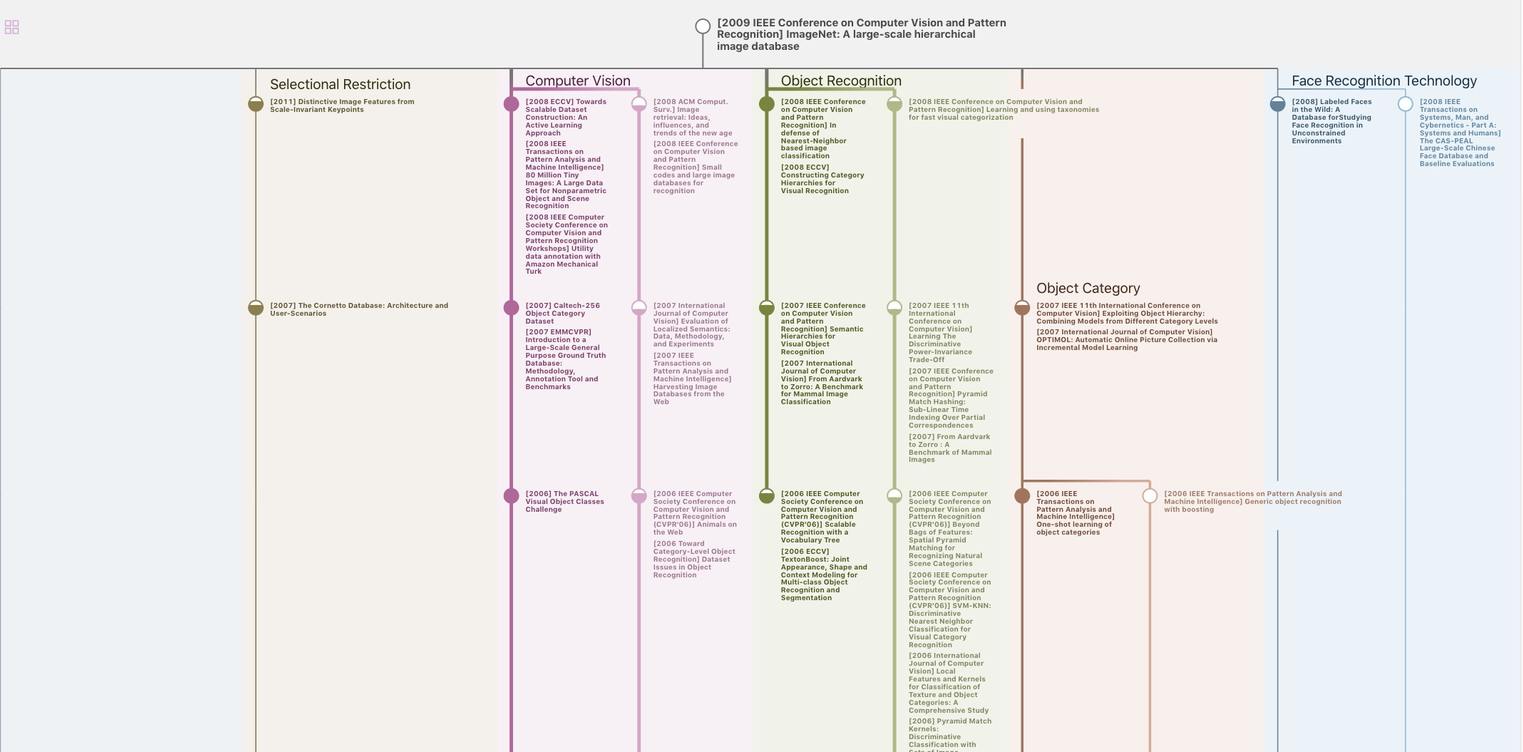
生成溯源树,研究论文发展脉络
Chat Paper
正在生成论文摘要