Structure Analysis of High Dimensional Tensor Data
semanticscholar(2013)
摘要
Multi-way tensor data have become prevalent in many scientific areas such as genomics and biomedical imaging. We consider a K-way tensor-normal distribution, where the precision matrix for each way has a graphical interpretation. We develop an l1 penalized maximum likelihood estimation and an efficient coordinate descent-based algorithm for model selection and estimation in such tensor normal graphical models (TNGMs). When the dimensions of the tensor are fixed, we provide theoretical results on the asymptotic distributions and oracle property for the proposed estimates of the precision matrices. When the dimensions diverge as the sample size goes to infinity, we present the rates of convergence of the estimates and the sparsistency results. Simulation results demonstrate that the TNGMs can lead to better estimates of the precision matrices and better identification of the graph structures defined by the precision matrices than the standard Gaussian graphical models. We illustrate the methods with an analysis of yeast gene expression data measured over different time points and under different experimental conditions.
更多查看译文
AI 理解论文
溯源树
样例
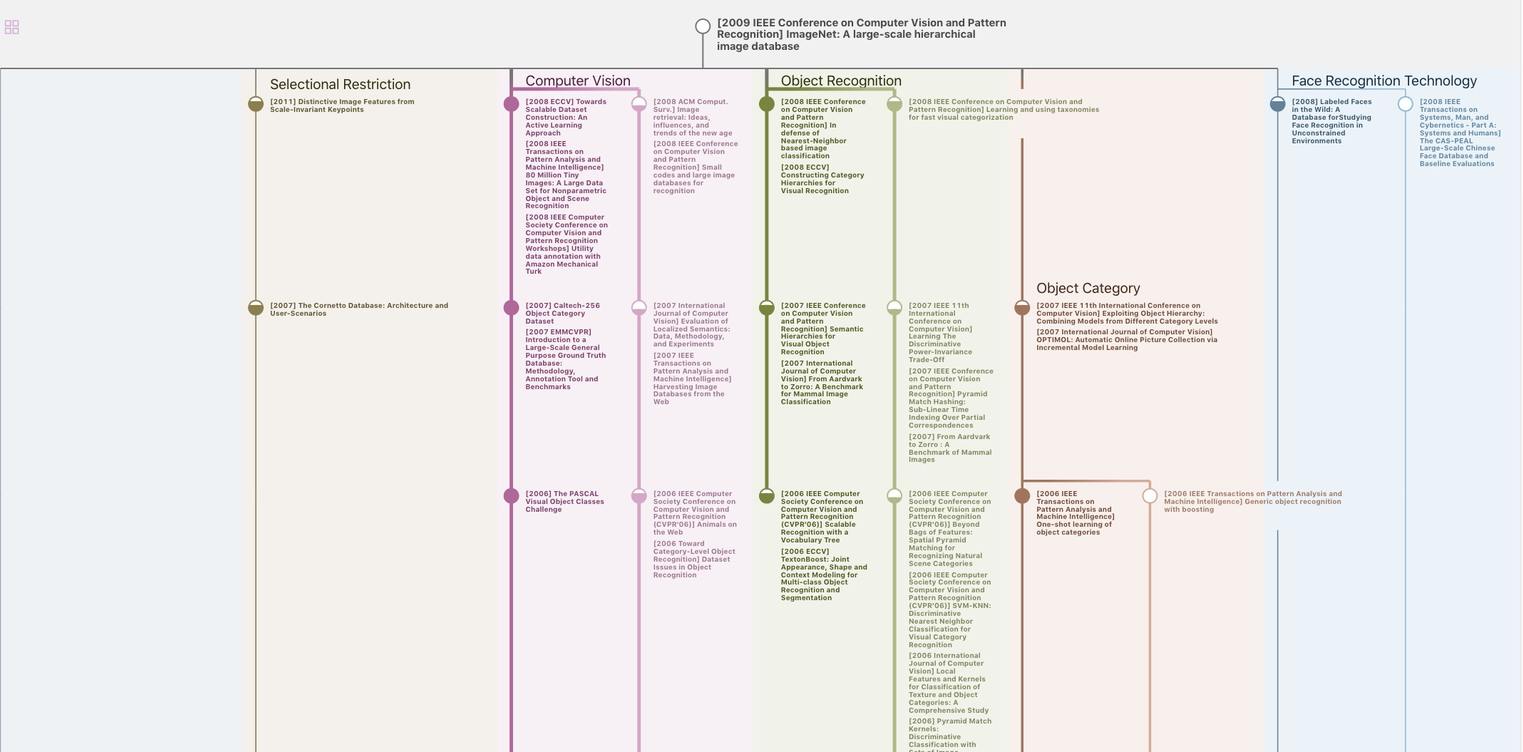
生成溯源树,研究论文发展脉络
Chat Paper
正在生成论文摘要