InferLine : Prediction Pipeline Provisioning and Management for Tight Latency Objectives
semanticscholar(2019)
摘要
Serving prediction pipelines spanning multiple models and hardware accelerators is a key challenge in production machine learning. Optimally configuring these pipelines to meet tight end-to-end latency goals is complicated by the interaction between model batch size, the choice of hardware accelerator, and variation in the query arrival process. In this paper we introduce InferLine, a system which provisions and executes ML prediction pipelines subject to end-to-end latency constraints by proactively optimizing and reactively controlling per-model configurations in a fine-grained fashion. InferLine leverages automated offline profiling and performance characterization to construct a cost-minimizing initial configuration and then introduces a reactive planner to adjust the configuration in response to changes in the query arrival process. We demonstrate that InferLine outperforms existing approaches by up to 7.6x in cost while achieving up to 34.5x lower latency SLO miss rate on realistic workloads and generalizes across state-of-the-art model serving frameworks.
更多查看译文
关键词
Hardware acceleration,Latency (engineering),Network calculus,Discrete event simulation,Combinatorial search,Provisioning,Distributed computing,Profiling (computer programming),Pipeline transport,Computer science
AI 理解论文
溯源树
样例
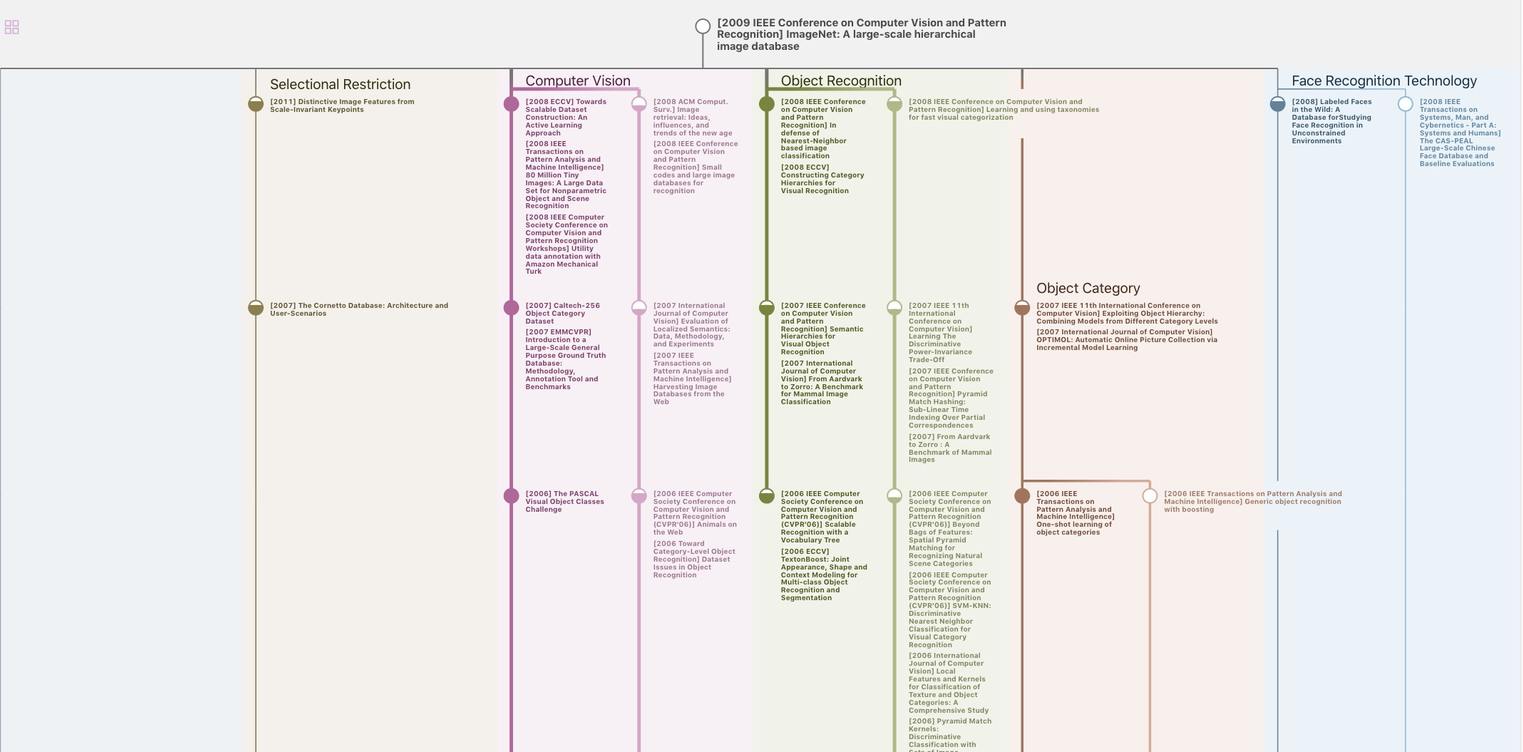
生成溯源树,研究论文发展脉络
Chat Paper
正在生成论文摘要