Fault prediction using the combination of regularized OS-ELM and strong tracking SCKF
semanticscholar(2015)
Abstract
As one of the most important aspects in maintenance, fault prediction has attracted an increasing attention for avoiding system catastrophic damage and ensuring reliability. Considering prognosis of unmeasured fault parameter in nonlinear system, a novel forecasting algorithm is presented based on the combination of ROS-ELM (regularized online sequential extreme learning machine) time series predictor and STSCKF (strong tracking square-root cubature Kalman filter). ROS-ELM is utilized to forecast system measurements in future time instant, which are used by STSCKF as measurement variables during filter process. The fading factor is introduced into the square root of the STSCKF prediction error covariance to tune the gain matrix. A state and parameter joint filter based on STSCKF is proposed to solve the problem that faulty changing function is unknown in practice. An experiment case is provided to verify the good performance of the presented approach. Key-Words: Strong tracking filter; Square-root cubature Kalman filter (SCKF); State and parameter joint filter; Online sequential extreme learning machine (OS-ELM) ; Three-tank system; Failure prognosis
MoreTranslated text
AI Read Science
Must-Reading Tree
Example
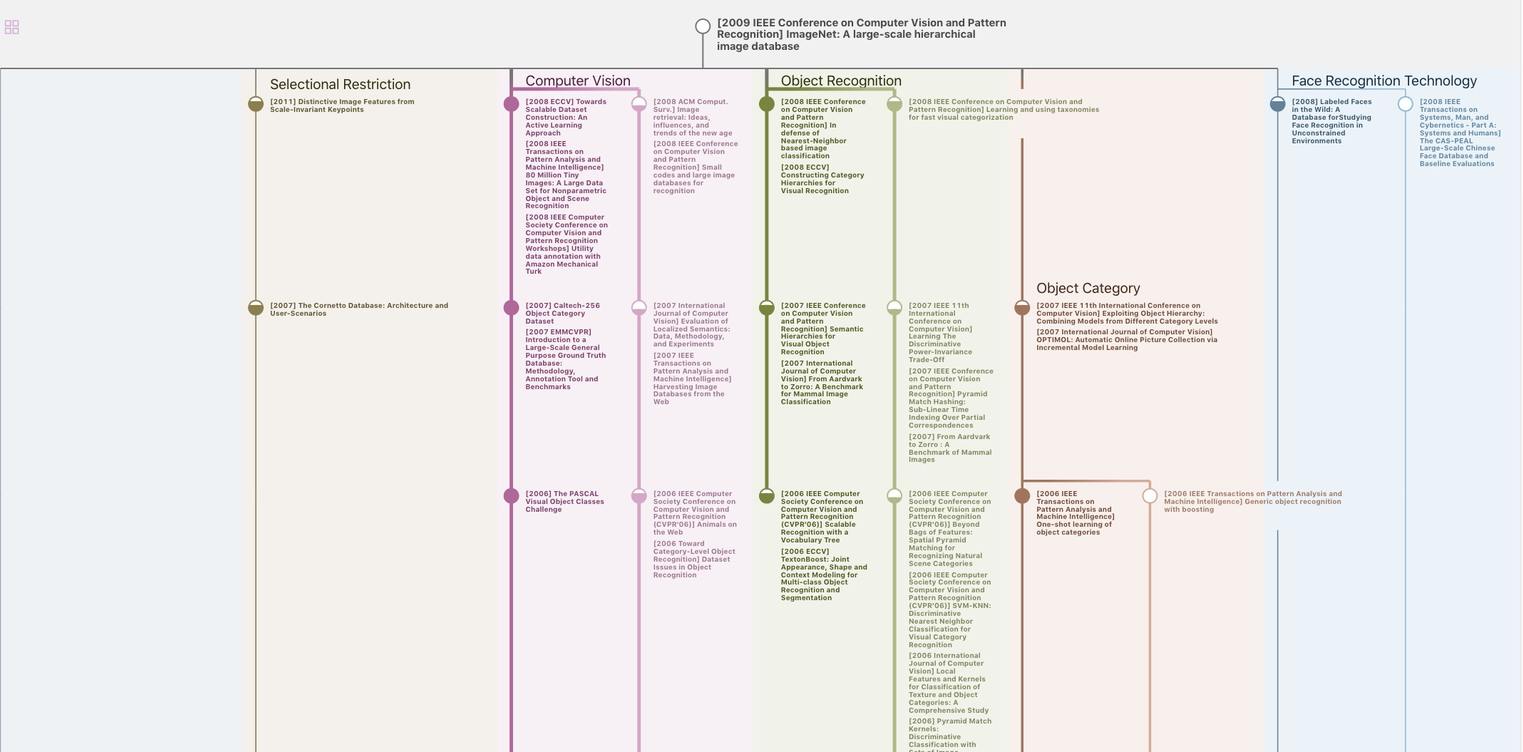
Generate MRT to find the research sequence of this paper
Chat Paper
Summary is being generated by the instructions you defined