Scalable Multi-Framework Multi-Tenant Lifecycle Management of Deep Learning Training Jobs
semanticscholar(2017)
摘要
With the ongoing rise and phenomenal success of machine learning (ML), particularly deep learning, efficient training of large neural network models in scalable cloud infrastructures becomes a priority. ML workloads have traditionally been run in high-performance computing (HPC) environments, where users log in to dedicated machines and utilize the attached GPUs to run jobs that train models on huge datasets. Providing a similar user experience in a multi-tenant cloud environment comes with its own unique challenges regarding fault tolerance, performance, and security. We tackle these challenges and present a deep learning stack specifically designed for on-demand cloud environments. Based on a detailed discussion of the system architecture, we examine real usage data from internal users, and discuss performance experiments that illustrate the scalability of the system.
更多查看译文
AI 理解论文
溯源树
样例
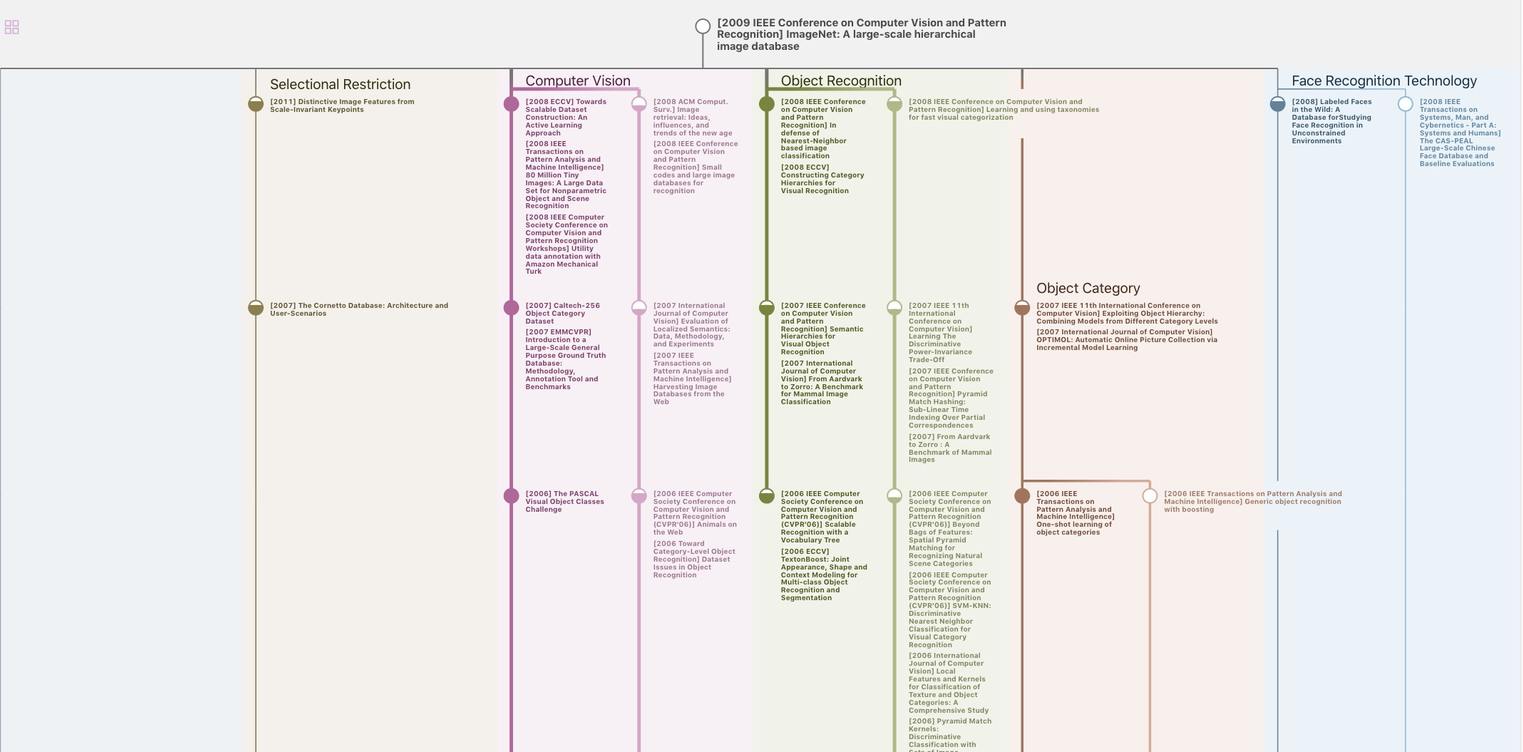
生成溯源树,研究论文发展脉络
Chat Paper
正在生成论文摘要