Lower PAC bound on Upper Confidence Bound-based Q-learning with examples
semanticscholar(2016)
摘要
Abstract Recently, there has been significant progress in understanding reinforcement learning in Markov decision processes (MDP). We focus on improving Q-learning and analyze its sample complexity. We investigate the performance of tabular Q-learning, Approximate Q-learning and UCB-based Q-learning. We also derive a lower PAC bound Ω( |S| |A| 2 ln |A| δ ) of UCB-based Q-learning. Two tasks, CartPole and Pac-Man, are each solved using these three methods. Some results and discussion are presented at last. UCB-based learning does better in exploration but lose its advantage in exploitation, compared to its alternatives.Recently, there has been significant progress in understanding reinforcement learning in Markov decision processes (MDP). We focus on improving Q-learning and analyze its sample complexity. We investigate the performance of tabular Q-learning, Approximate Q-learning and UCB-based Q-learning. We also derive a lower PAC bound Ω( |S| |A| 2 ln |A| δ ) of UCB-based Q-learning. Two tasks, CartPole and Pac-Man, are each solved using these three methods. Some results and discussion are presented at last. UCB-based learning does better in exploration but lose its advantage in exploitation, compared to its alternatives.
更多查看译文
AI 理解论文
溯源树
样例
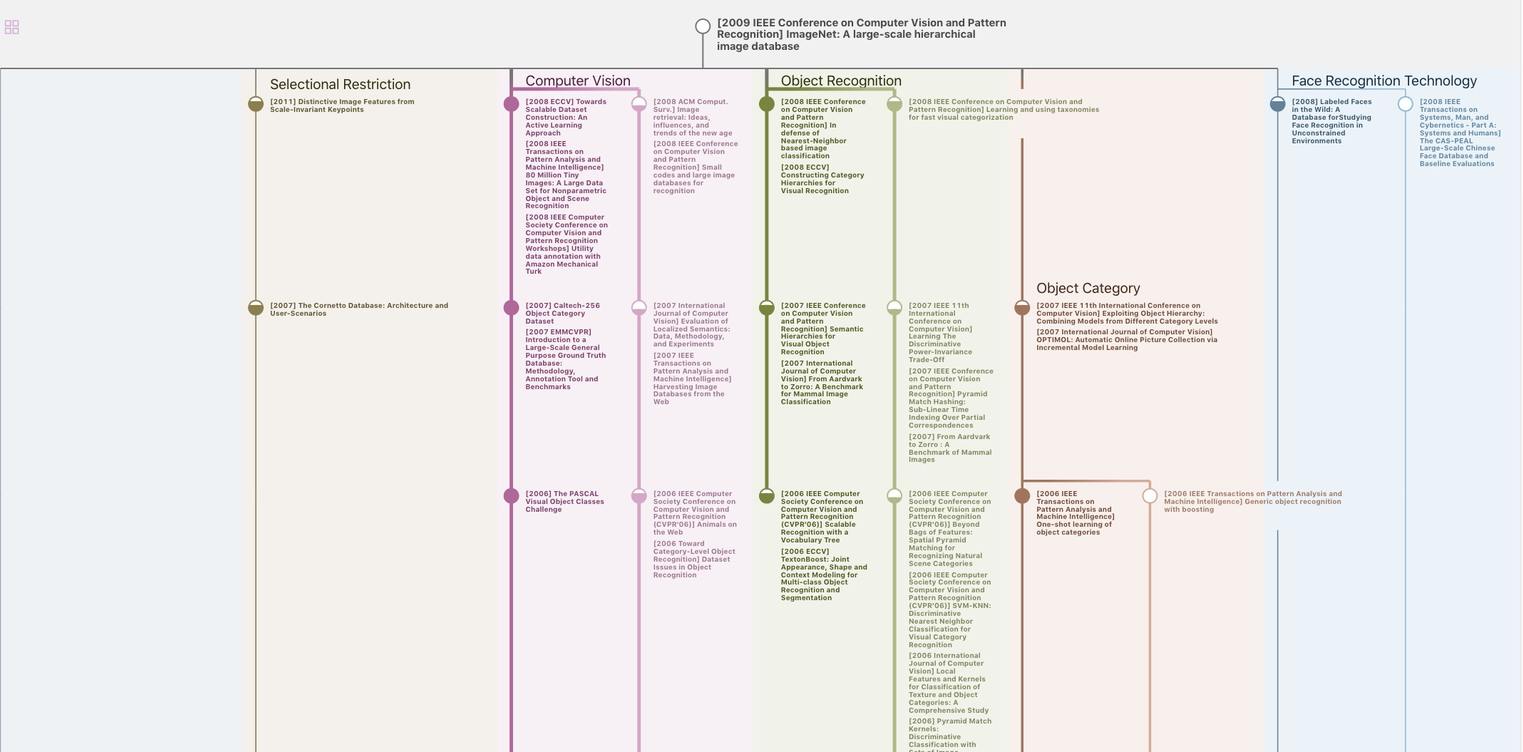
生成溯源树,研究论文发展脉络
Chat Paper
正在生成论文摘要