A cluster-centric batch-based question answering approach for fine-grained object recognition
semanticscholar(2020)
Abstract
Object recognition deals with the recognition of objects belonging to various categories such as aeroplane, bird, chair etc. A more challenging sub-problem is fine-grained object recognition wherein objects belonging to the subordinate categories in a same entry level category are to be recognized; such as recognition of bird species and car models. It is one of the cornerstones in object recognition due to its potential in augmenting human vision. In the recent years, fine-grained object recognition using Image based Recognition (IR) has seen tremendous progress with the advent of convolutional neural networks. However, IR is cost-sensitive as it is excessively picture-dependent and fails without the adequate number of quality pictures. On the other hand, a picture-independent recognition approach called Question-answering based recognition (QR) has been investigated in which the visual semantic attributes of objects are exploited by posing them as questions to a human-in-the-loop. The responses received to the questions are then utilized for recognizing the test object. However, QR entails heavy human labor as it relies entirely on the human input for recognition. Subsequently, hybrid human-computer recognition called Image and Question answering based recognition (IQR) has been proposed which utilizes both IR and QR jointly for more effective recognition. Still, many of the works in IQR are picture-dependent making them cost-sensitive. The few picture-independent works under IQR which use binary/multiple choice question answering are slow and fail to produce the desirable performance as needed by the present recognition applications. To this end, in this thesis, we propose an improved batch-based question answering method for making the IQR recognition cost-effective, i.e., efficient and picture-independent. We refer to the proposed approach as Recognition via Image and Batch based local Question answering (RIBQ). Unlike the existing picture-independent recognition methods, the proposed method poses a batch of multiple discriminating questions at each time-step to the human-in-the-loop for distinguishing the probable classes. As a part of this, we introduce a cluster based local feature extraction method for finding the best set of N highly discriminating questions to pose to the human-in-the-loop at each time-step. At each time-step, the proposed method first clusters the probable classes into N clusters. From each of the obtained clusters, we mine an attribute whose expected presence/absence is exclusively predominant in that cluster using the proposed concept of locality degree of an attribute. The mined set of attributes from the clusters called as cluster-centric local attributes are then posed as questions to the
MoreTranslated text
AI Read Science
Must-Reading Tree
Example
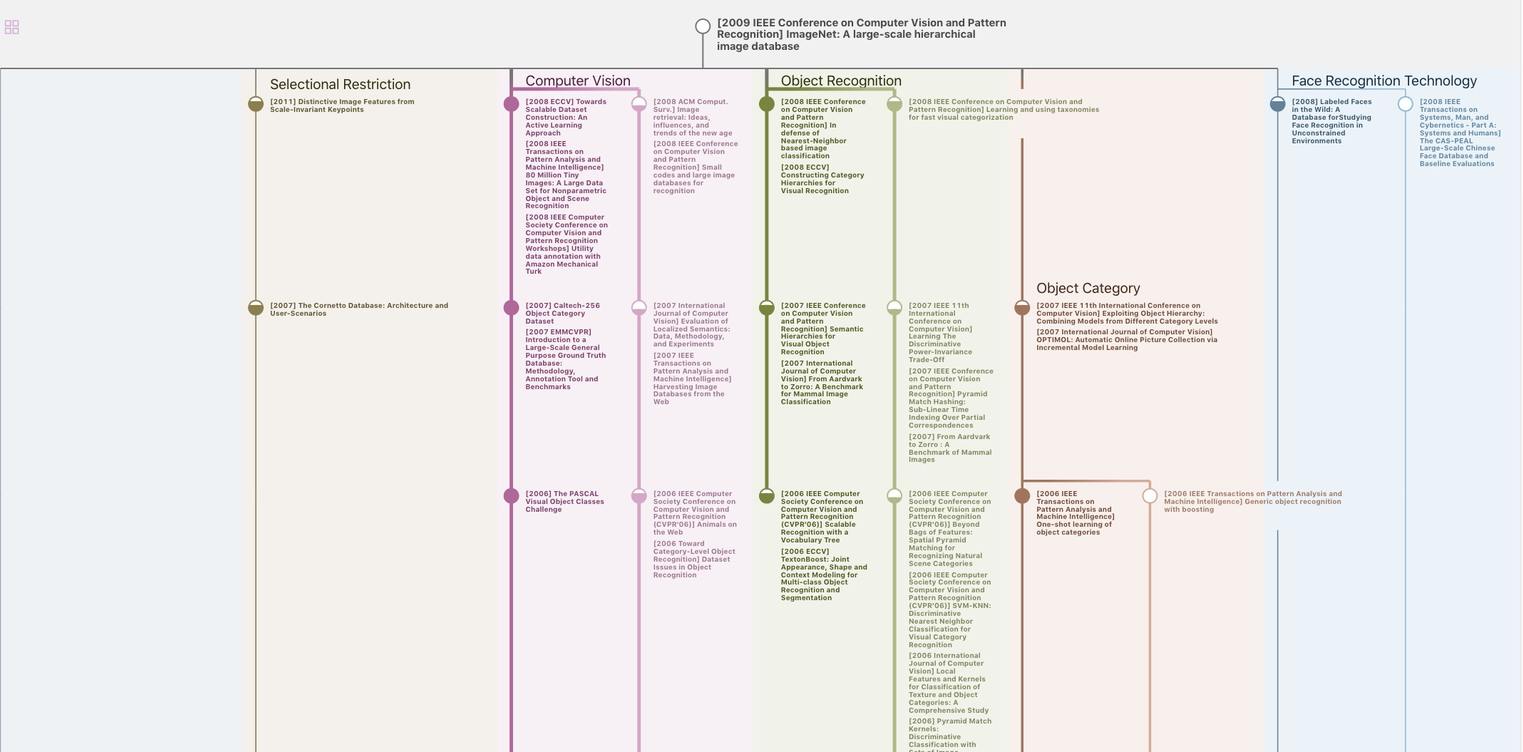
Generate MRT to find the research sequence of this paper
Chat Paper
Summary is being generated by the instructions you defined