Prediction of Cholera Epidemics in Africa
semanticscholar(2010)
摘要
OBJECTIVE This paper describes two methodologies that we developed for predicting cholera epidemics in Africa. BACKGROUND Long lasting cholera outbreaks in Africa suggest limitations in the current strategy of disease control [1]. Effective methodologies to predict cholera outbreaks one to several months in advance would allow taking the preventive actions early, to avert large epidemics. Previous studies identified factors associated with cholera outbreaks, but most focused only on a few predictors in a single country. METHODS As part of development of an operational prediction system, we assessed demographic, economic, environmental, and climatic variables associated with Vibrio cholerae infections across Africa during 19952005. The epidemiological data came from ProMedmail as presented in [2]. The main cited causes of outbreaks include water source contamination, poor sanitation, rainfall, flooding, and refugee settings. The predictor data sets that we identified as showing correlation with outbreaks included data from World Health Organization (percent improved drinking water, percent improved sanitation, Gross National Income per capita, life expectancy at birth), National Aeronautics and Space Administration (rainfall), National Oceanic and Atmospheric Administration (southern oscillation index, sea surface temperature), Gridded Population of the World (population density, percent urban constitution), Dartmouth Flood Observatory (flood, flood severity, flood susceptibility). Another predictor that we used was the occurrence of a cholera outbreak in a neighboring province during the previous month. We used two approaches for predicting cholera outbreaks: hierarchical logistic regression (LR) and Support Vector Machines (SVM). Both methods performed predictions one month in advance, separately for each province of each country from Western, Eastern, Middle and Southern Africa. The models excluded Northern Africa since there were almost no outbreaks in that region in our data set. RESULTS The LR models were developed using 1997-2004 data, and tested on 2005 data. They used the following predictors: population density, percent urban constitution, flood susceptibility, gross national income, occurrence of an outbreak in a neighboring province during previous month, region-specific random effects for rainfall one month prior, and countryspecific random intercepts. The SVM model was developed using 1996-2004 data and tested on 2005 data. There was only one model covering Western, Eastern, Middle and Southern Africa. SVM used all the fourteen predictors identified. The ROC curves for the final logistic regression and SVM models (Fig. 1) show similar predictive accuracy, with a slight advantage by logistic regression. Logistic Regression has certain separate parameters for Eastern, Western, Middle and Southern Africa. The SVM has at this point only one model covering the whole four regions of the continent.
更多查看译文
AI 理解论文
溯源树
样例
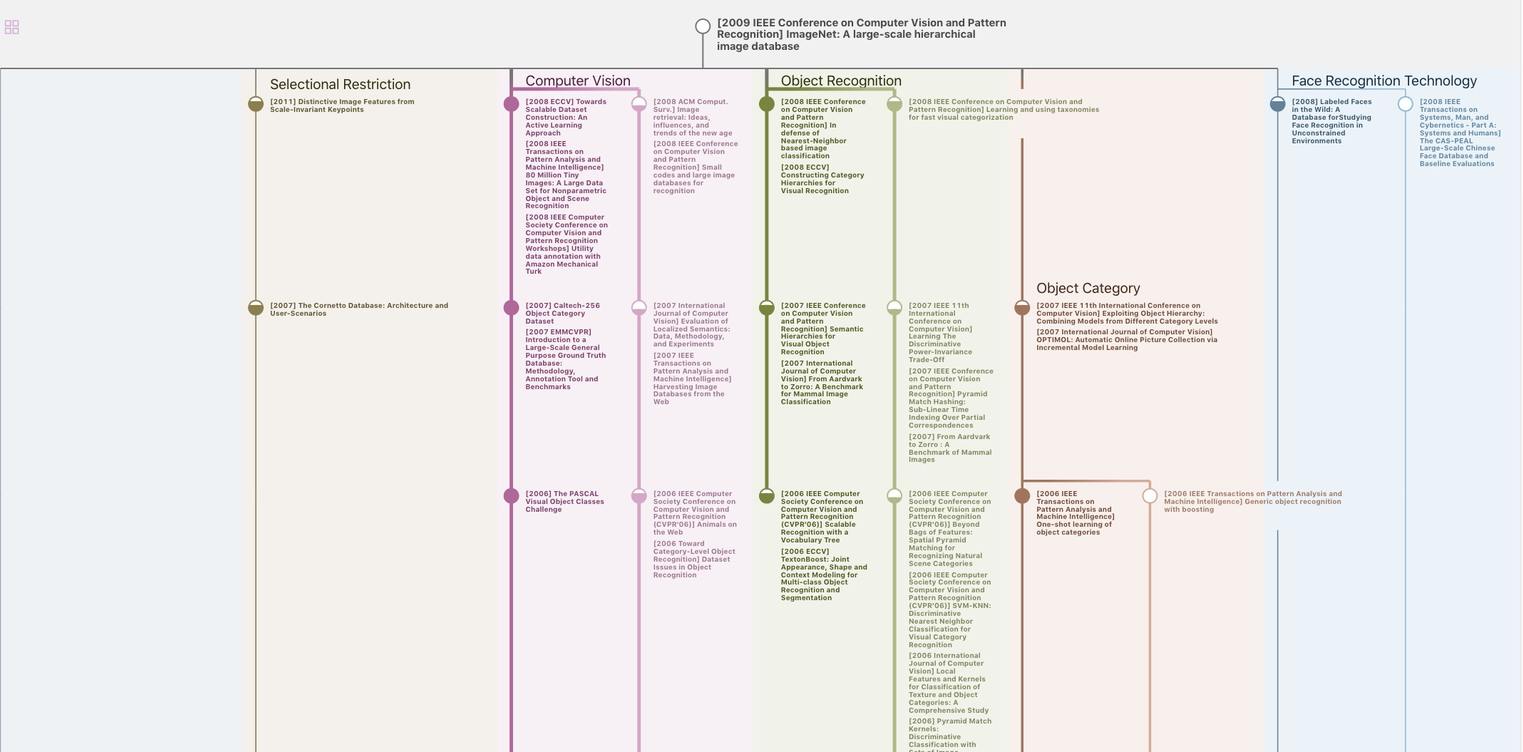
生成溯源树,研究论文发展脉络
Chat Paper
正在生成论文摘要