Hierarchical PAC-Bayes Bounds via Deep Probabilistic Programming
semanticscholar(2019)
摘要
PAC-Bayes approaches have recently generated some of the tightest generalization bounds for neural networks, as well as providing objective functions for regularization when training networks de novo, and in the context of transfer learning. However, existing approaches often place restrictions on the form of the prior and/or posterior. We show how general and tractable PAC-Bayes bounds can be derived in a deep probabilistic programming (DPP) framework. This allows both prior and posterior to be arbitrary DPPs, hyper-priors to be easily introduced, and variational techniques to be used during optimization. We test our framework using generalization and transfer learning tasks on synthetic and biological data.
更多查看译文
AI 理解论文
溯源树
样例
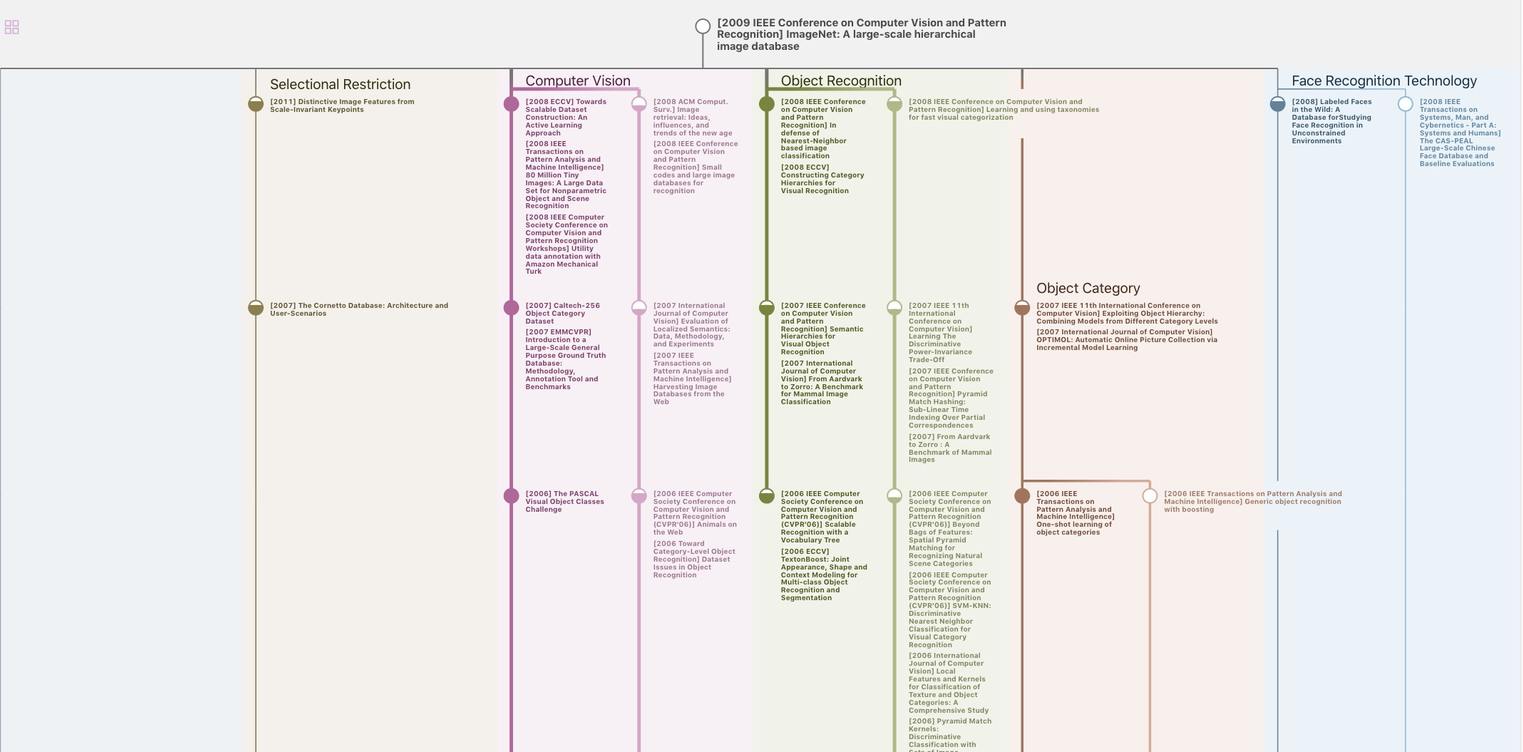
生成溯源树,研究论文发展脉络
Chat Paper
正在生成论文摘要