A parallelized model reduction library
semanticscholar(2013)
摘要
We describe a new parallelized Python library for model reduction, modal analysis, and system identification of large systems and datasets. Our library, called modred, handles a wide range of problems and any data format. The modred library contains implementations of Proper Orthogonal Decomposition (POD), balanced POD (BPOD), Petrov-Galerkin projection, and a more efficient variant of the Dynamic Mode Decomposition (DMD). The library contains two implementations of these algorithms, each with its own advantages. One is for smaller and simpler datasets, requires minimal knowledge to use, and follows a common matrix-based formulation. The second, for larger and more complicated datasets, preserves the abstraction of vectors as elements of a vector space and, as a result, allows the library to work with arbitrary data formats and eases distributed memory parallelization. We also include implementations of the Eigensystem Realization Algorithm (ERA), and Observer/Kalman Filter Identification (OKID). These methods are typically not computationally demanding and are not parallelized. The library is designed to be easy to use, with an object-oriented design, and includes comprehensive automated tests. In almost all cases, parallelization is done internally so that scripts that use the parallelized classes can be run in serial or in parallel without any modifications.
更多查看译文
AI 理解论文
溯源树
样例
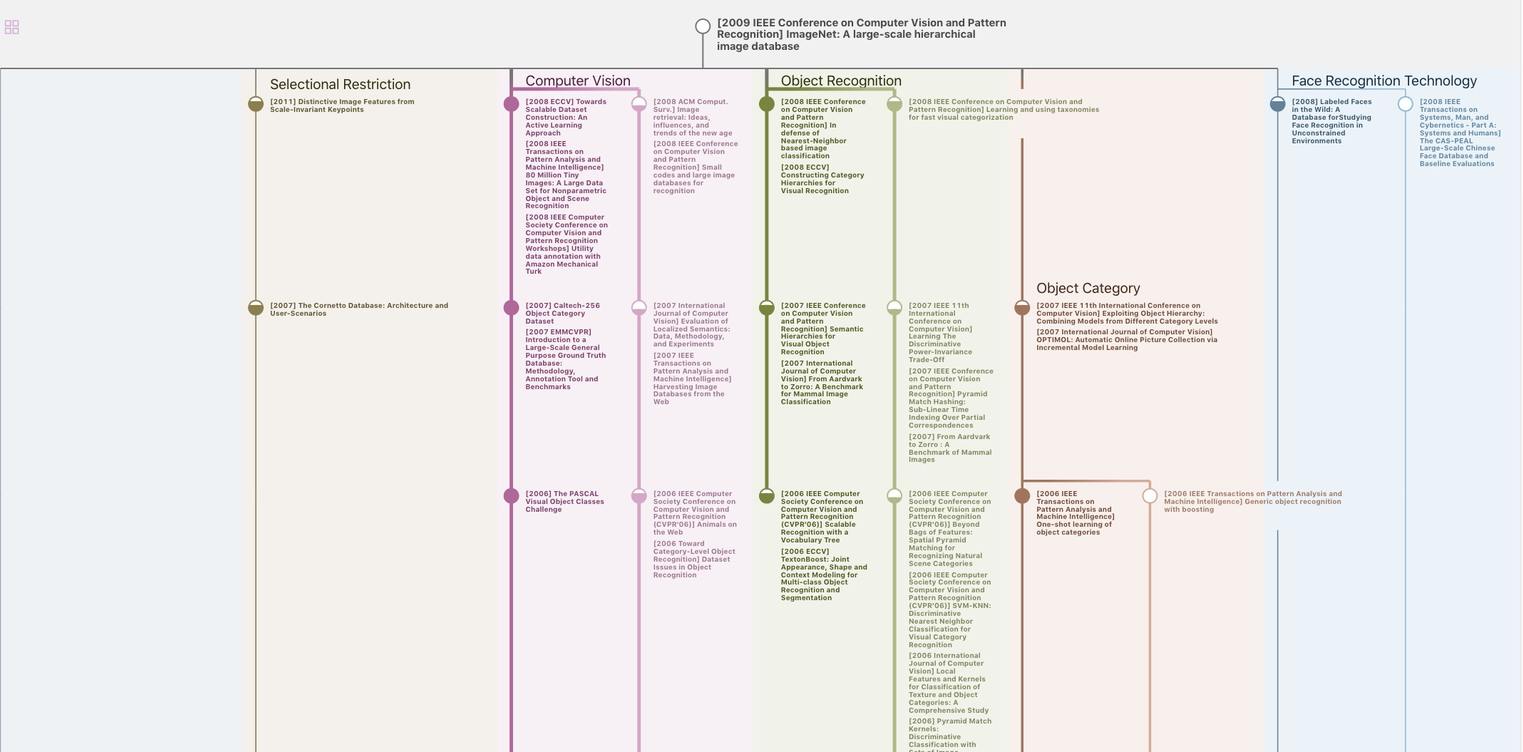
生成溯源树,研究论文发展脉络
Chat Paper
正在生成论文摘要