Nonlinear Modeling via Self-Organizing Trees
semanticscholar(2017)
摘要
We study online supervised learning and introduce regression and classification algorithms based on self-organizing trees (SOTs), which adaptively partition the feature space into small regions and combine simple local learners defined in these regions. The proposed algorithms sequentially minimize the cumulative loss by learning both the partitioning the feature space and the parameters of the local learners defined in each region. The output of the algorithm at each time instance is constructed by combining the outputs of a doubly exponential number (in the depth of the SOT) of different predictors defined on this tree with reduced computational and storage complexity. The introduced methods are generic such that they can incorporate different tree construction methods than the ones presented in this chapter. We present a comprehensive experimental study under stationary and nonstationary environments using benchmark datasets and illustrate remarkable performance improvements with respect to the state-of-the-art methods in the literature.
更多查看译文
AI 理解论文
溯源树
样例
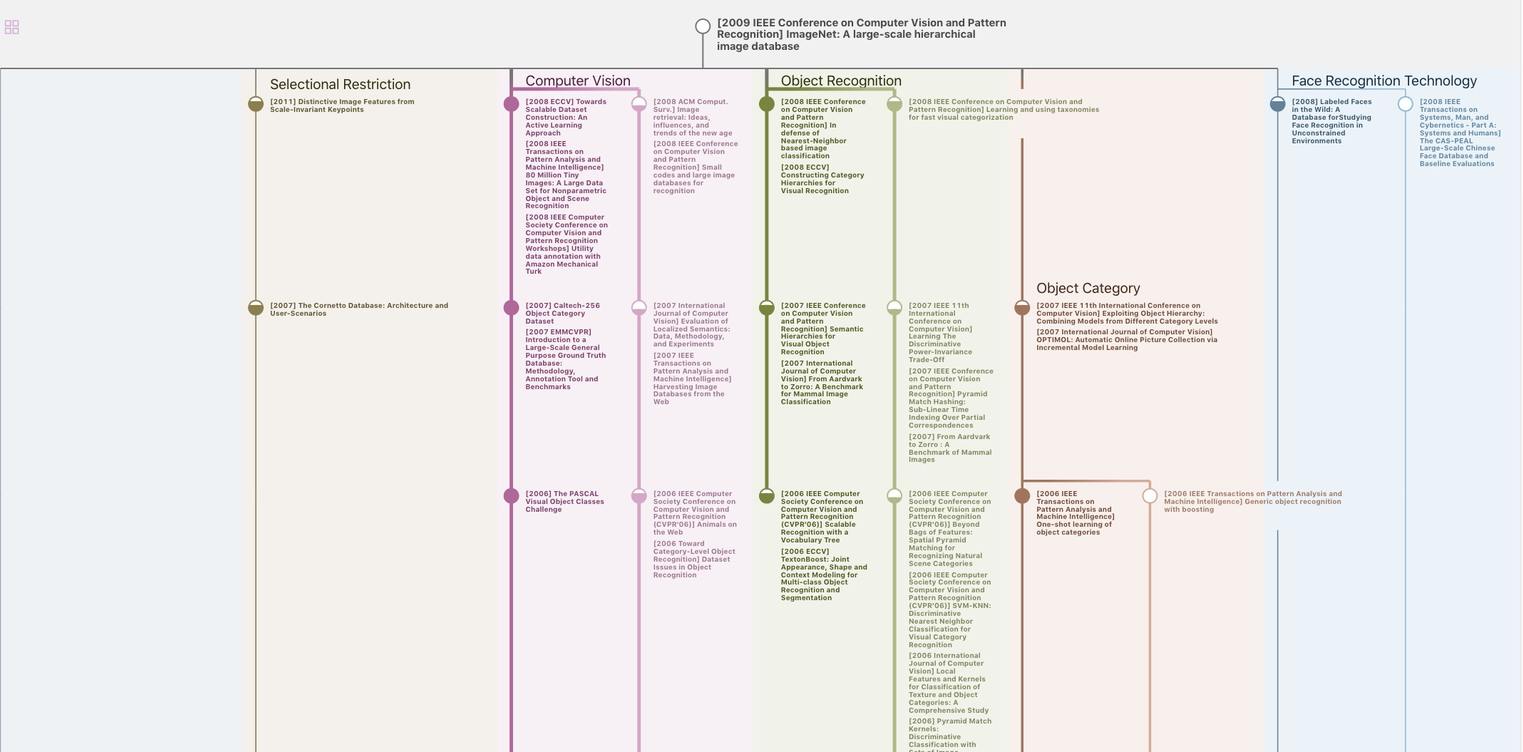
生成溯源树,研究论文发展脉络
Chat Paper
正在生成论文摘要