Optimal Separation , Detection , and Analysis of FISH Images
semanticscholar(2018)
摘要
Fluorescence In-Situ Hybridization (FISH) is a widely-used technology in biological research. FISH images show the locations of specific nucleotide-based species in the cell, such as cellular RNA transcripts, genomic regions of interest such as specific genes or non-coding regions, and chromosomal features such as telomeres among others. Though manual counting of spots remains the most common methods of processing FISH images by biological researchers, the advent of high throughput labeling technologies makes this extremely difficult, as high throughput single molecule FISH images may have on the order of thousands of individual spots per cell. Strategies exist to automatically analyze FISH data, but few are broadly applicable. We implemented and analyzed the performance of 4 algorithms for FISH spot counting, as well as 3 ensemble methods and an additional post-processing segmentation strategy to refine FISH spot detection. We found that K-means based segmentation performed best when measured by Precision Recall plots. We found that adding a further spot detection segmentation step after the original algorithm improved performance, but further optimization is required. Finally, we qualitatively assessed our algorithms on a new mouse placental cell dataset and found biologically meaningful results.
更多查看译文
AI 理解论文
溯源树
样例
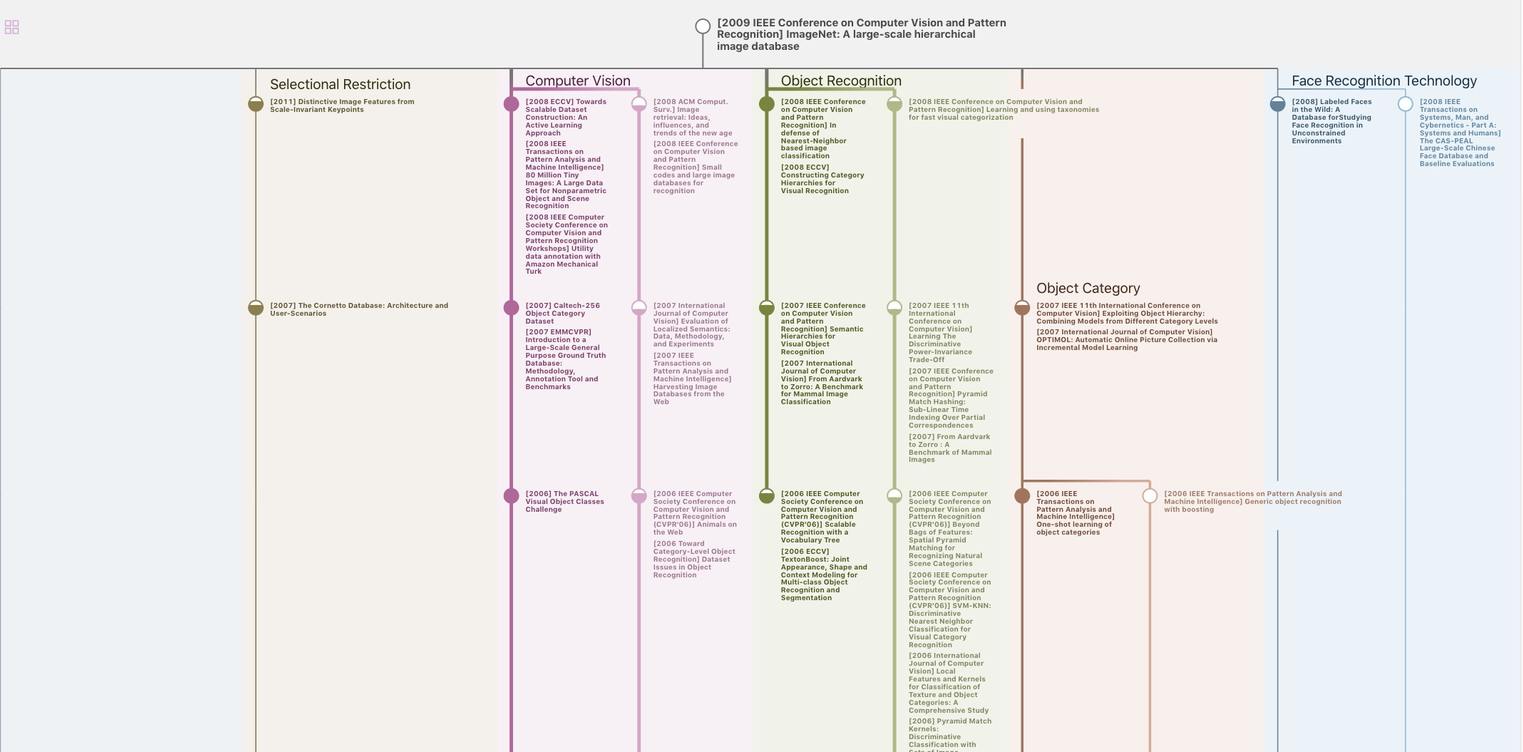
生成溯源树,研究论文发展脉络
Chat Paper
正在生成论文摘要